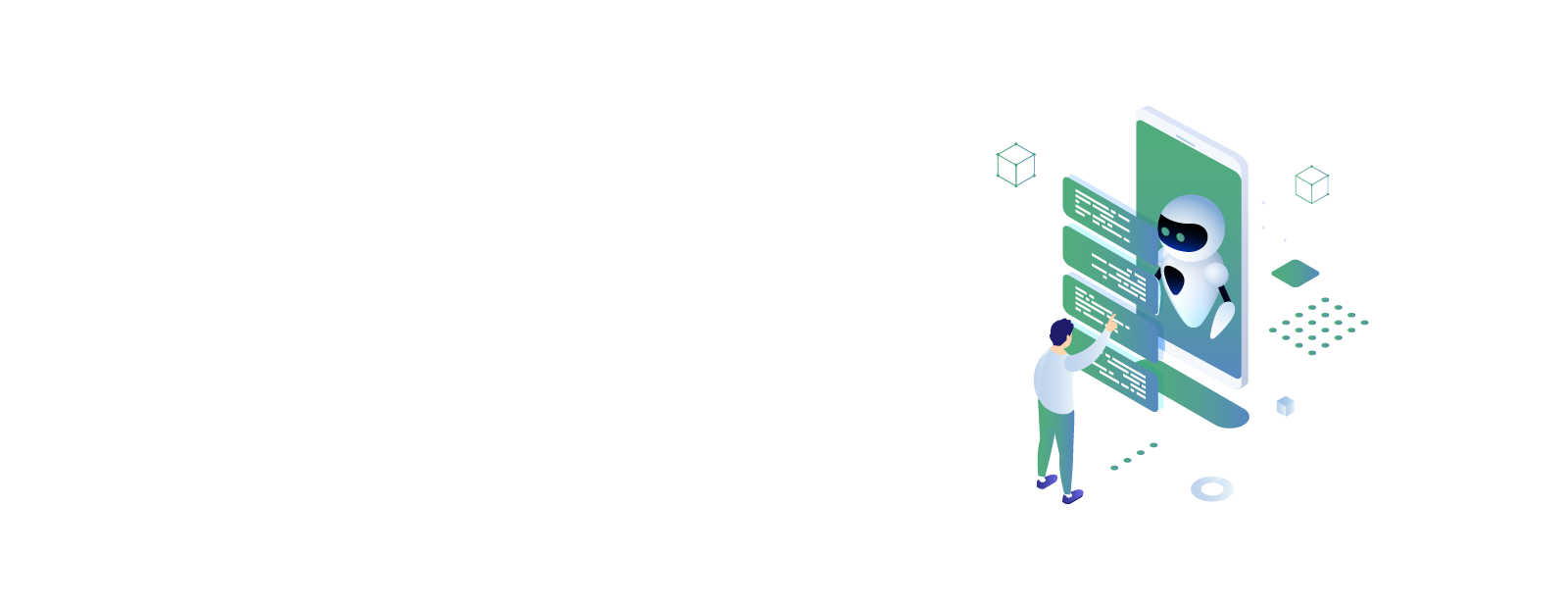
Fully Automated ML Ops Pipelines
ManufacturingBusiness Impacts
Analysis of over two million images per day
Reduced costs with scalable pipeline
Ability to alter the pipeline incorporating both real time and batch predictions
Customer Key Facts
- Location : USA
- Industry : Manufacturing
Problem Context
The client is a leading American producer of computer memory and data storage including dynamic random-access memory, flash memory, and USB flash drives. The client’s Data Science team wanted to perform image-related analysis and needed help to build the “Model Training Flow” section. The customer also wanted to automate continuous monitoring of model performance instead of the existing time-consuming and resource-intensive manual process. There was also a requirement to build a CI/CD pipeline that continuously tests and optimizes the code.
Challenges
- Managed pipelines service of Google was not whitelisted
- Images were not in the required format and size
Technologies Used
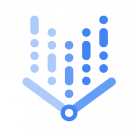
Vertex AI
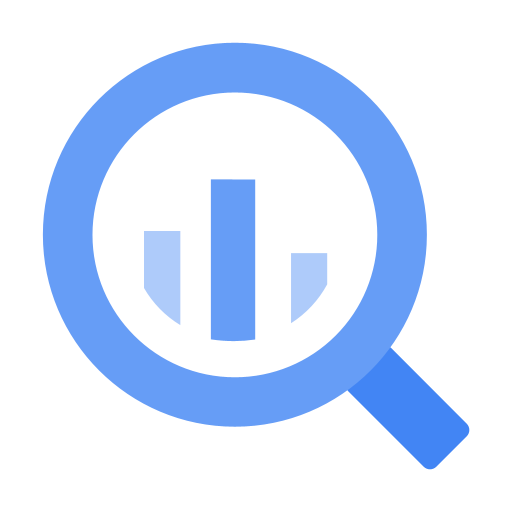
BigQuery
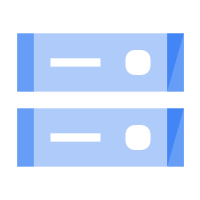
Cloud Storage

Data Flow
Solution
Quantiphi developed a fully-automated MLOps pipeline in Google Cloud Platform (GCP) in two phases to perform analysis of over two million images per day, resulting in faster analysis and quicker defect detection in the images. Phase one of the engagement involved developing an end-to-end semi-automated orchestration pipeline. Phase two dealt with the complete automation of the pipelines that involved continuous evaluation pipelines and the development of CI/CD pipelines. Foreseeing the success of the earlier engagements, the customer wanted help in setting up an AI CoE for advisory on their ongoing projects. In addition, the client also wanted bespoke training for their teams across the US and APAC region.
Results
- Reduced the QC time for the images, manual dependency, and error
- Reduced latency and referencing time
- Facilitated easier data lineage and metadata tracking
- Statistically-determined, automated model monitoring service to minimize human intervention