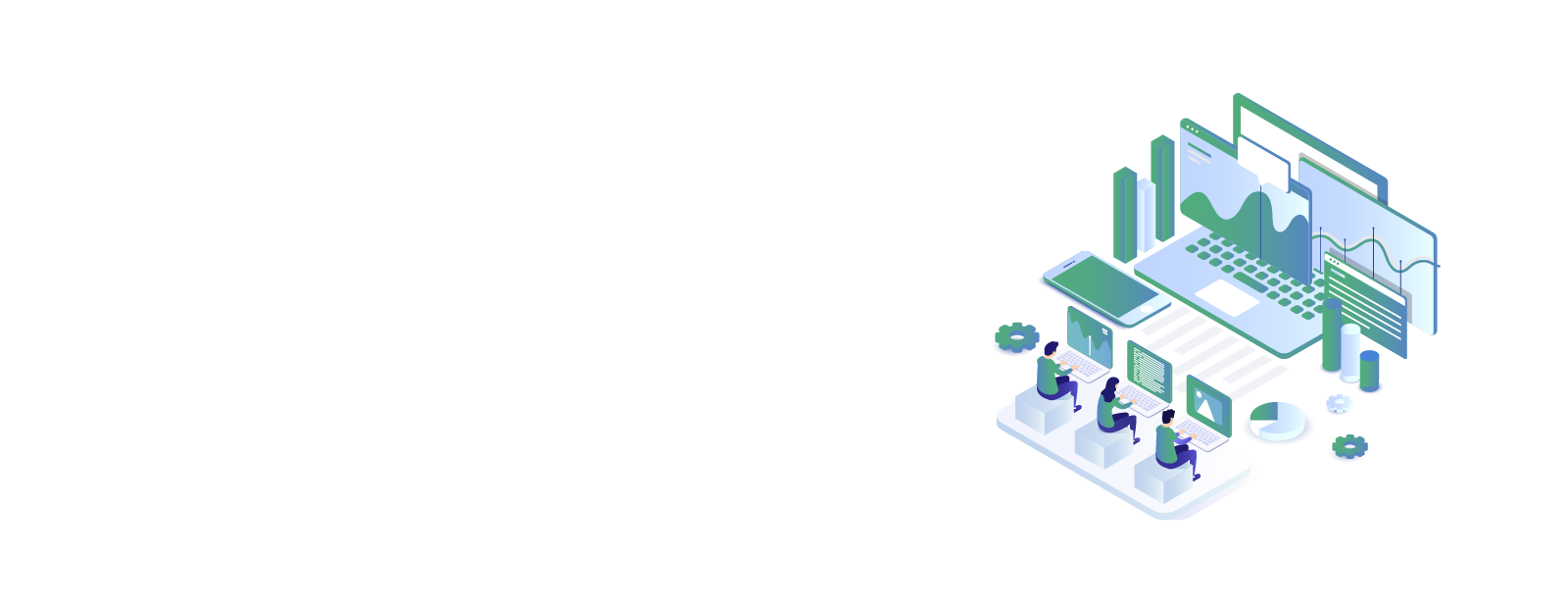
Business Impacts
30%
reduction in downtime
25%
cost saving compared to the previous application
Customer Key Facts
- Country : United States
- Industry : Semiconductor manufacturing
Problem Context
The client, a global semiconductor manufacturing leader, is dedicated to reshaping manufacturing through innovative solutions, specializing in advanced process technology for rapidly expanding markets. They had initially established their data pipeline on AWS, with the goal of transitioning their data sources to Redshift and improving the efficiency of their existing ETL pipeline to handle extensive Terabytes of data processing.
Challenges
- High data volume and a daily load of thousands of Avro and Parquet files
- Different fabrication units and data types within the context of ETL and Ingestion SLA
- Managing query performance, encompassing Upserts and Deletes due to high data volume
- High cost and performance issues due to previous platform licensing
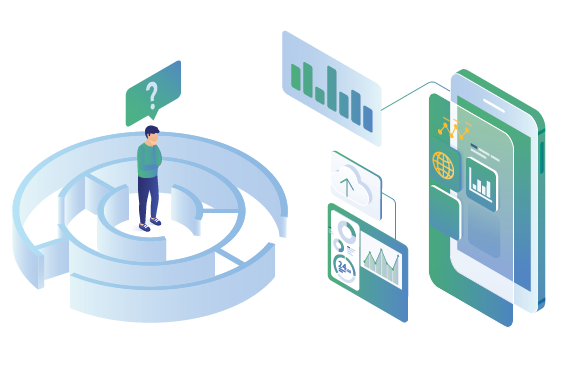
Technologies Used
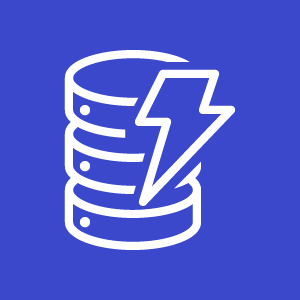
Amazon DynamoDB
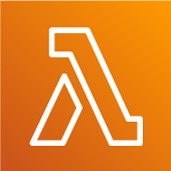
AWS Lambda
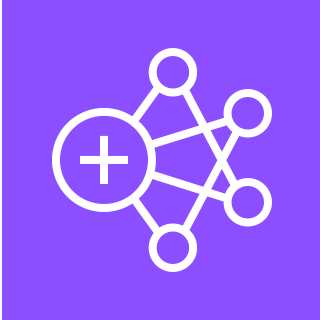
Amazon Elastic MapReduce (EMR)
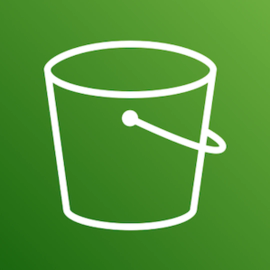
Amazon Simple Storage Service (S3)
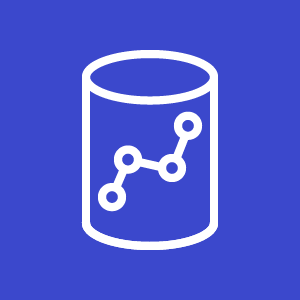
Amazon Redshift
Solution
Quantiphi conducted a comprehensive pipeline re-architecture, leveraging big data technologies such as Spark on Amazon EMR to efficiently process extensive datasets. They successfully transitioned the client's data warehouse to Amazon Redshift, optimizing data management. Additionally, Quantiphi engineered an event-driven framework for streamlined pipeline automation and established a secure AWS Lake House architecture to ensure seamless data ingestion and persistence.
Results
- Improved ETL SLA: Enhancing the ETL pipeline to achieve a 30-minute SLA (Service Level Agreement) target.
- Cost Reduction through Architecture: Leveraging architecture changes to reduce operational costs.
- Efficient Handling of Large Data Volumes: Ensuring the seamless processing of high volumes of data in a single operation.
- Federation Capabilities of Redshift: Leveraging Redshift's support for data federation to consolidate and query data from multiple sources.
- Native AWS Integration: Leveraging Redshift's native integration with various AWS services, including IAM (Identity and Access Management) and S3 (Simple Storage Service), to streamline data management and access.