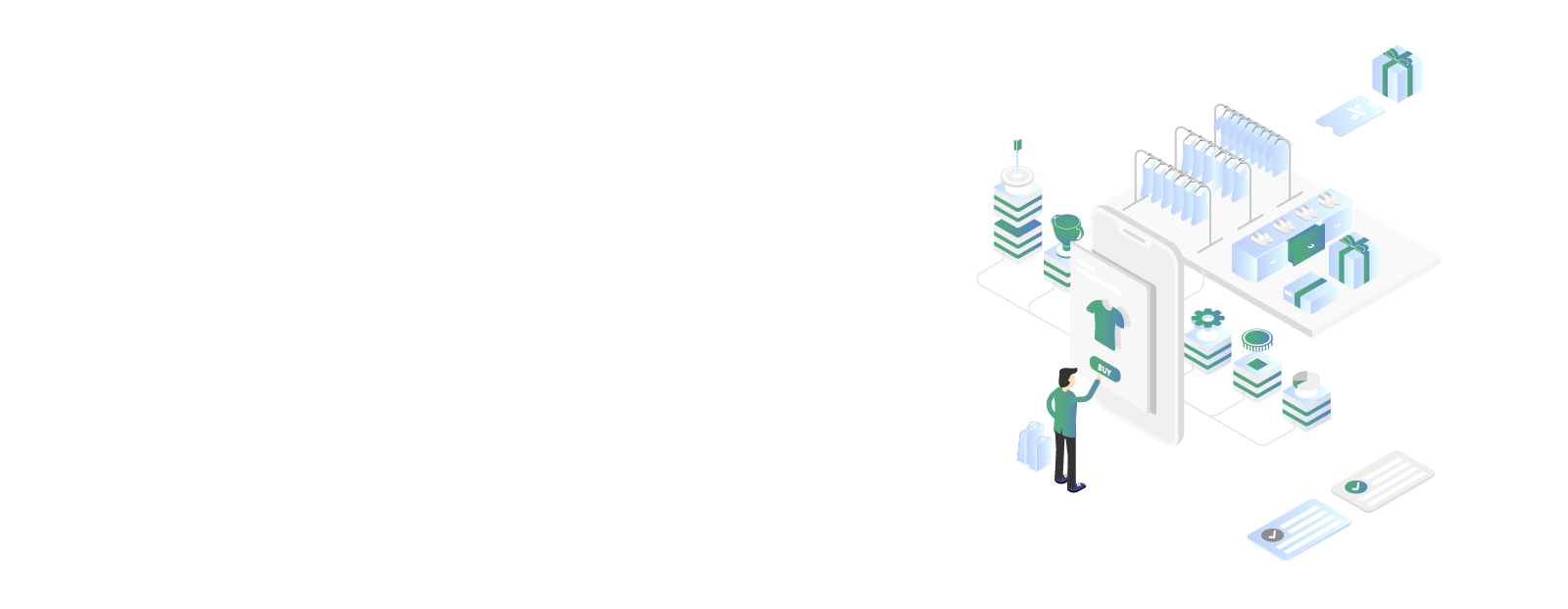
Personalized Recommendation System
Retail & CPGBusiness Impacts
Higher conversion rates for retailers
Higher growth in customer retention
Enhancement in click-through rates
Customer Key Facts
- Location : North America
- Industry : Retail
Problem Context
The customer is a universal shopper profile that built a fashion product platform that enables consumers to search and shop based on product images listed on individual retailers’ websites. However, they had no recommender system in place to provide suggestions based on the product uploaded or clicked by the consumer.
Challenges
- Highly sparse user purchase history and preference data
- Lack of relevant attribute details in product metadata
- Disparate products of multiple retailers
- Aligning recommendations with rapidly changing fashion trends
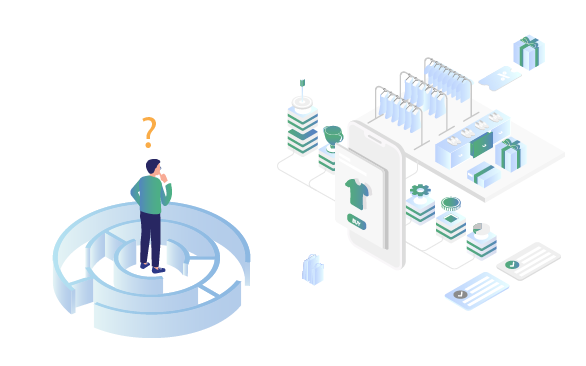
Technologies Used
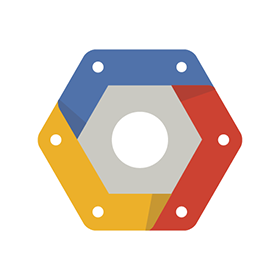
Google Cloud Platform
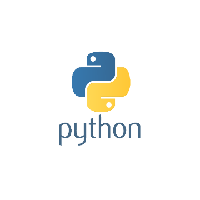
Python
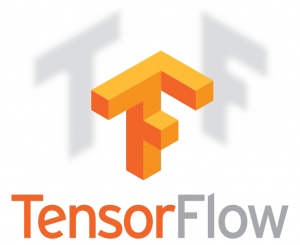
TensorFlow
Combining Fashion and Machine Learning to Develop a Recommendation System That Suggests Products to Customers Based on Their Profile & Preferences
To maintain high-quality user experience, they wanted to leverage highly adaptable ML models that could generate compatible product recommendations for consumers in the absence of co-purchase data by leveraging fashion domain expertise, product images, and textual metadata.
Solution
Quantiphi built a custom recommendation engine that generates personalized fashion product suggestions by analyzing customer clickstream data, uploaded products, purchase history and browsing patterns. In turn, understanding customer style, preferences and wishlists; thus, going beyond analyzing consumption patterns. Using object detection and computer vision, image embedded metadata is collected which is then used to find cosine similarity for suggesting a similar product as well as providing "Complete the Look" recommendations of apparel that can be paired together.
Result
- Hyper-personalization through "Complete the Look" recommendations based on user's universal shopping history and fine-grained preferences
- "Pair with" product suggestions promote cross-selling and boost user engagements