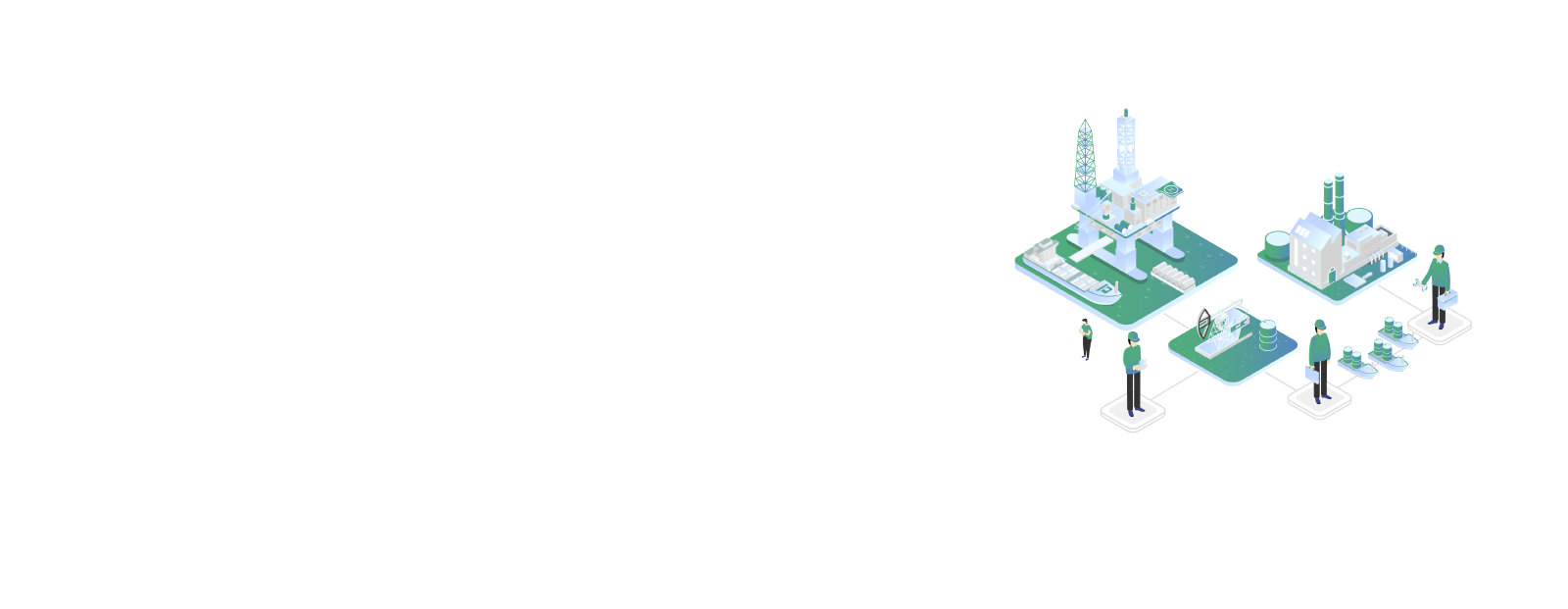
Predictive Maintenance in Oil Rigs
Energy & UtilitiesBusiness Impacts
15s
Near real-time inference of time series telemetry data
5M
Autoscaled data ingestion (in records per second)
Customer Key Facts
- Location : North America
- Industry : Oil & Energy
Problem Context
The customer is an American multinational energy corporation engaged in oil, natural gas, and geothermal energy and produces approximately 160 thousand barrels of oil per day across ten oil rigs, also known as facilities. Approximately 7 to 8 percent of facility downtime in their oil rigs could be attributed to malfunctioning compressors, leading to significant increases in resource and maintenance costs. They wanted to minimize facility downtime by using predictive tools to understand causes of such failures.
Challenges
- Incredibly large volumes of input data
- High throughput required in streaming data with milliseconds of lag
- Customizing third-party application OpenTSDB to increase performance
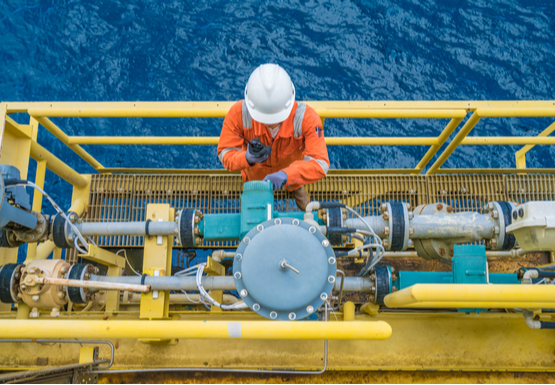
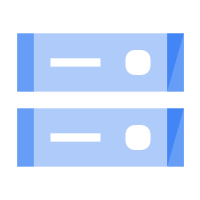
Cloud Storage
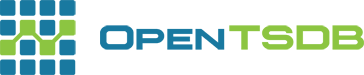
OpenTSDB
Cloud Pub/Sub
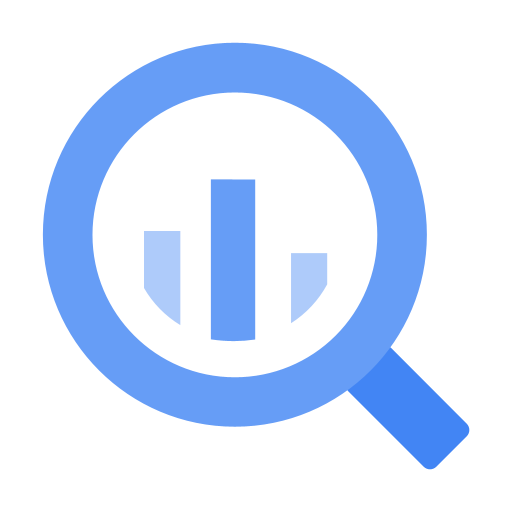
Google's BigQuery
Cloud ML

Google Cloud Dataflow
Cloud Bigtable
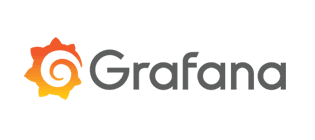
Grafana
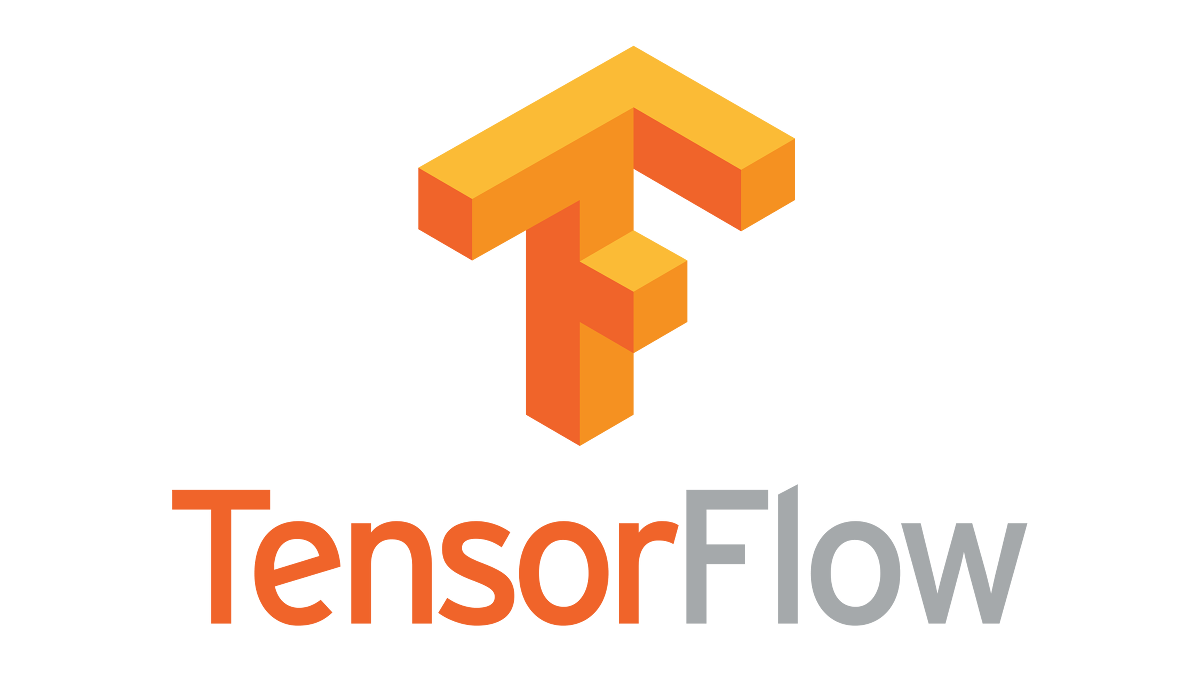
TensorFlow
Data Pipeline and Data Science Workflow to Streamline Processes, Make Predictions, and Visualize Telemetry Data
Solution
Quantiphi built an end-to-end data pipeline from the client's on-prem to Google Cloud Platform (GCP) that routes high throughput telemetry data to GCP APIs, data stores, and visualization platforms. In addition to building machine learning training and serving pipeline to identify patterns in the ingested data and inference, the data science workflow facilitates predictive maintenance.
Result
- Near real-time ingestion of telemetry data
- Minimized facility downtime
- Increased cost savings
- Improved safety of operators