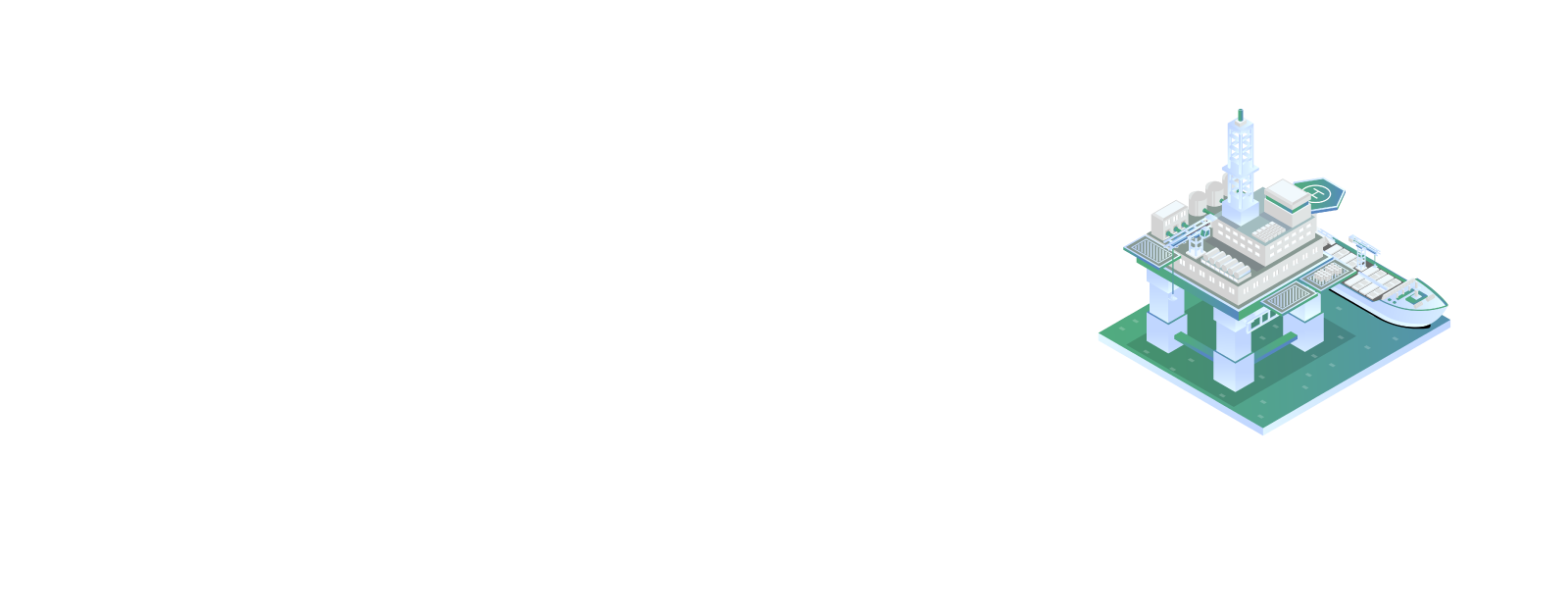
Scalable 3D Image Segmentation
Energy & UtilitiesBusiness Impacts
Efficient Visualization of Overlap Results
Improvement in Process Efficiency
Improved UX with Modular Model Selection
Customer Key Facts
- Location : Europe
- Industry : Oil & Energy
Problem Context
The client, a French multinational integrated oil and gas company and one of the seven “Supermajor” oil companies in the world, wanted to augment the capabilities of their current software by providing refactoring and additional features that highlight seismic artifacts. The inherent data (geological) characteristics required a deeper customization of the model components.
Challenges
- Hardware memory limitations
- Latency requirements
- Technical domain specific processing
- Custom loss functions
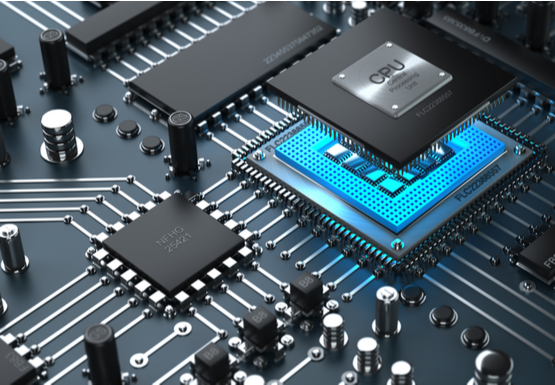
Technologies Used

Cloud Functions
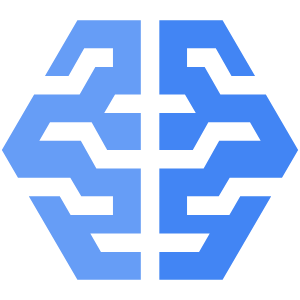
Cloud ML Engine

Cloud Dataflow
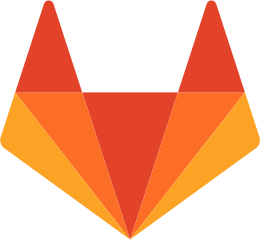
GitLab
Building Scalable 3D Image Segmentation Models with ML Pipelines for Training & Prediction
Solution
Quantiphi built a robust multi-functionality application for geoscientists to train seismic machine learning models and predict 2D seismic images and 3D volumes; allowing flexibility when running the models on Cloud Machine Learning Engine (CLME).
Result
- Scalable and robust ETL pipeline
- Functional web app to overlap seismic volumes and artifacts
- Modularity for users to select the relevant models for inference directly from the UI