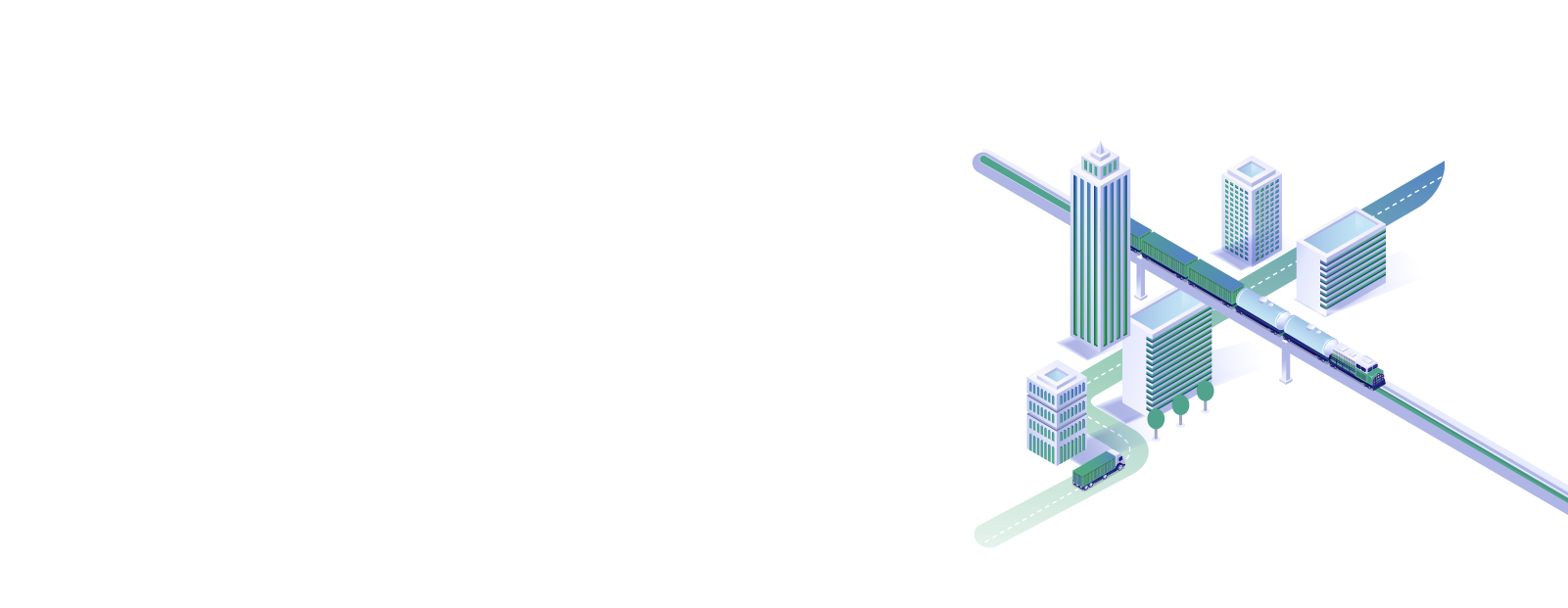
Embedded ML System for Object Detection on Railway Tracks
TransportationBusiness Impacts
90%
Object Detection Accuracy
84%
Object Classification Accuracy
20-22 fps
Rate of Stream Display
6-12 fps
Rate of Predictions from AI Engine
Customer Key Facts
- Location : North America
- Industry : Transportation
Real-Time Video Analytics Solution to Detect Signals, Flags, and Trespassers
The customer is a leading railway company and its locomotive crew needs to manually identify flags and signals, interpret them, and take actions according to the Canadian Rail Operating Rules. The customer lacked a video analytics solution to monitor trespassers on the railway tracks and wanted a solution to identify the concerned objects in frames faster with higher accuracy.
Challenges
- Lack of sufficient training data
- Limited hardware capabilities
- Poor visibility conditions combined with real-time inference
- Extreme class imbalance
Technologies Used
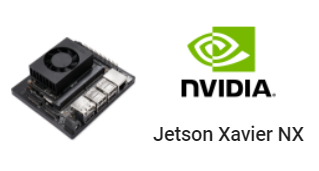
NVIDIA Jetson Xavier NX
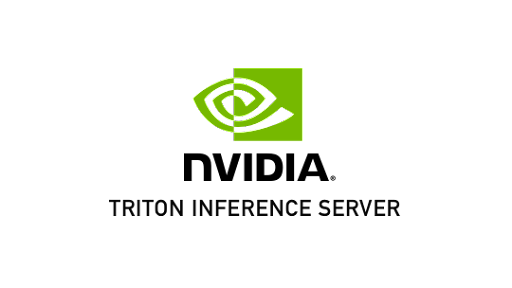
Triton Inference Server
Solution
Quantiphi developed a custom IVA solution to identify trespasser hotspots and detect appropriate signals and flags. This enabled the crew to follow the standard safety guidelines effectively.
The solution was embedded with a real-time inference engine on a Rogue Carrier for NVIDIA® Jetson AGX Xavier that allowed the user to selectively switch between prediction modes (CAMS & TDS). The inference engine was a combination of custom-built object detection, classifiers, and tracking models to provide high mAP while maintaining a smaller memory footprint.
Results
- Faster and more accurate identification of the concerned objects in the frame and quick alerting mechanism
- Identification of trespasser hotspots enables the crew to mitigate regions with high trespassers density