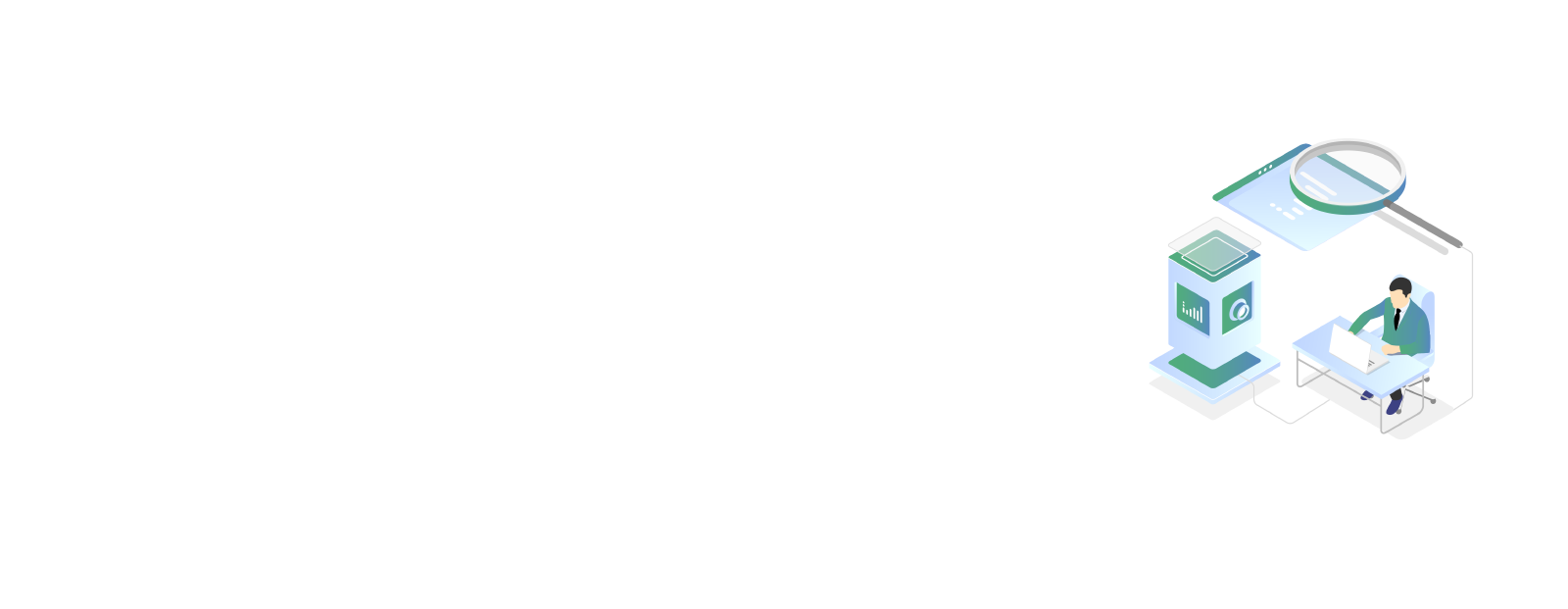
AI-Enabled Monitoring System for Railways
Public SectorBusiness Impacts
90%
Accuracy
10-15 FPS
Inference engine running
Customer Key Facts
- Location : Montreal, Canada
- Industry : Transportation
- Size : 24,000+ employees
Detect hazards and objects around railway tracks with intelligent video analytics
The customer, one of Canada’s largest railway organizations, wanted to undertake large-scale initiatives for situational awareness around train tracks to keep employees, consumers’ goods, and communities safe. The customer used railway personnel to patrol tracks, identify flags and signals, and respond to trespassers which was resource and labour intensive as well as geographically limited. The possibility of accidents as a result of poor human judgment posed a real concern for employees and civilians.
Challenges
- Delayed response time – Crew members must manually identify upcoming flags and signals
- Trespasser hotspots – Difficulty in detecting trespasser hotspots, leading to unwanted business losses (i.e. theft of cargo goods)
- Lack of Connectivity – No solution in place for connecting railway offices to edge devices and checking deployed system and hardware conditions
- Hardware Limitations – The edge device to be deployed had limited memory to run multiple classification and detection models
- Data Insufficiency – Poor lighting conditions and obstructed views resulted in poor prediction accuracy with customer’s in house model
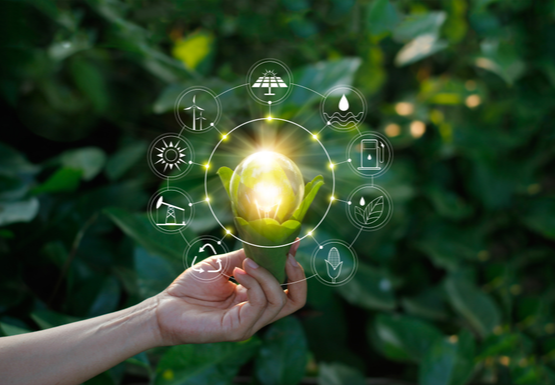
Technologies Used
Google AutoML
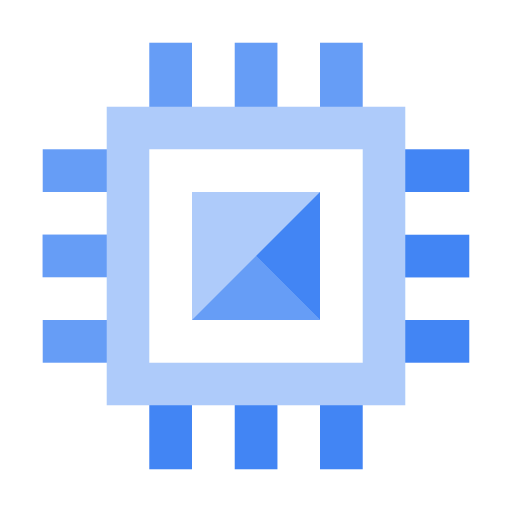
Compute Engine
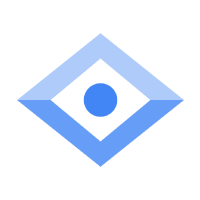
Vision API
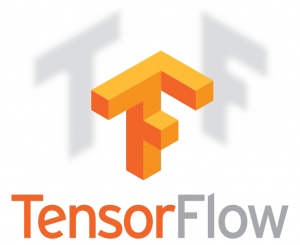
TensorFlow
Solution
Quantiphi leveraged Google’s open-source TensorFlow library, CloudML Engine, and other Google Cloud products to develop a custom deep learning solution that performs intelligent video analytics and provides real-time assistance to crew members. The solution autonomously detects objects and people near and around its 24,000-mile network of railway tracks. Machine learning models were designed to detect signals, flags, crew members, and trespassers present on the tracks as well as store this data in real-time on live dashboards. The Trespasser Detection System helped in identifying trespasser hotspots, enabling crew members to take actions where trespassers are often prevalent. The Crew Assisted Monitoring System allowed for faster and more accurate identification of flags and signals and generated alerts faster than the customer's in-house model.
Result
- Improved detection of trespasser hotspots and reduced theft
- Increased monitoring and safety for employees, consumer goods, and communities