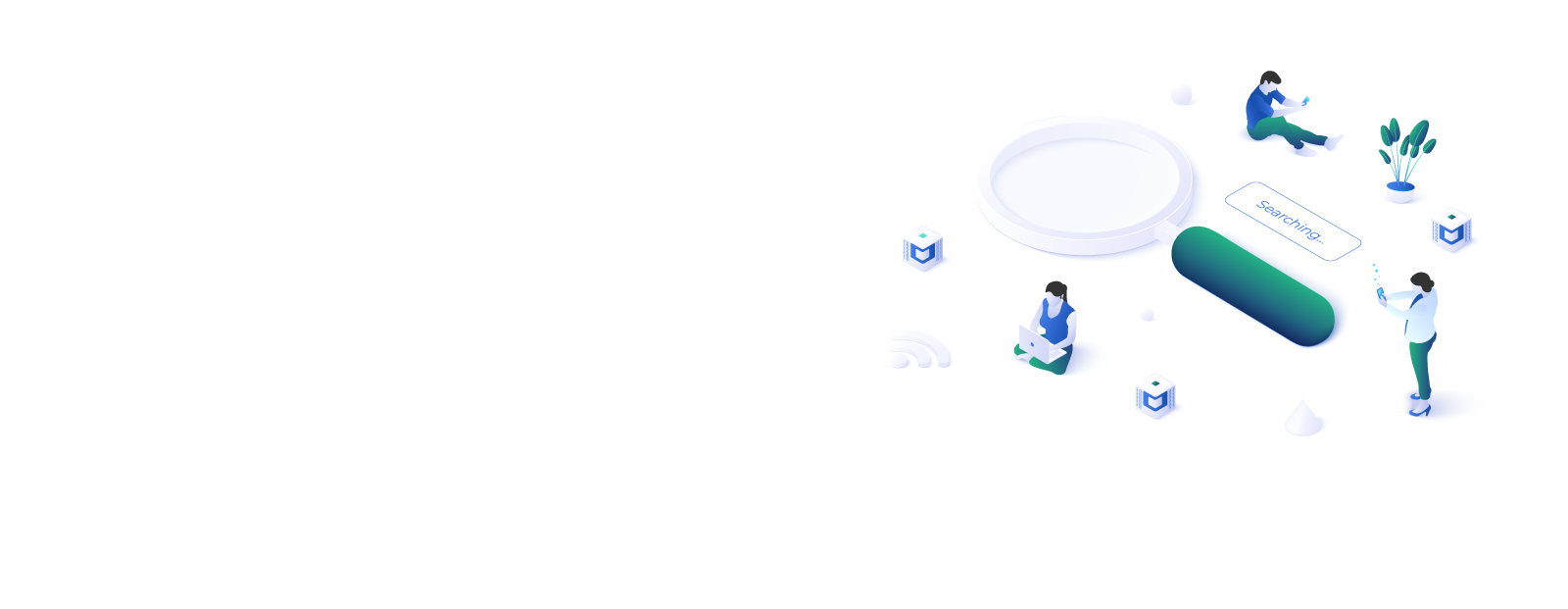
Parameta Solutions
Parameta Solutions – Web Search Engine and Entity Extraction
Information Technology & ServicesBusiness Impacts
32
different keywords searched in prospectus documents across the web
>90%
accuracy of classification model for identifying the downloaded documents
12
different entities/fields fetched from the documents
Customer Key Facts
- Location : United Kingdom
- Industry : Information Technology
Problem Context
Parameta Solutions uses search engines to assist with its regulatory compliance. Due to the lack of an automated search framework, the client had to manually search and browse through the web to locate the prospectus documents, and further analyze the document content from a regulatory perspective.
They were looking for a Search and Extract solution to search, extract, and analyze public prospectus documents on the web using predefined keywords, automating the existing manual process.
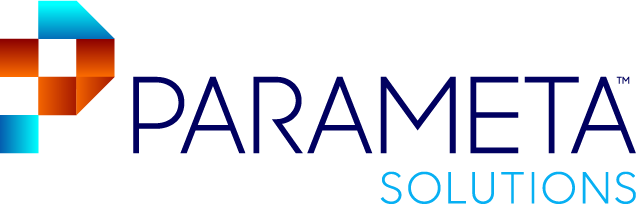
Challenges
- Laborious process of searching the relevant documents on the internet
- Manual classification of prospectus/non-prospectus documents
- Limitation in identifying the key entities/fields for regulatory compliance
- Access to the latest data on the internet
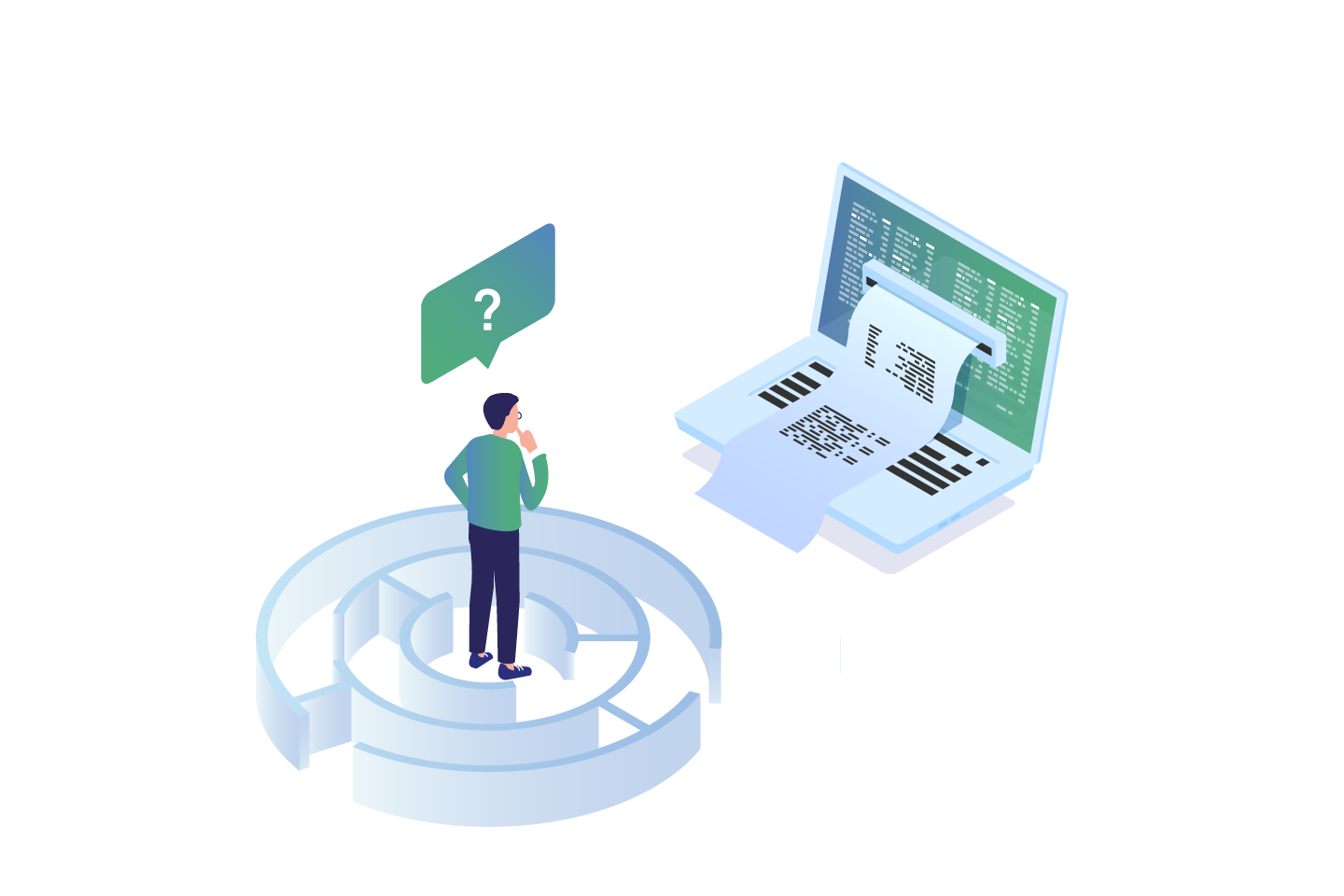
Technologies Used
Google Cloud

Google Cloud Identity Access Management
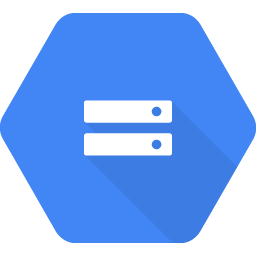
Google Cloud Storage

Google Cloud Scheduler
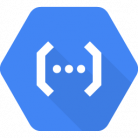
Google Cloud Functions
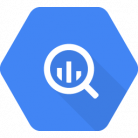
Google BigQuery
Google Cloud Auto ML
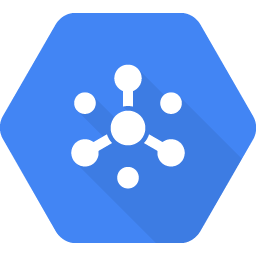
Google Cloud Pub/Sub
Solution
- Quantiphi built an easy-to-use customized Web Search and Entity Extraction solution for Parameta Solutions.
- Powered by Google’s Programmable Search Engine, the solution helps to search and locate the prospectus documents from the internet using 32 predefined keywords.
- The identified documents are downloaded and stored in Google Cloud Storage buckets. A classification model categorizes these documents into two types: Prospectus and Non-prospectus.
- These documents are then passed through an end-to-end automated entity extraction pipeline which helps extract the required entities from the documents using AutoML models.
- The extracted entities are stored in BigQuery for downstream analytics, and can be easily exported as .CSV files.
- The entire solution is supported by a robust GCP infrastructure.