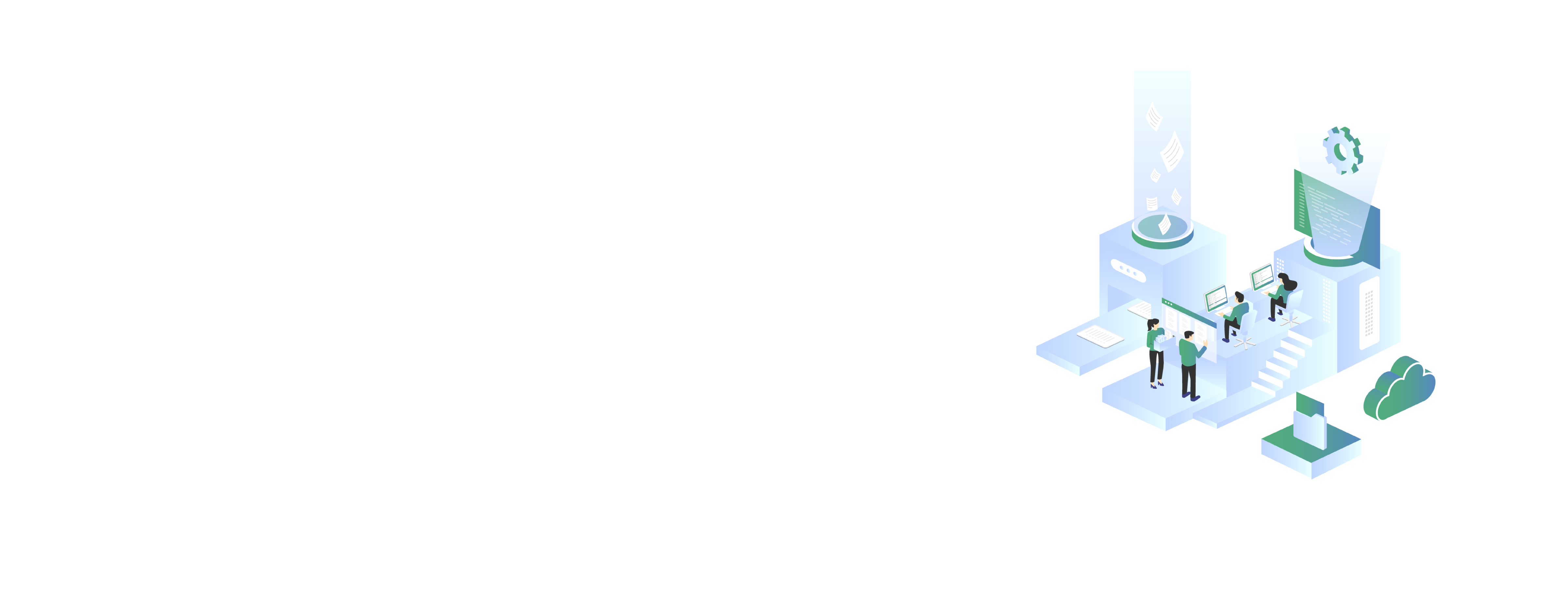
Document Classification & Entity Extraction
Banking & Financial ServicesBusiness Impacts
97.8%
Classification accuracy
700+
Documents in 2 minutes processing speed
Customer Key Facts
- Location : North America
- Industry : Financial Services
Problem Context
The client, a leading federal national mortgage association that receives over one million paper documents a year, including invoices, tax statements, and checks from their customers and vendors was compelled to manually sort and organize the documents. This was posing a risk for fraud that could go undetected due to the large volume and scale of these documents.
Challenges
- Manual effort to digitize and classify 1+ million documents per year
- Entity extraction in a template-free format
- Documents of more than one type might be packaged together or on the same page (i.e. invoices and checks)
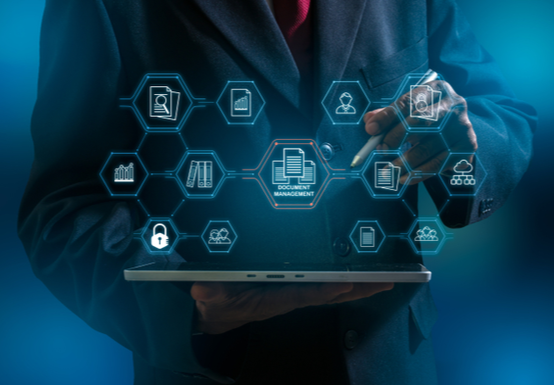
Technologies Used
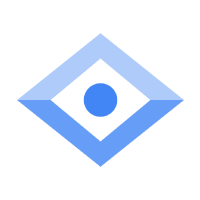
Google Cloud Vision API
Automating the Classification & Digitization of Documents with Document AI
The client wanted to organize their service reimbursement process by automating the digitization of documents and efficiently detecting fraudulent requests.
Solution
Quantiphi developed a machine learning-based custom document classification model to organize and extract information from these documents into a structured dataset at scale.
Result
- Cost optimization
- Time savings
- Enterprise grade accuracy levels for Optical Character Recognition