Executive Summary
Modern engineering operates in a dynamic, data-driven world. Engineering organizations, in particular advanced manufacturing enterprises, are under growing pressure to innovate faster, reduce costs, and improve product quality.
However, traditional approaches to product development and process optimization often rely on time-consuming and resource-intensive methods like first-principles simulations and physical prototyping. These legacy processes hinder rapid exploration of the design landscape, limit adaptability to evolving requirements, and prevent the full realization of digital twin potential for real-time monitoring and control. This bottleneck slows innovation and impacts time-to-market.
That’s where Quantiphi's research-backed Advanced Engineering Neural Operators (ENOs) step in. By harnessing the power of deep learning and integrating physics-based constraints, these cutting-edge models can deliver rapid, accurate simulation and optimization of complex engineering systems.
This translates into significant advantages: accelerated design cycles, reduced development costs, improved product quality, and enhanced innovation in the realm of Industry 4.0 and AI-guided design. In this article, we will explore how Advanced ENOs have the potential to make engineering processes smarter, faster, and scalable.
The Limitations of Traditional Simulation and Deep Learning-Based Methods
- Traditional Simulation Bottlenecks: Conventional methods like Finite Element Analysis (FEA) and Computational Fluid Dynamics (CFD) are computationally expensive, require specialized expertise, and limit accelerated design space exploration.
- Shortcomings in Deep Learning-based Models: While pure data-driven deep learning approaches often fail due to challenges related to data, generalizability; other hybrid approaches including Physics-informed Neural Networks (PINNs) and vanilla Neural Operators often lack the accuracy and robustness required for real-world engineering applications. PINNs require frequent retraining and vanilla operators often struggle with modeling complex non-linear behaviors and are sensitive to data scarcity and quality issues.
The Answer: Advanced Engineering Neural Operators (ENOs)
Quantiphi’s ENOs leverage deep learning, available in-situ data, expert domain knowledge, and known governing equations, to decipher complex relationships and underlying mappings between input parameters (e.g., process settings, material properties, environmental conditions) and resulting outputs (e.g., product performance, manufacturing defects, energy consumption). This capability extends across diverse engineering domains, offering a unified, powerful approach to optimization.
The ENOs overcome the limitations mentioned above through:
- Physics-Informed Architecture: Integrating physical principles ensures predictions adhere to fundamental governing laws, boosting accuracy, reliability, and trustworthiness for critical decisions, while also mitigating data deficiency issues.
- Enhanced Deep Learning Framework: Advanced architectural enhancements, including nonlinear decoders and multi-branch networks customized specifically to the domain and physical phenomena, capture the complex, non-linear dynamics of engineering processes, creating robust and adaptable models.
- Optimized Training Strategies: Advanced training methodologies like curriculum learning and domain decomposition accelerate training, and improve model accuracy for complex system dynamics and geometries, facilitating the seamless integration into existing workflows.
Tangible Business Benefits of Advanced ENOs
- Accelerated Design & Optimization: Rapid, accurate predictions drastically shorten design cycles, enabling faster prototyping, iteration, and informed decision-making.
- Reduced Development Costs: Minimize reliance on expensive physical prototypes and time-consuming simulations, significantly lowering development expenses and accelerating time-to-market.
- Improved Product Quality: Accurate predictions minimize defects, enhance product quality, and drive customer satisfaction.
- Enhanced Innovation: Explore a wider design parameter space, fostering innovation and product differentiation.
- Real-Time Insights & Control: Integrate ENOs for real-time inference, enabling on-the-fly process adjustments and agile, responsive engineering.
Real-World Use Case: Aerospace Composites Curing
Problem Statement: Fiber-reinforced polymer composites are increasingly used in high-performance applications due to their superior mechanical properties, light weight, and durability. Autoclave processing is a common manufacturing method where resin-impregnated fibers are cured under a controlled temperature and pressure cycle.
The goal is to achieve uniform resin cure, optimal resin content, and a void-free product while minimizing residual stress and deformation. However, designing the cure cycle and optimizing tooling for new part geometries or material properties is a time-consuming and expensive iterative process. Traditional computational methods like FEA, while accurate, can be computationally prohibitive for such iterative optimization.
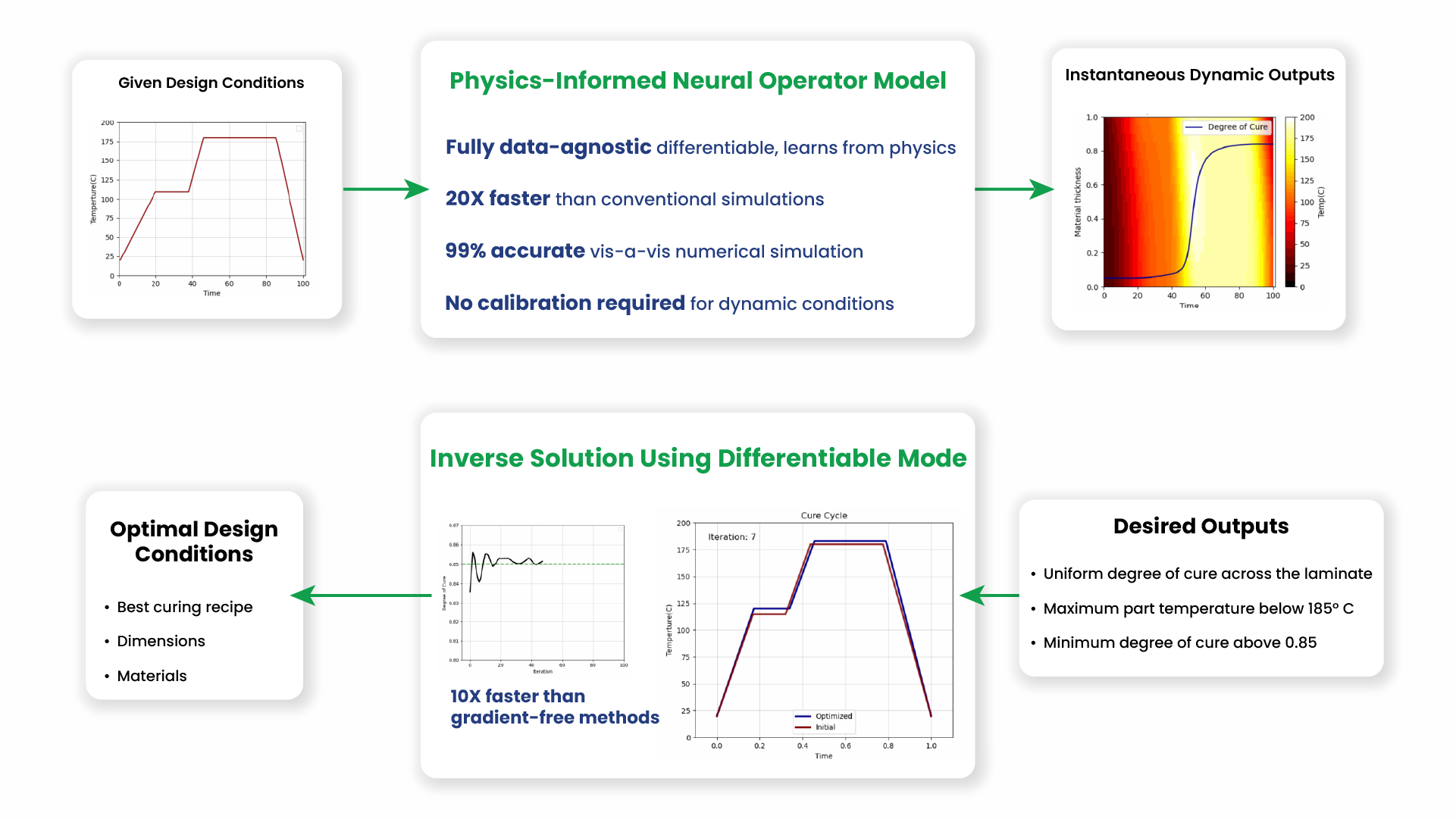
The Solution, Powered by Quantiphi’s ENOs: The ENOs provide real-time and highly accurate predictions for various process configurations, significantly accelerating design optimization and enabling the exploration of a wider range of processing scenarios, tooling designs, and material properties.
For example, engineers can rapidly evaluate the impact of different cure cycle parameters (e.g., temperature ramps, hold durations and temperatures) on the final composite part's properties without running computationally expensive simulations. This allows for quick identification of optimal cure cycles that minimize defects and maximize performance.
By integrating nonlinear decoders, domain decomposition, and curriculum learning, Quantiphi's enhanced ENO framework accurately captures complex solution operators and provides robust, reliable predictive performance for composite curing process optimization.
Tangible Benefits: Quantiphi’s ENOs have demonstrated over 99% accuracy while being 20X faster than conventional simulations. Furthermore, the optimization time is reduced by 10X compared to traditional optimization methods.
Critically, Quantiphi’s ENO framework enables zero-shot prediction capabilities, representing a significant step towards achieving Foundation Model-like performance in engineering. This means the model can generalize to new, unseen scenarios without requiring retraining, further accelerating the design process and reducing reliance on extensive datasets.
Beyond Engineering: Expanding the Impact of ENOs
The impact of Advanced Engineering Neural Operators extends beyond engineering applications, addressing critical needs in diverse areas such as:
- Improved Design & Development: Accelerating the simulation-driven engineering of new materials, processes with tailored specifications for life sciences, healthcare, semiconductor, batteries and other industries.
- Sustainable Manufacturing & Processing: Optimizing industrial processes such as heavy manufacturing, chemical and food processing for minimal environmental impact and reduced energy consumption.
- Predictive Maintenance & Real-time Process Control: Predicting critical equipment failures and optimize maintenance schedules for minimal downtime for critical industries including oil and gas, power and renewable energy. Enabling dynamic adjustments to processes based on real-time data and predictions.
- Urban planning, Policy making, Financial processes: Accelerating simulation and what-if scenario analysis for varied processes including traffic simulation, disease spread, financial forecasts among others.
Quantiphi's Expertise: Driving Innovation in Engineering
Our research-backed solutions have been featured in leading publications and conferences, including Engineering applications of AI, NeurIPS ML4PS workshop 2024, ASME IMECE 2024. Contact us today to explore how our advanced ENOs can revolutionize your engineering and business processes.