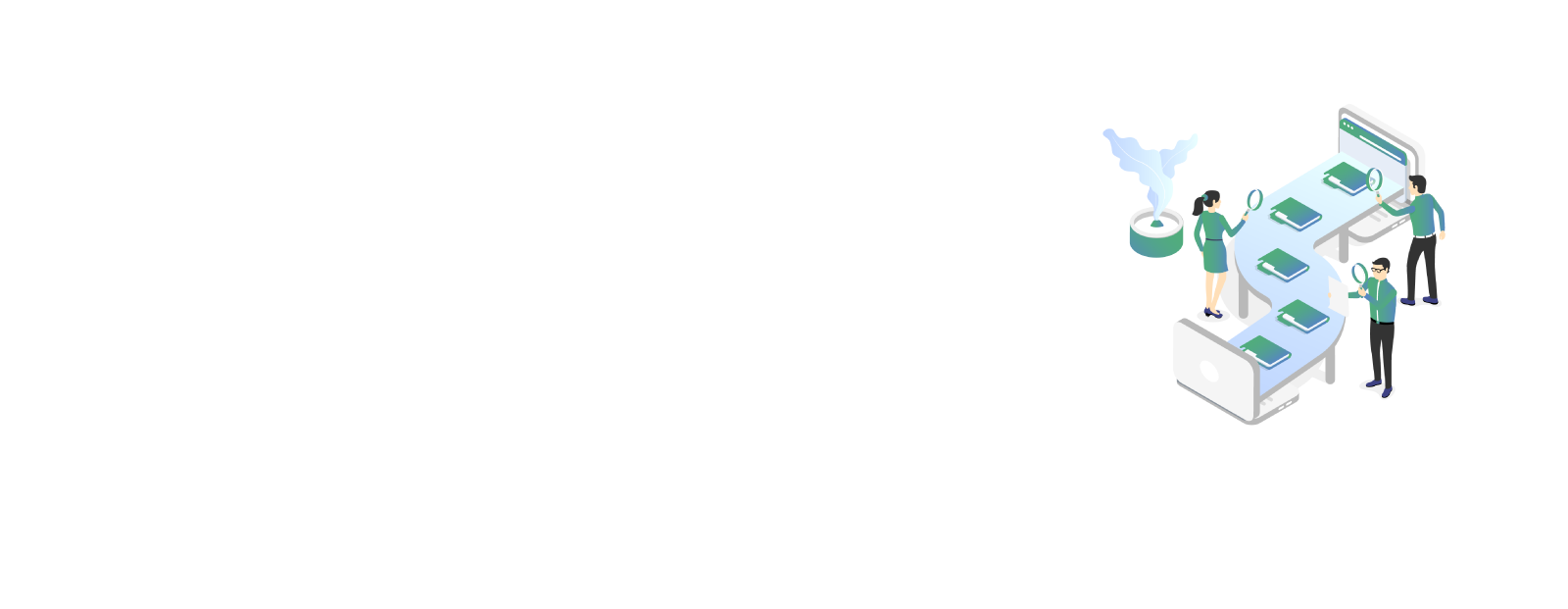
Automated Check Image Processing
Banking & Financial ServicesBusiness Impacts
95%
Model accuracy for printed text
75%
Model accuracy for handwritten text
2s
Processing time per check
Customer Key Facts
- Location : North America
- Industry : Financial Services
Problem Context
Financial Services institutions need to process thousands of checks on a daily basis. Manually going through the check images to extract information is a very time-consuming process. While the structure of endorsements is similar across financial institutions, the vast variation in templates and types of handwriting constitutes a true challenge.
Challenges
- Huge variation in the format and layout of checks
- No access to real data that resulted in mock data generation
- Manual effort needed for the check tagging and endorsement verification
- Dealing with a third-party software for the information extraction process resulting in overhead costs
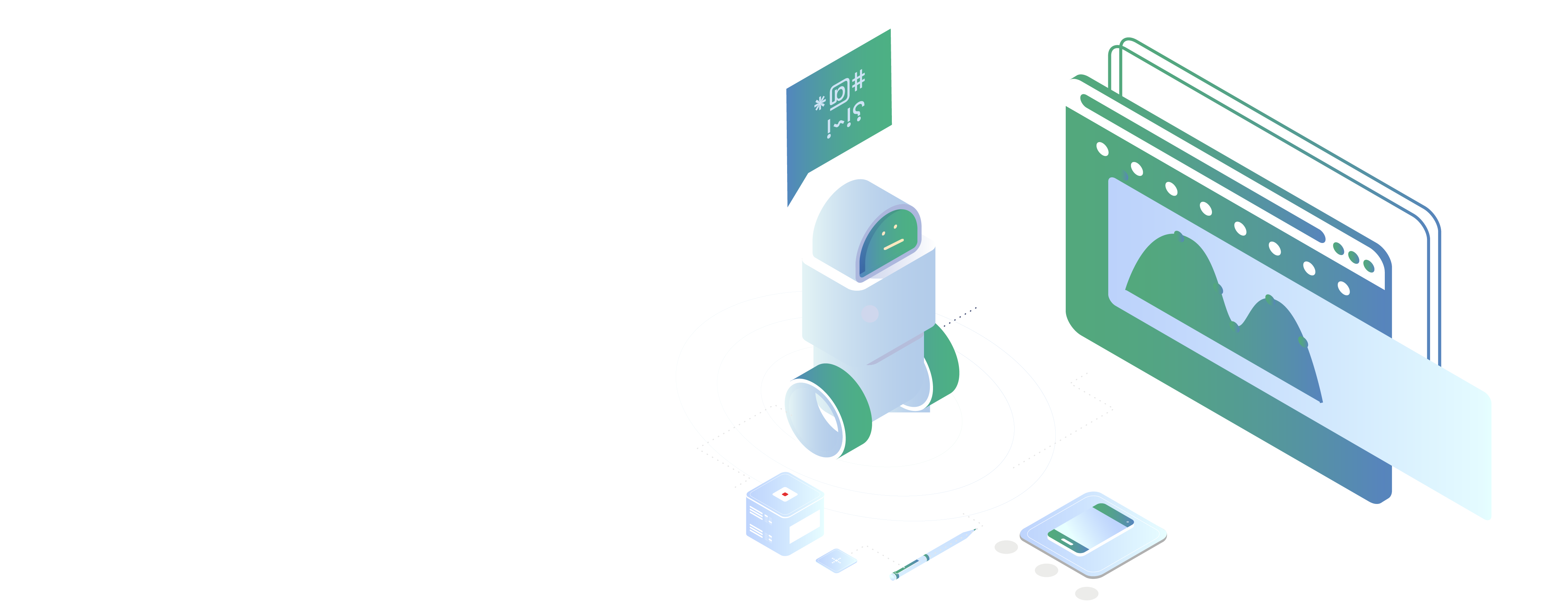
Technologies Used
Google Cloud Platform
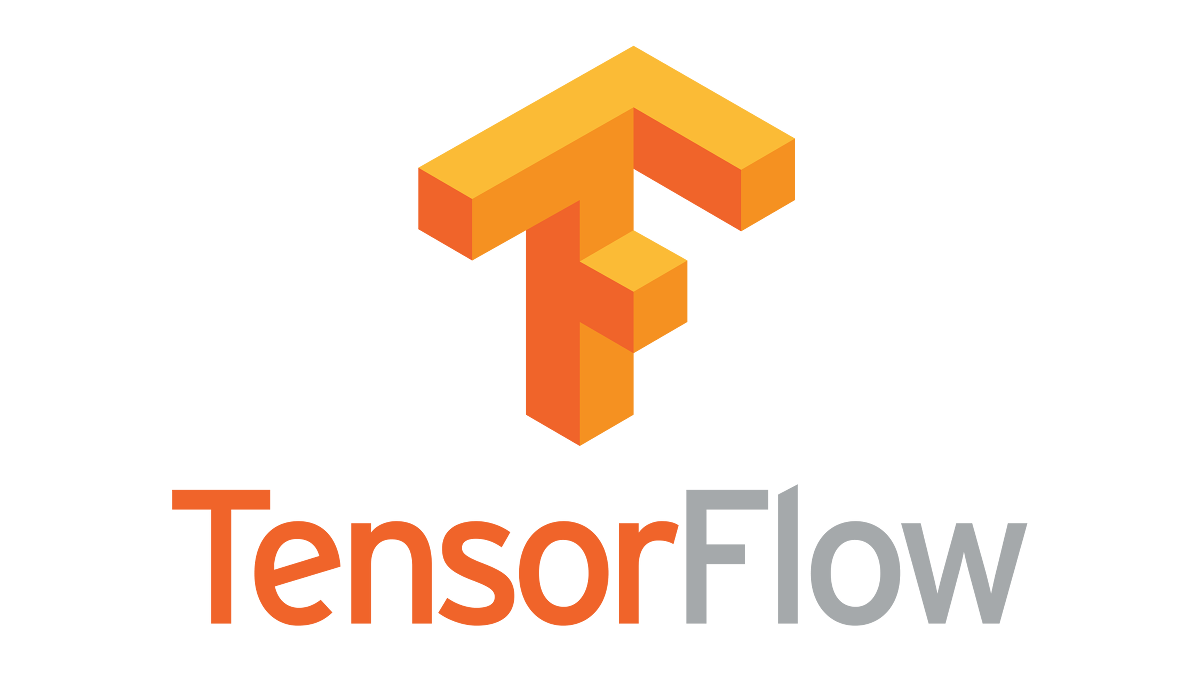
TensorFlow
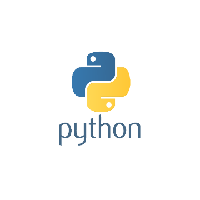
Python
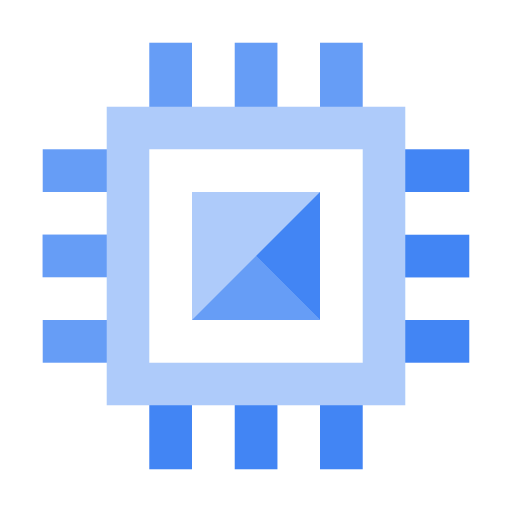
Compute Engine
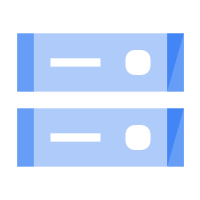
Cloud Storage
Automating Manual Bank Check Screening Process Using Deep Learning
Solution
Quantiphi developed a custom machine learning-based solution to automate the detection of fields of interest and extraction of the corresponding information. The solution processes and captures check details furnished by customers in less than two seconds with no manual intervention and with a detection accuracy of over 96 percent across all fields.
Minimal manual interruption enables a near error-free check endorsement process, with reduced misinterpretation of handwriting and input typos/incorrect spellings recorded. The solution is scalable and PII compliant; thus reducing costs and security issues at production-level.
Result
- Real-time solution: A single check image will process and display information in around three seconds
- Reduced manual effort