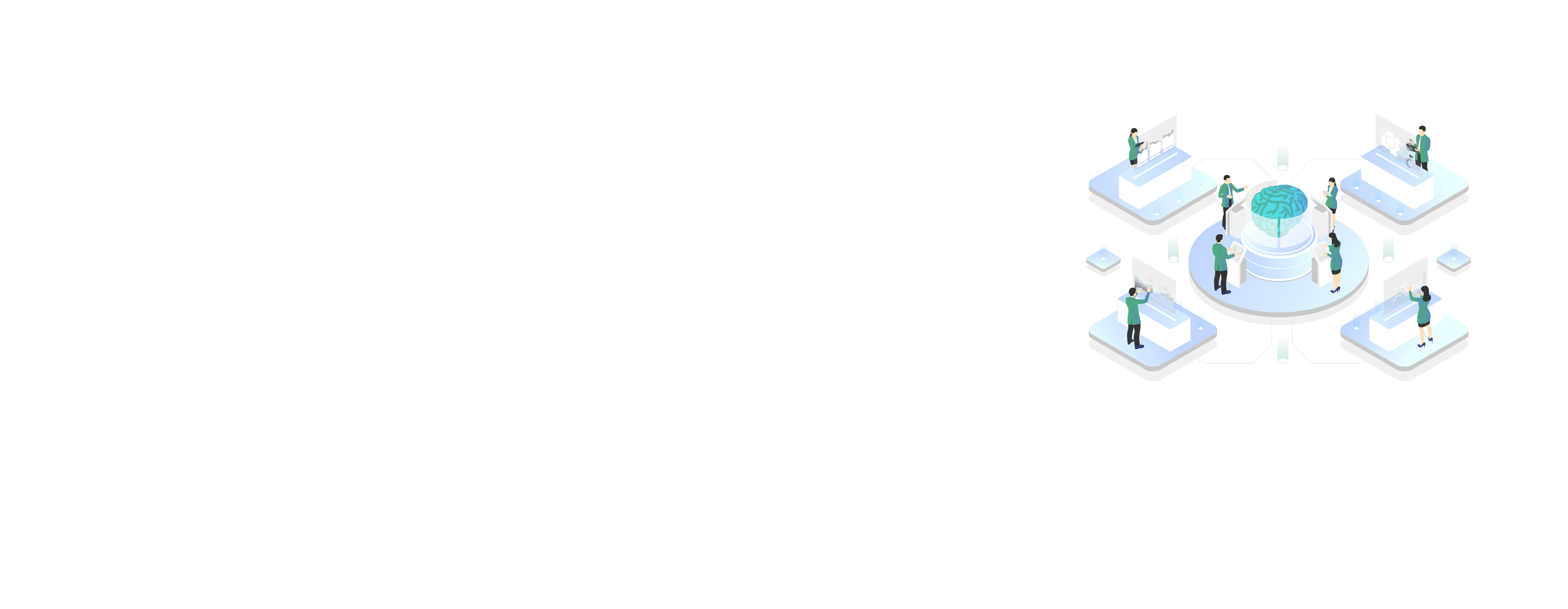
Business Impacts
93%
Model accuracy
99%
Reduction in exam time
Customer Key Facts
- Location : Baltimore, Maryland
- Industry : Higher Education
Problem Context
Johns Hopkins University (JHU) is one of the world’s top universities with a well-known reputation for their research in Bioengineering and Bioinformatics. Their researchers have dedicated decades to develop new treatments, accelerate delivery of care, and improve the quality of life for brain trauma patients. They wanted to leverage AI-enabled software tools that can localize and measure brain hemorrhages from patient CT scans.
Challenges
- Data had been sourced from multiple manufacturers
- Integrating all the manual pre-processing steps into the end-to-end pipeline
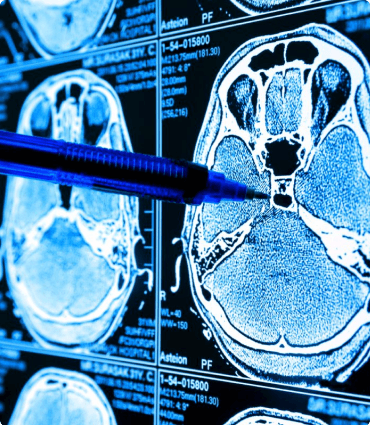
Technologies Used
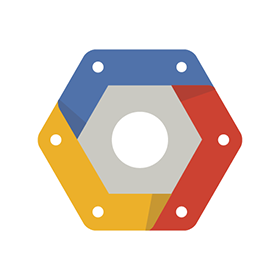
Google Cloud Platform
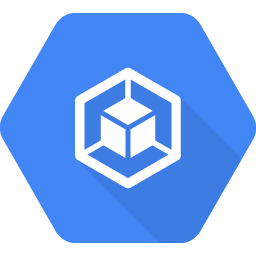
Google Kubernetes Engine
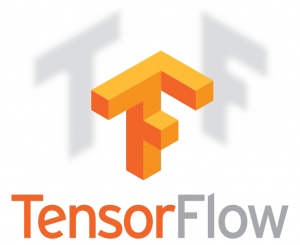
TensorFlow
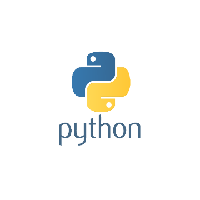
Python
AI-Assisted Medical Imaging for Brain Trauma Patients
Quantiphi partnered with the Johns Hopkins Brain Injury Outcomes (BIOS) Division, a clinical trial coordination center within the Johns Hopkins School of Medicine Department of Neurology, to leverage image segmentation models to detect Intracerebral Hemorrhage (ICH).
Localizing and quantifying ICH is complicated and requires an expert eye to examine DICOM images. The measurement of the blood clot is done manually and subject to human error. Furthermore, manual measurement of a blood clot is time consuming, while neurological deterioration occurs within minutes. The resulting delay in surgery decision-making creates life-threatening scenarios.
Solution
Quantiphi built a scalable machine learning-based medical imaging model that accurately isolates the region of interest and determines the volume of blood clots in a patient’s CT scans. This enabled JHU's BIOS Division to accelerate clinical trial insights for over 500 patients and predict the presence of ICH in traumatic brain injury cases with 93 percent accuracy. As a result, they are able to pinpoint the optimal location for the surgery within seconds, delivering life-saving care to brain trauma patients.
Results
- Faster and more accurate patient diagnosis
- Expedites the surgical decision-making process
- High-compute power processes large volumes of medical images