The ever-increasing demand for medical imaging across the globe is keeping radiologists working at full capacity. This imbalance is only expected to get more skewed as imaging volumes are projected to increase at a much faster rate than the number of new radiologists entering the field. Although radiology departments are typically well-resourced, radiologists are under growing pressure due to the additional efforts required to transition from volume-based service to value-based care delivery. In addition, manual interpretation of medical images is highly subjective, often contingent on an individual pathologist’s perception, experience, intuition, and knowledge.
According to Michael Snyder, Ph.D., Professor and Chair of Genetics at Stanford University, "Radiology as it is practiced now is very subjective. Two highly skilled radiologists assessing the same slide will agree only about 60% of the time. A machine learning approach to medical imaging replaces this subjectivity with sophisticated, quantitative measurements likely to improve patient outcomes."
Medical imaging encompasses a variety of technologies used to view the human body in order to diagnose, monitor, or treat medical conditions. Each technology provides unique information about the area of the body being studied or treated, related to disease, injury, or the effectiveness of medical treatment. Different types of medical imaging procedures include X-rays, magnetic resonance imaging (MRI), ultrasounds, endoscopy, tactile imaging, computerized tomography (CT), etc. The non-invasive nature of medical imaging tests allows radiologists to diagnose injuries and diseases without being intrusive.
Application of AI in Medical Imaging
The emergence of AI in medical imaging established artificial neural networks (ANN) as the backbone of machine learning and deep learning. The artificial neural network is an analysis algorithm made up of layers of connected nodes. A key driver for the prevalence of AI in medical imaging has been the enhancement of visual identification through AI in radiology, which generates lower error rates than that of a human observer.
The genesis of AI application in medical imaging dates back to the 1980s, prompting the advent of techniques such as principal component analysis (PCA), support vector machines (SVM), and convolutional neural networks (CNN). Since then, as the iterative evolution of machine learning algorithms has progressed in different imaging modalities and therapeutic areas, the adoption of AI in machine learning has resulted in key breakthroughs. It has revolutionized the landscape of the healthcare and life sciences industry, enabled professionals to diagnose maladies and diseases accurately, and fostered reliability by eliminating potential biases and inaccuracies that may occur due to human interpretation.
According to the Journal of the American College of Radiology (JACR), AI-driven computer-aided design software demonstrates fewer false positives in digital mammograms as compared to FDA-approved computer-aided design software, with no sensitivity losses. In recent years, there have been advancements in the application of AI in other fields too. Noteworthy among them is the role of AI in the treatment planning of brain tumors and the identification of catheters and tubes in pediatric X-ray images.
AI that leverages ML performs the following functions in medical imaging:
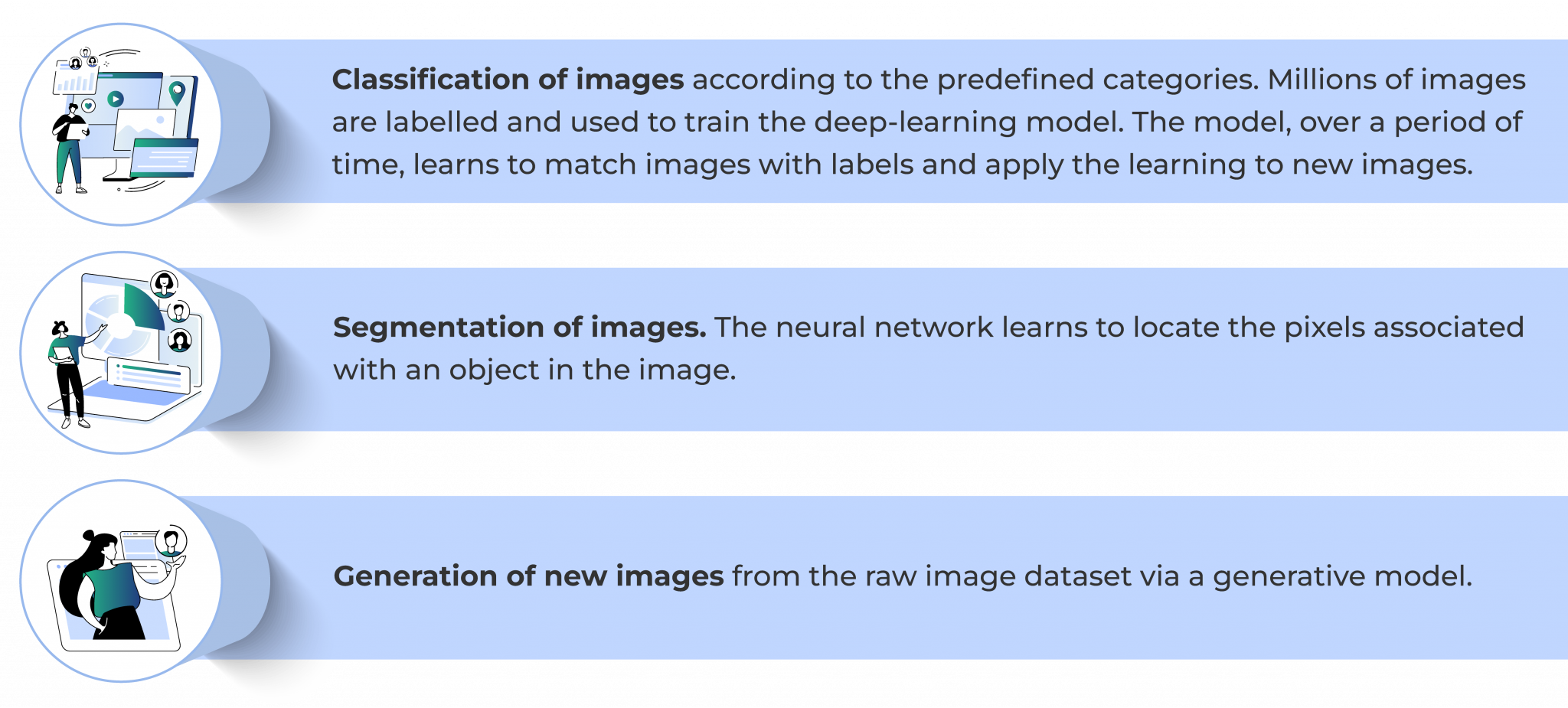
Apart from facilitating a deeper understanding of the underlying condition and its impact on the patient, a machine learning approach to medical imaging aids in tracking disease progression and in discerning responses to treatment, enhancing the scope for treatment personalization. This technique also makes early intervention possible due to its ability to identify specific characteristics and intricacies. Specific applications of machine learning in medical imaging include, but are not limited to, the detection and classification of lesions, automated image segmentation, image registration, data analysis, extraction of radiomic features, report prioritization and study triage, and image reconstruction.
Among these applications, image reconstruction is a primary step in the clinical workflow that significantly impacts further downstream tasks. Images acquired from medical imaging modalities such as CT, MRI, X-ray, and ultrasound are sometimes noted to suffer from low signal-to-noise ratio (SNR) and low contrast-to-noise ratio (CNR) along with image artifacts. To address and overcome these challenges, image reconstruction techniques are implemented in order to improve the quality of images for efficient visual interpretation, understanding, analysis, and decision making.
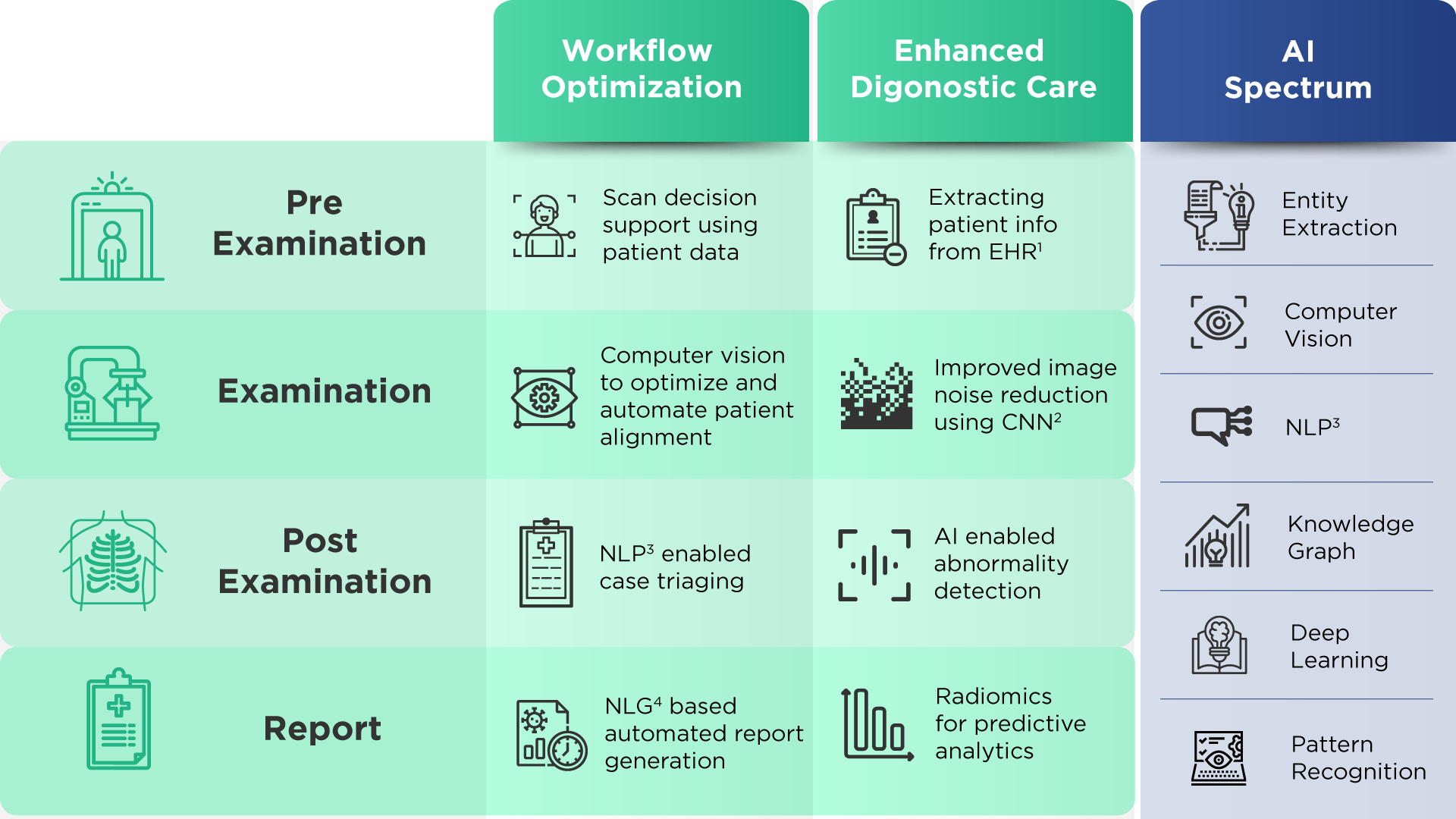
AI-led Medical Imaging in Practice
Quantiphi leverages the power of Google Cloud to develop and deploy futuristic solutions that cater to the needs of the healthcare industry. Google Cloud presents a range of services tailored to meet the security and privacy standards necessitated by the healthcare and life sciences industry. The Healthcare API is HIPAA-compliant and HITRUST CSF-certified and can wrangle patient de-identification tasks and PHI data. Quantiphi has successfully delivered over 110 projects with over 25 Fortune 500 clientele in the healthcare and life sciences segment alone. Quantiphi’s extensive experience, coupled with cutting-edge Google cloud offerings, facilitates the delivery of robust solutions of exceptional standards.
Success Story
Johns Hopkins University (JHU) is an American private research university and features among the most prestigious medical schools in the world. JHU houses the Brain Injury Outcomes (BIOS) Division. BIOS provides multicenter management for clinical trials evaluating therapeutic, preventive, and diagnostic interventions. BIOS is leading the CLEAR initiative, under which brain images are collected and collated from multiple institutions across the globe, and are routed to appropriate specialists, based on the findings of the scans.
The primary objective of Quantiphi’s engagement with JHU was to develop an ML model to aid doctors in identifying lesions, the volume of lesions, and their locations prior to treatment for Intracerebral Brain Hemorrhage (ICH) - a fatal stroke caused by bleeding within the brain tissues. The pre-existing process to measure and localize the lesion was tedious and led to high inference times. ICH blood clots were also measured manually, and therefore, were quite prone to errors. Since time is of the utmost essence in such cases, there was a pressing need to minimize decision-making time.
Quantiphi developed ML models to detect brain hemorrhage using CT scans and integrated all the manual pre-processing steps into the end-to-end pipeline. The overall cloud pipeline orchestration included data management and de-identification (integration with the Healthcare API and Data Loss Prevention API), model development, and a production-grade pipeline.
Key outcomes of the engagement include:
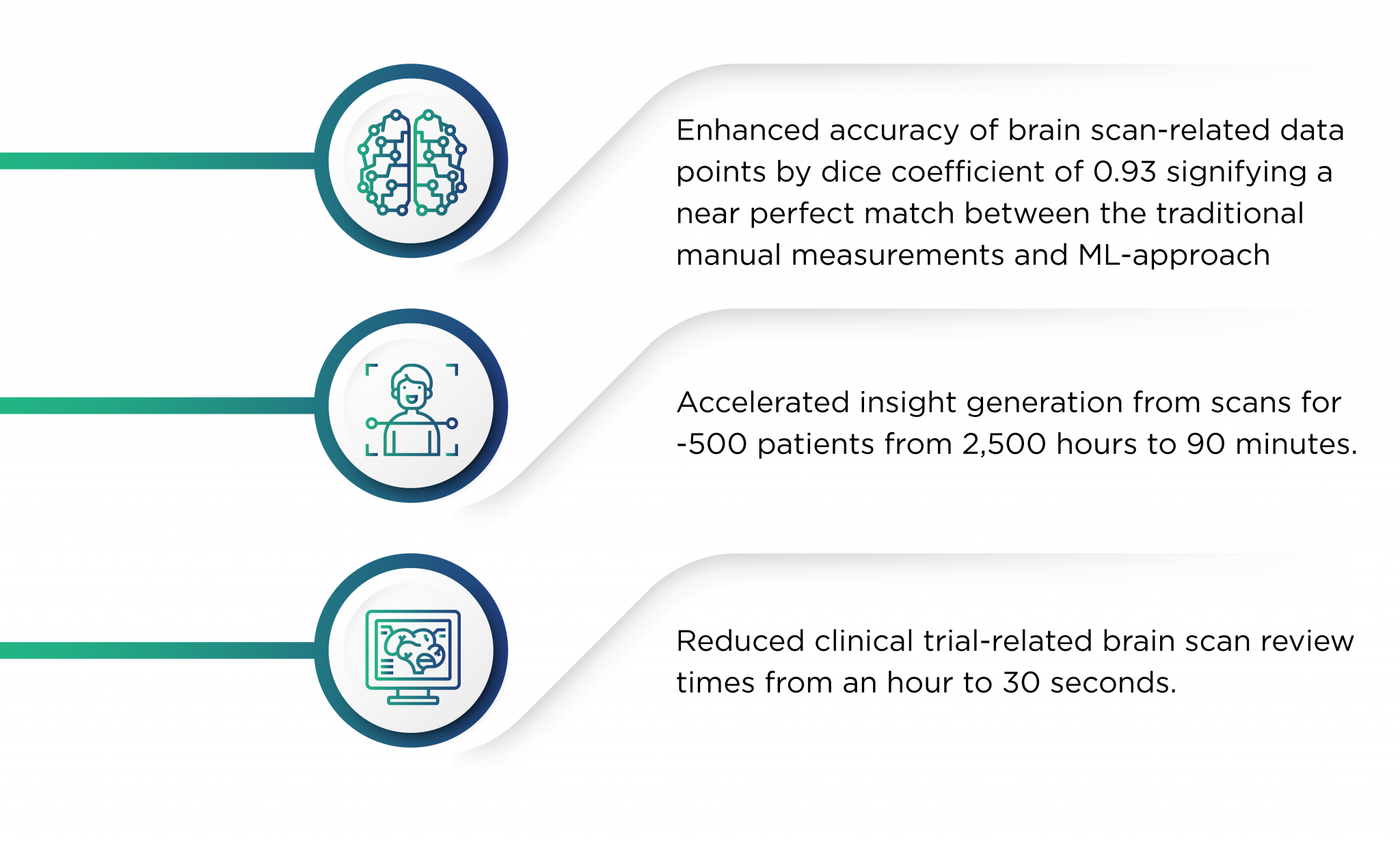
Get in touch with our experts to learn more about how radiologists can leverage AI-led medical imaging to enhance patient outcomes.