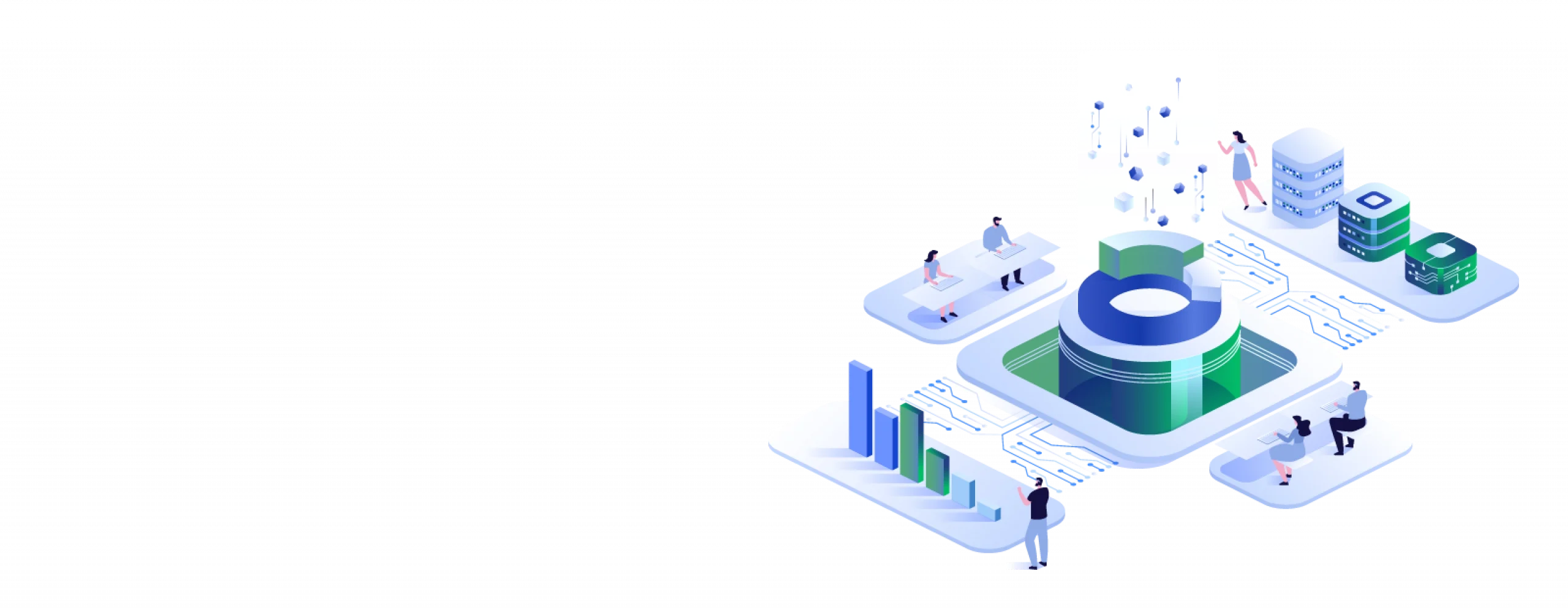
Business Impacts
5x Improvement in inference time with GCP accelerators (TPUs)
Optimized cost-to-performance ratio with GCP accelerators
Up to 93% reduction in model training time using GCP TPUs
Customer Key Facts
- Country : USA
- Size : Startup
- Industry : Technology
- Rank : Named as one of the 50 most promising AI startups in the world in 2023 by Forbes
- Customer Base : Global 2000 enterprises, government, and AI Innovators (5 of the top 10 US banks use Snorkel AI)
- Website : www.snorkel.ai
Problem Context
Snorkel AI’s platform enables data scientists to bring high-quality models to production faster with an iterative, interactive data-centric AI approach powered by programmatic labeling and foundation models. The Snorkel AI Research team wanted to evaluate the impact of running transformer models (CLIP/Owl-ViT) on GCP accelerators. The client aims to improve latency and optimize costs by using GPUs and TPUs in GCP’s preprocessing and training workflow.
Snorkel Al seeks to achieve the following objectives:
1. Explore the possibilities to leverage GCP GPUs and TPUs to accelerate current ML workflows leading to faster inference and training duration
2. Benchmark the results based on costs incurred and time taken
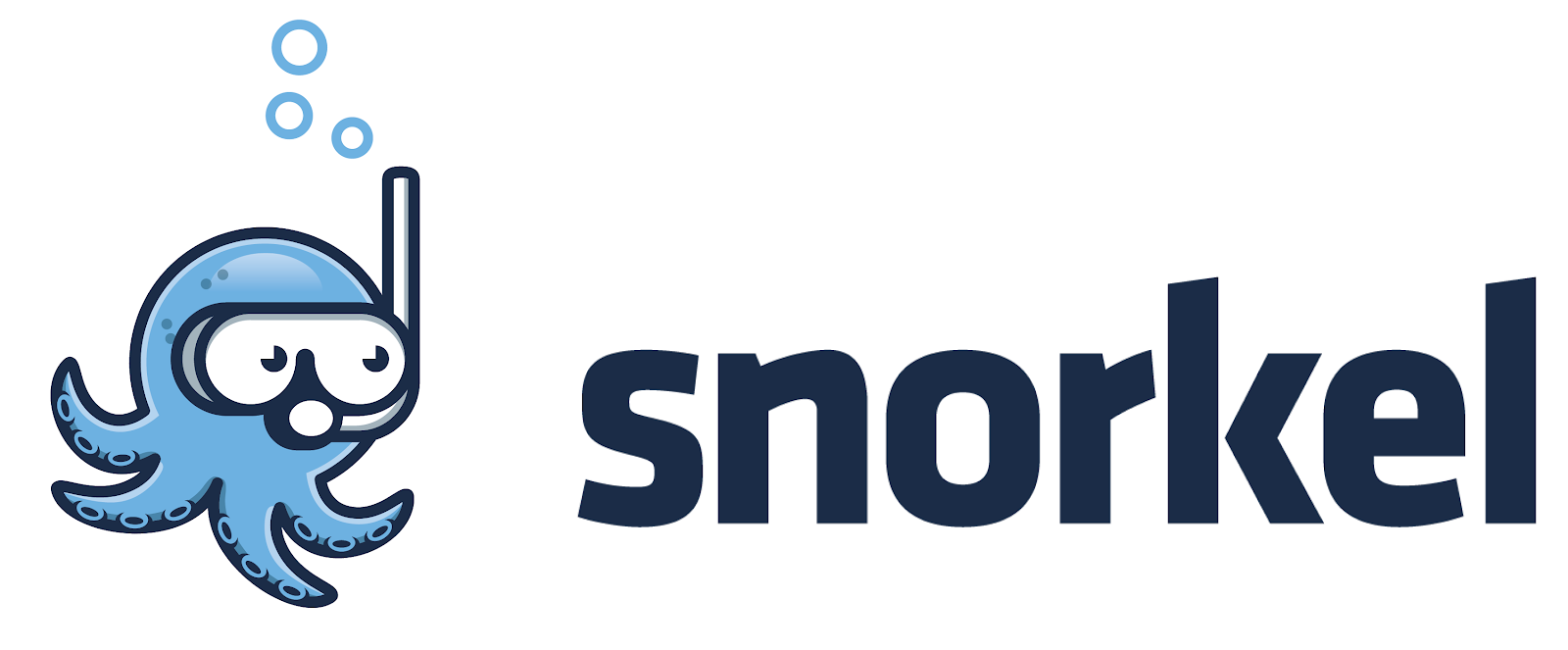
Challenges
- Implementing multi-TPUs and benchmarking the metrics captured
- Effort and resource intensive project with a fixed end date
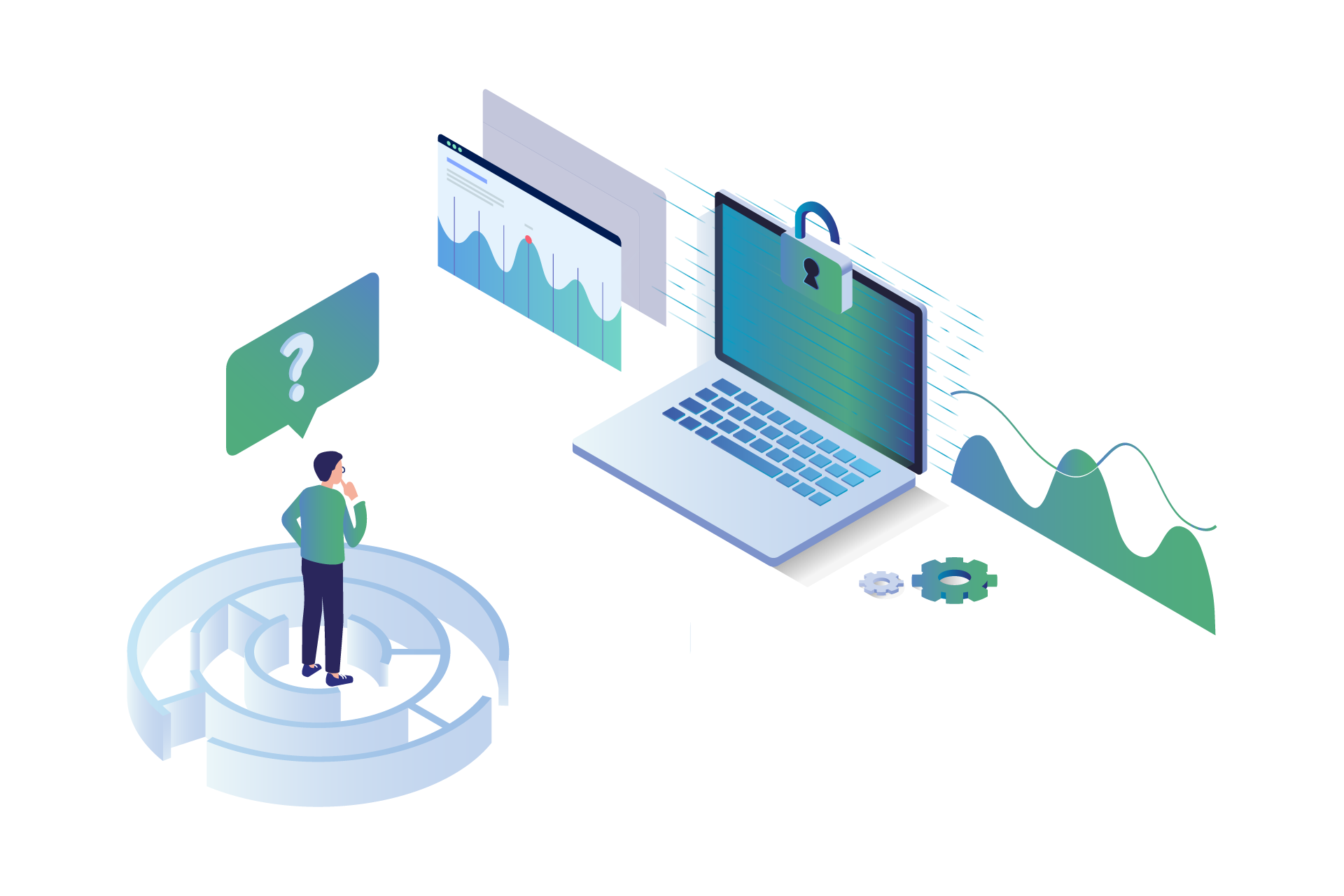
Technologies Used

Google Cloud Filestore
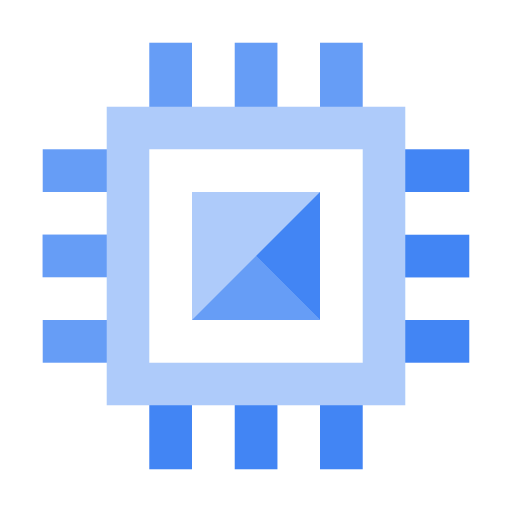
Google Cloud Compute
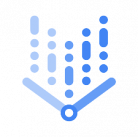
Vertex AI Workbench
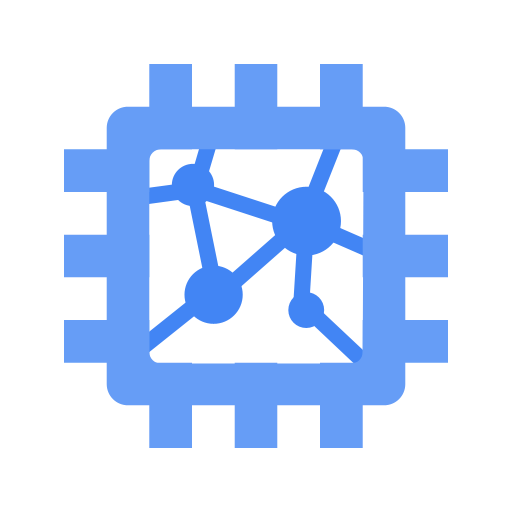
Google Cloud TPU VM

Google Cloud Functions

Google Cloud Scheduler
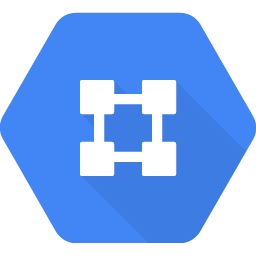
Cloud VPC
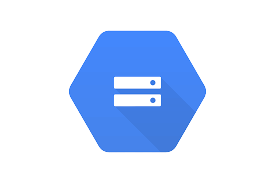
Google Cloud Storage

Cloud Monitoring

Cloud Logs
Solution
Quantiphi worked with Snorkel AI to develop the solution in three phases:
- Phase One: Performing tests on GCP GPUs using CLIP or DETIC
- Phase Two: Compare performance metrics for a single model setup using a TPU VM/Node
- Phase Three: Compare performance metrics for a single model setup using a multi-TPU set-up
As part of the engagement, Quantiphi was successfully able to:
- Demonstrate that GCP accelerators can reduce model training time up to 93% for key use cases
- Demonstrate that GCP accelerators can enable faster interactive workflows for key use cases, ultimately leading to better inference and training throughputs
- Leverage GCP accelerators effectively to optimize the cost-to-performance ratio
- Benchmark the client's existing setup versus GCP accelerators (GPUs and TPUs) and provide a detailed report on the results
Results
- Developed understanding of running models on TPUs
- Migrated critical workloads to GCP
"Quantiphi has been an excellent partner as we explore how Google Cloud TPUs can accelerate AI/ML workloads and enable new interactive workflows for foundation model fine-tuning and training. The Quantiphi team was professional, well-organized, and kept the project on track to ensure completion on schedule. Not only did we have a great experience working with Quantiphi, the project was successful and we saw excellent results on inference and training throughputs."“
Braden Hancock, Co-Founder and Head of Research, Snorkel AI