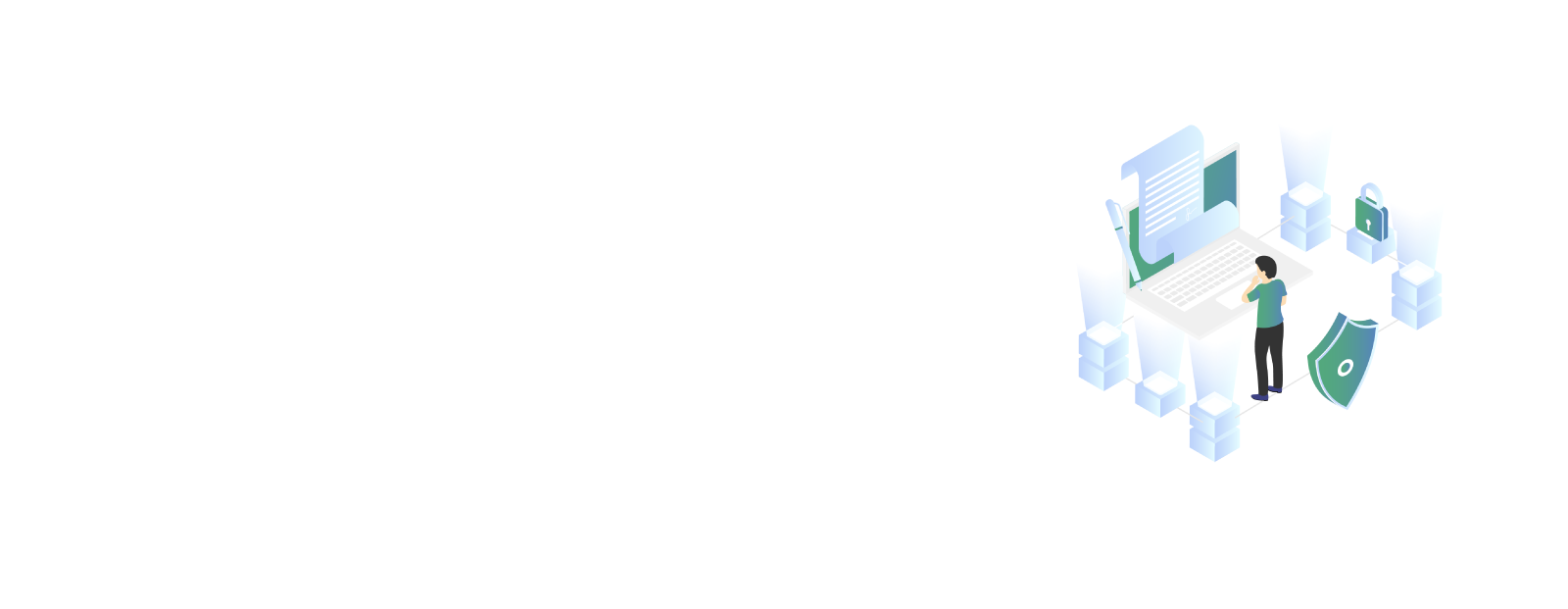
Fraudulent Claims Detection
InsuranceBusiness Impacts
48,00+
Claims analyzed
335+
Potentially suspicious claims identified
20+
Fraudulent claims identified
Customer Key Facts
- Location : North America
- Industry : Insurance
Problem Context
The customer is a specialty insurance company that wanted to understand trends in their data and prepare a model to identify potential fraudulent claims in their workers compensation line of business.
Challenges
- Identify anomalies in the data
- Tag potentially fraud claims based on a set score
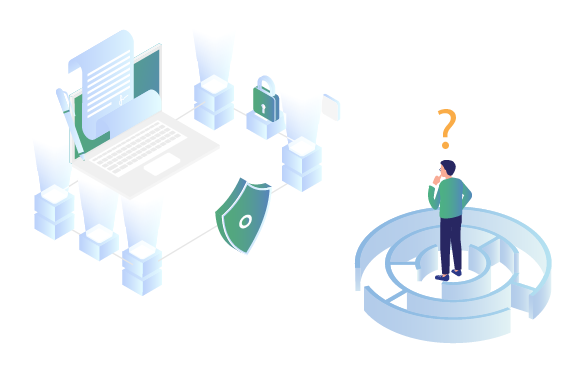
Technologies Used
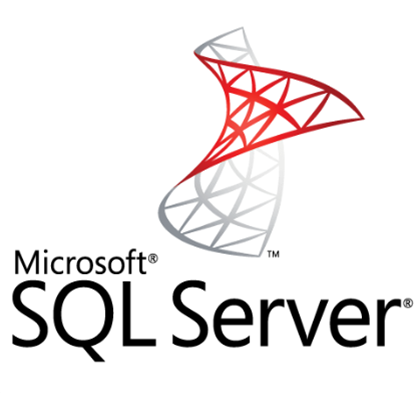
Microsoft SQL Server
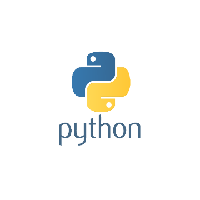
Python
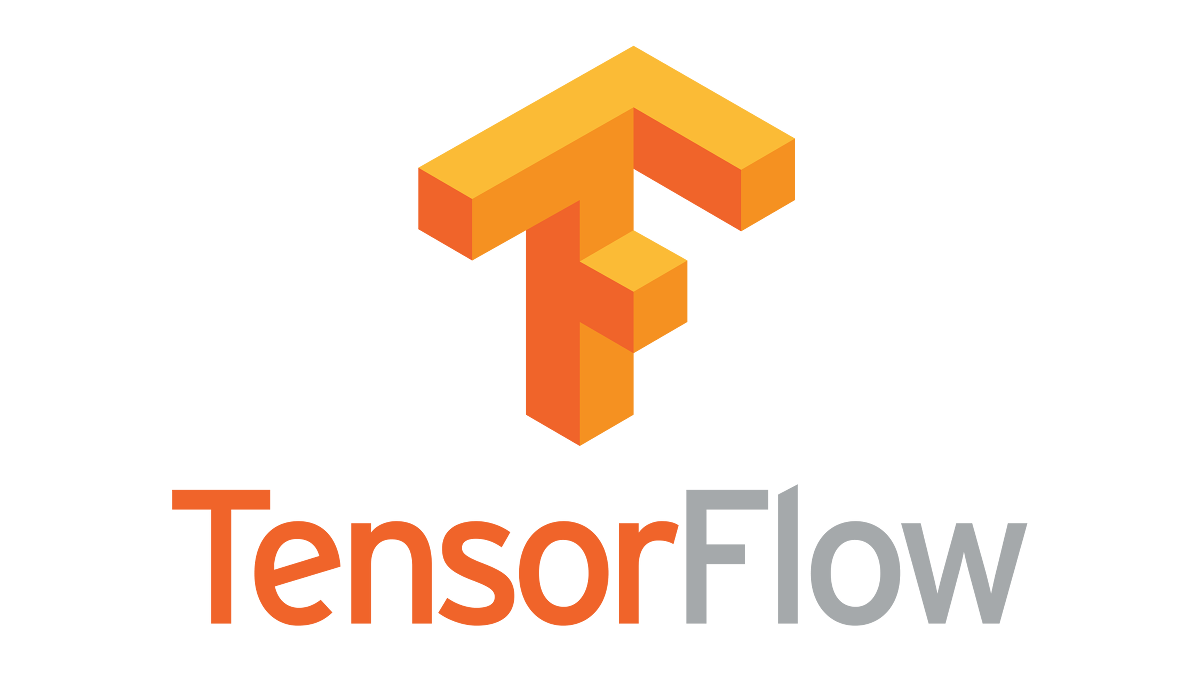
TensorFlow
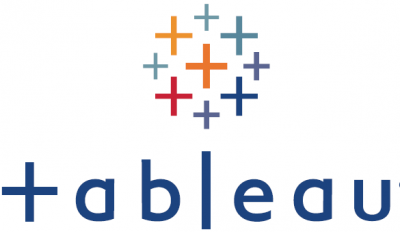
Tableau
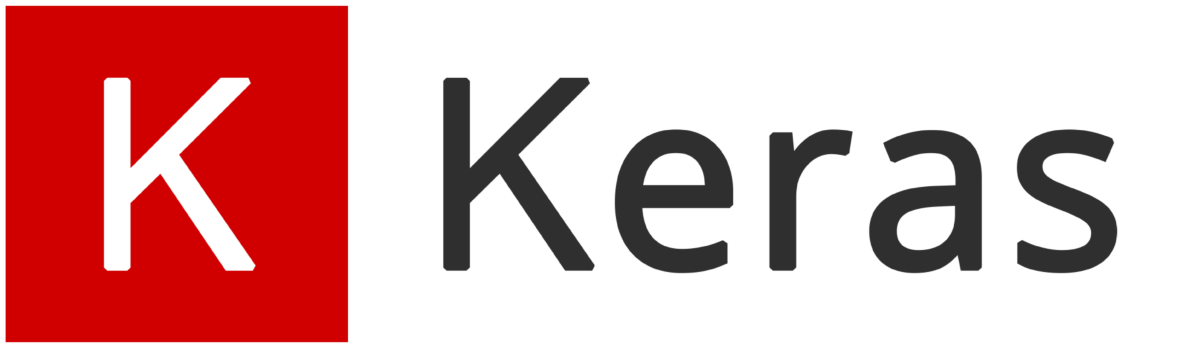
Keras
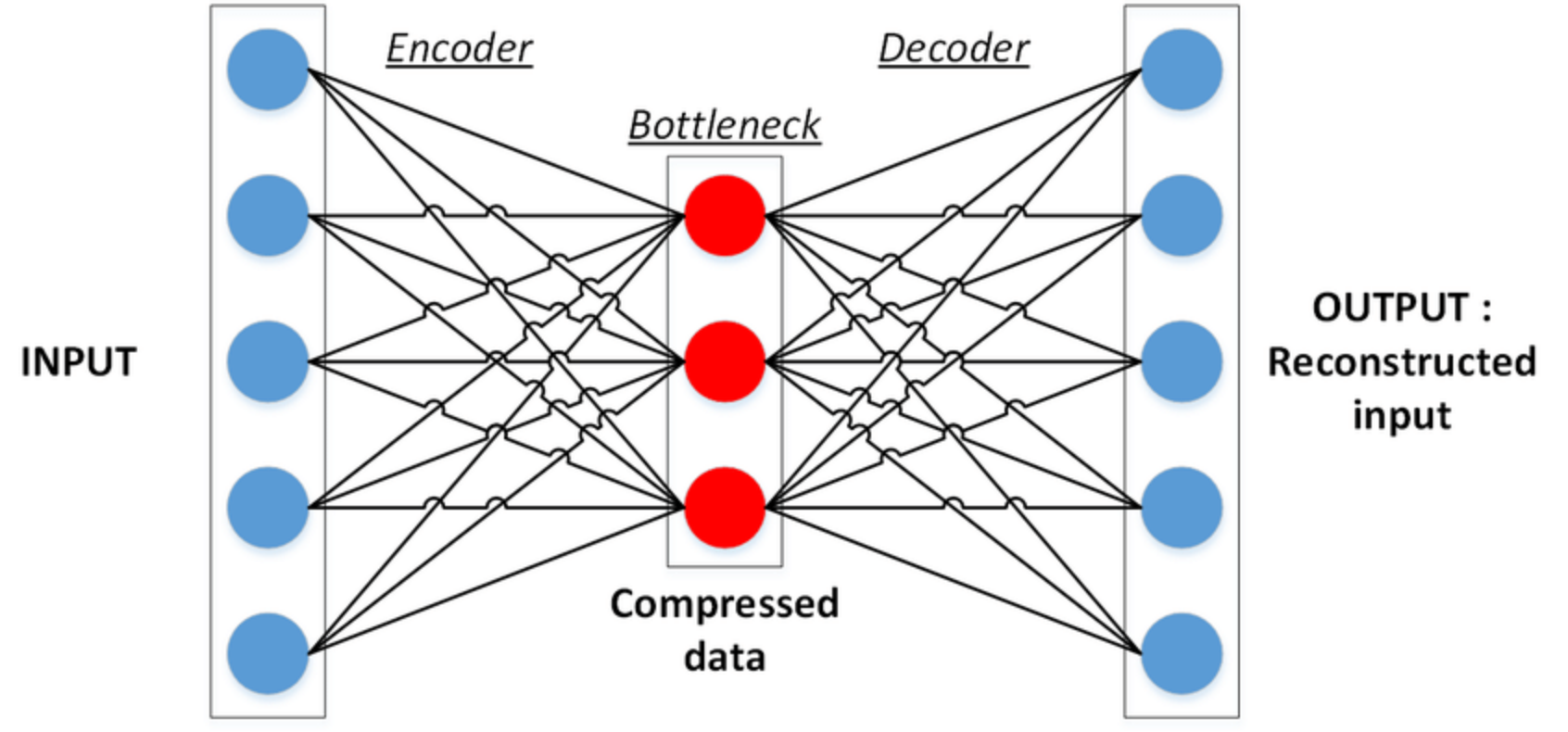
Auto Encoder
Developing a ML model and Rule-based Indicators to Classify Potential Fraudulent Claims
Solution
Quantiphi leveraged various types of the customer's claims-related data, such as Claims note, Claims details, ISO match and Loss run, to train a machine learning model and build a rule/indicators based system to tag potentially fraudulent claims based on a set score. Additionally, we built an unsupervised machine learning model to find anomalies in the data. A Tableau Dashboard was also built to showcase which claims are detected as suspicious.
Result
- Reduced claims payout and expenses owing to identification of fraudulent claims
- Gained the ability to gauge the fraud risk of a claim in real time
- Improved operational efficiency
- Analytics-driven reporting via a BI platform