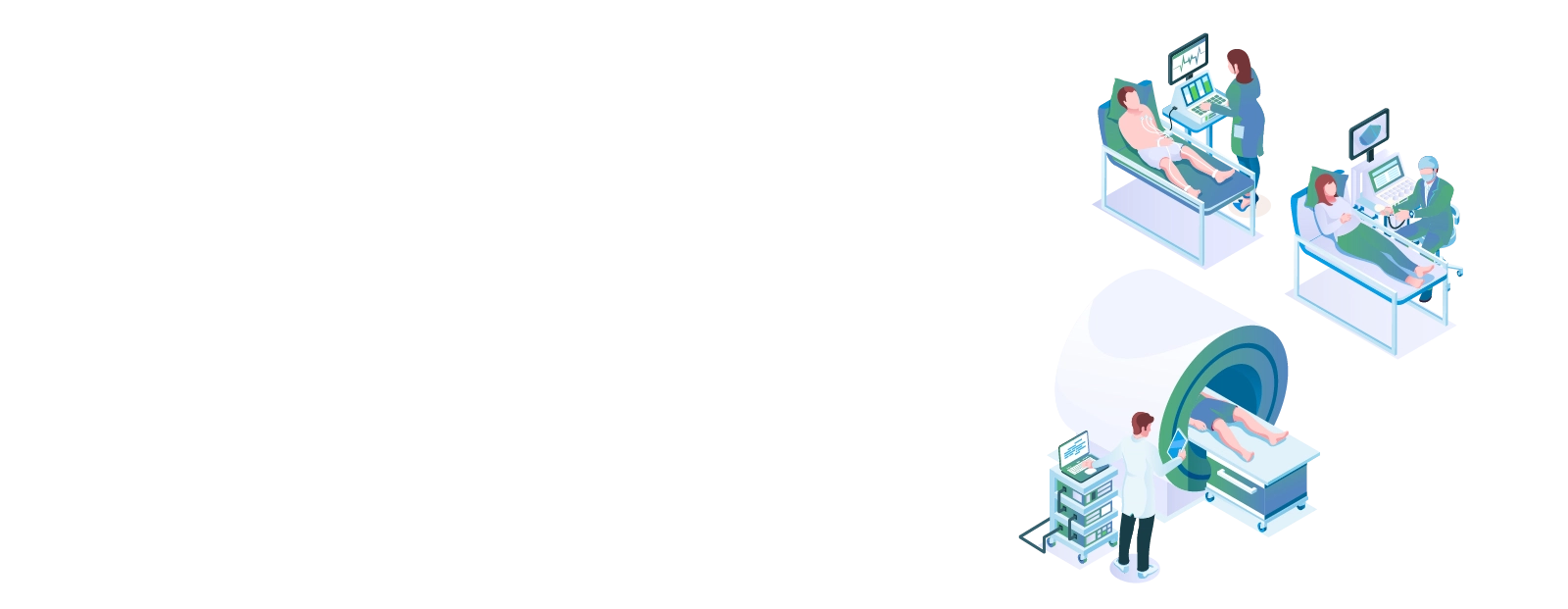
Business Impacts
Significant improvement in fine-tuned LLMs towards understanding the voice of the patient
Enhanced patient and caregiver understanding by pinpointing critical treatment benefits, risks, and burdens
Enhanced efficiency by reducing cloud costs 30% through better training and deployment setups
Enabled improved user journey tracking to strategically navigate and capitalize on the evolving landscape
Customer Key Facts
- Country : Headquatered in Darmstadt, Germany
- Size : >60000
- Industry : Pharmaceutical company
- Rank : One of Fortune 100
Problem Context
Leveraging patients’ growing digital engagement presents an avenue for conducting patient-centric research and refining drug development by comprehending their requirements. Social media stands out as a valuable source of patient and caregiver insights, integral to Patient-Focused Drug Development strategies. (Refer https://www.fda.gov/media/131230/download). This exploration aims to pinpoint critical information sought by patients concerning treatment transitions, psychosocial impacts, and the burdens associated with the current treatment landscape.
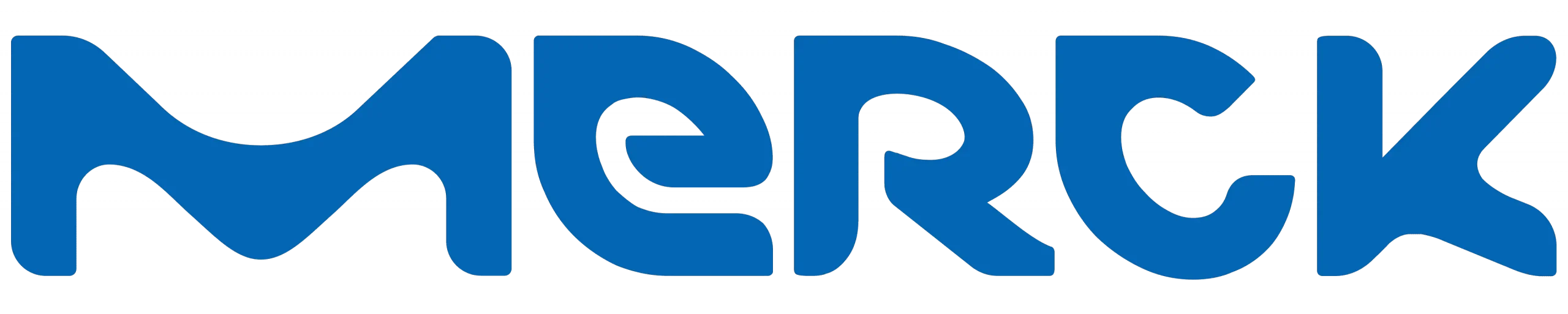
Challenges
- Patient Needs: Current approaches to drug development lack a holistic understanding of patients’ experiences, perspectives, needs, and priorities.
- Use of Social Media information: Identification of relevant data points for downstream AI workflow to generate qualitative insights from social media data.
- Resource Allocation: Allocate personnel, budget and tools effectively for data collection, analysis and engagement within a limited resource environment.
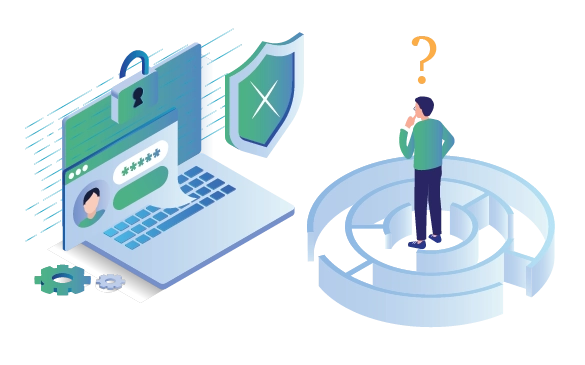
Technologies Used
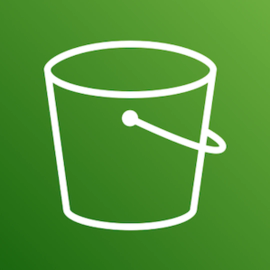
AWS S3 bucket
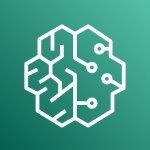
AWS Sagemaker
Qliksense
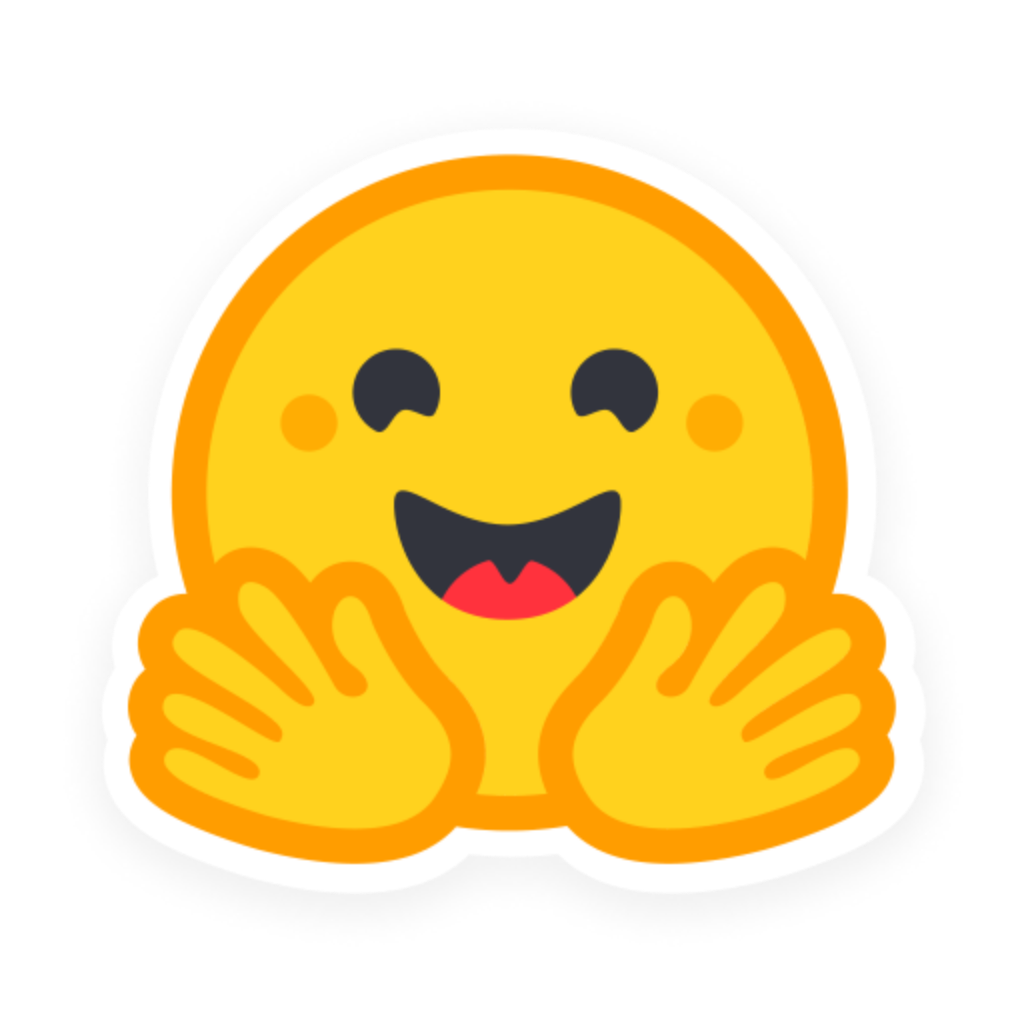
HuggingFace
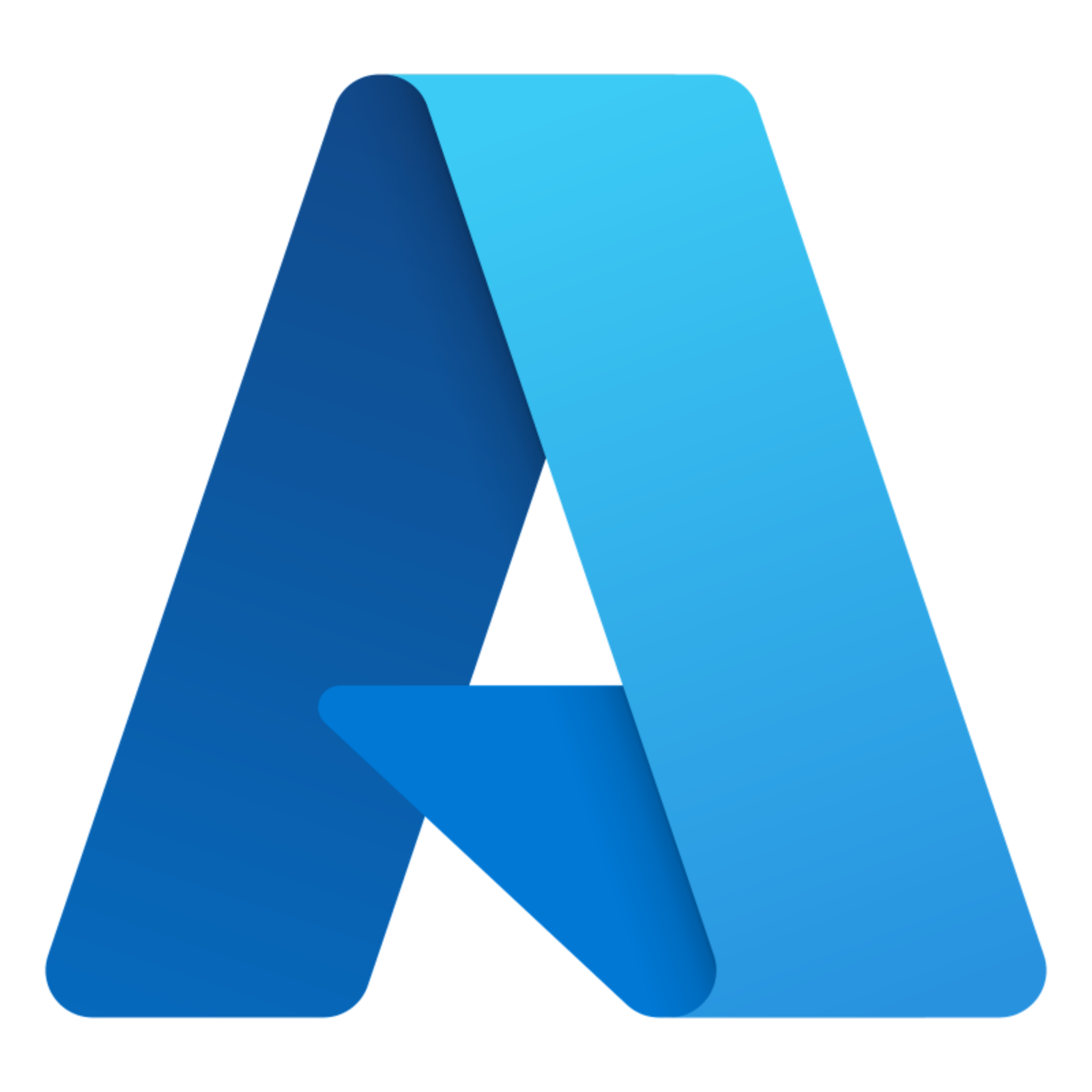
Azure OpenAI services
Solution
To address the challenges associated with hosting and training the resource-intensive GPT-4 model (Refer: https://platform.openai.com/docs/models/gpt-4-and-gpt-4-turbo), Quantiphi developed a lightweight LLM model suitable for commercial GPUs. This solution enables efficient and high-performing model execution, matching or surpassing GPT-4 capabilities for specific tasks.
- Models are selected based on their suitability for fine-tuning and evaluation on a commercial-grade GPU, capacity for swift inference, and alignment with anticipated task outcomes.
- Pinpointed and forecasted instances where changes in treatment were prompted by disease diagnoses. Isolated examples of asset pairs that exhibited transitions from one treatment type to another.
- Identified factors affecting patients' treatment continuance, such as cost, time, travel, job retention, family responsibilities, or specific barriers, no hesitancy.
- Determined and predicted treatment or asset efficacy; categorized outcomes as still exists, recovered, deteriorated, slightly improved, no medical sentiment.
- Identified post-diagnosis psychosocial impact for varying cancer types and labeled them such as anxiety, stress, suicidal tendencies, depression, panic, and no psychosocial impact.
Results
- Using fine-tuned models - we were able to improve performance by 25% and reduce the infrastructure cost by 40%.
- Finetuned models performed better on most NLP tasks than GPT4.
"It was a great experience collaborating with Quantiphi team which showcased their expertise in the area of NLP, machine learning, LLM and prompt engineering for problem statement in the engagement. The team was quite thorough in the experimentation as well as documenting the outcomes throughout the engagement. The team also has decent expertise in the area of managing the deliverables and provide consulting on potential ways forward. Thanks to entire team of Quantiphi for an insightful engagement."
Text Analytics team at Merck Group