The future of healthcare and life sciences is brimming with the potential of AI. From analyzing medical images with incredible accuracy to predicting patient risks and recommending personalized treatments, AI is poised to transform how we approach health. However, as AI’s influence in healthcare deepens, focusing on ethical principles is more crucial than ever.
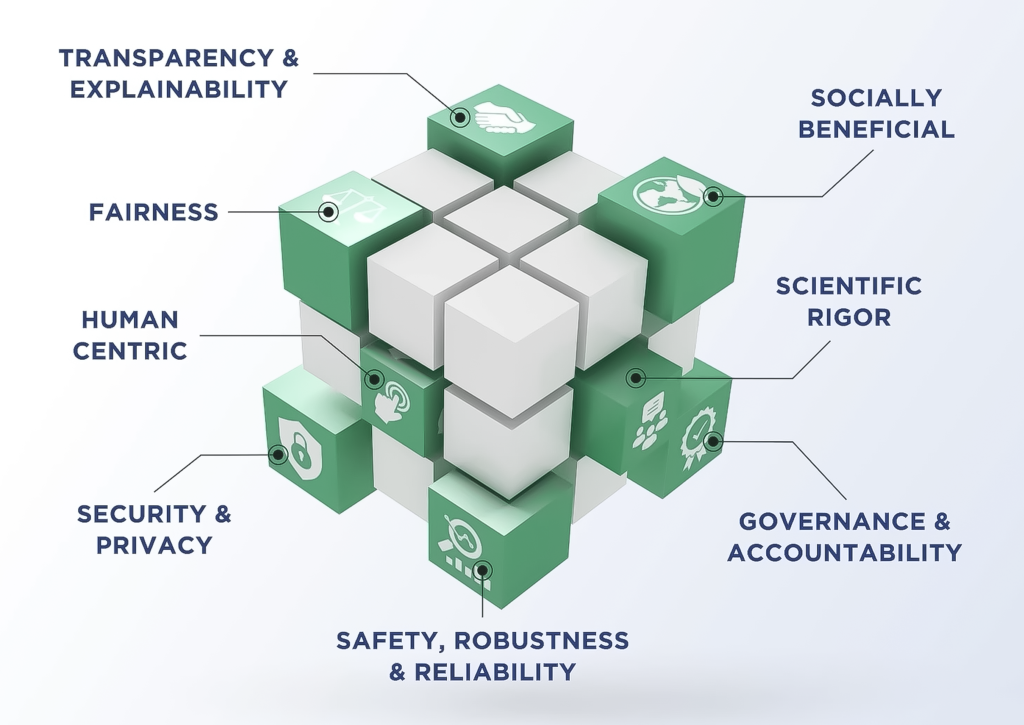
Quantiphi champions Responsible AI development. Our framework integrates ethical and legal considerations throughout the AI lifecycle, from design to deployment. This ensures trustworthy AI solutions for healthcare and life sciences validated through continuous measurement and adjustments. By empowering organizations and stakeholders to innovate responsibly, we foster a safe and ethical environment for advancements in these critical fields. We can ensure this technology serves humanity effectively and ethically through a comprehensive strategy:
1. Security & Privacy: Protecting Patient Data
The foundation of trust in AI-powered healthcare is built on ironclad security and respect for patient privacy. This implies that implementing robust encryption methods and access controls keeps patient information safe. Transparency is key, so responsible AI practices require clear communication about what data is collected, how it's used, and where it's stored.
Fortunately, innovative solutions are helping address privacy concerns. Differential Privacy adds statistical noise to data while preserving its overall trends, allowing for analysis without compromising individual privacy. Federated Learning is another approach that keeps patient data on local devices, with only the insights (not the raw data) shared. This enables collaborative research without centralized data storage, further safeguarding individual privacy.
2. Scientific Rigor: Maintaining Research Integrity
Achieving high standards of scientific approaches in developing AI systems requires close collaboration with the scientific community at large. We can maintain this scientific rigor in healthcare AI through a robust feedback loop between developers and medical professionals. Working with the subject matter experts throughout the AI development cycle fosters a culture of continuous improvement, where potential biases and limitations are identified and addressed promptly. Additionally, real-world data from clinical settings should be continuously fed back into the AI models, allowing them to learn and adapt over time. This iterative process ensures AI models remain clinically relevant and continue to deliver accurate results that align with evolving medical knowledge and best practices. This collaborative approach fosters trust in AI's capabilities and paves the way for its seamless integration into healthcare settings.
3. Safety, Robustness & Reliability: Developing Trustworthy AI
The success of AI in healthcare depends on a commitment to reliability, requiring a comprehensive evaluation of AI models beyond just accuracy to include a range of performance measures. For instance, an AI for pathology medical imaging might excel at identifying ER/PR-positive cells for breast cancer, but not be able to identify PD-L1 cells to support the diagnosis of lung cancer. By considering multiple metrics, we can identify these shortcomings.
Reliability in healthcare AI requires rigorous real-world testing, similar to how new drugs must undergo clinical trials. AI models must also be tested in actual hospital and clinic settings to confirm their theoretical uses translate into practical benefits. This ensures that AI solutions are robust and effective not only in development but in real-world conditions.
The use of Grounding LLMs and Retrieval-Augmented Generation (RAG) further bolsters trustworthy AI in healthcare. By grounding responses in real-world information and potentially incorporating evidence from external sources (through RAG), these technologies enhance the reliability of AI outputs, ensuring consistent and dependable results.
Additionally, the ability of healthcare AI to continuously learn and improve is crucial. As AI systems process new data and feedback from everyday use, they can enhance their accuracy and adapt to changes. This ongoing refinement helps AI tools remain relevant and effective, continually supporting clinical decision-making and improving healthcare operations.
4. Governance & Accountability: Ethical AI Practices
Responsible AI in healthcare requires shared accountability between the developers and deployers of these AI systems and the medical professionals who utilize them. To ensure this, clear guidelines and oversight mechanisms, akin to an ethical framework, should be established. The development of such a framework should prioritize clear guidelines and oversight mechanisms, mirroring the meticulous monitoring of clinical trials for safety and efficacy.
This ethical framework directly impacts the functionalities of AI tools like generative AI. While these tools empower doctors and nurses by automating tasks and streamlining information access, it's crucial to remember that they function as supplements, not replacements. Just as clear instructions and oversight are vital in a clinical setting, they are equally important when utilizing tools like generative AI. This collaborative approach, guided by ethical principles, ensures that AI complements and enhances human expertise within the healthcare system.
5. Socially Beneficial: Leveraging AI for Positive Impact
AI holds the potential to significantly transform healthcare, especially in underserved regions. By prioritizing applications that broaden access to healthcare, it can become more affordable and accessible to those in need. For instance, an AI system capable of analyzing medical images in remote locations can facilitate diagnoses without requiring costly specialist equipment.
However, the potential of AI is not without challenges. Algorithmic bias, if left unchecked, can lead to unfair outcomes. For example, a patient risk prediction tool could perpetuate existing healthcare disparities if its training data is skewed toward a specific demographic. It's crucial to meticulously examine and adjust these algorithms to ensure they serve all patients equitably. By prioritizing positive societal impact and mitigating these potential biases, AI can become a powerful tool for improving healthcare for everyone.
At Quantiphi, we are committed to advancing health equity through AI. We envision a future where AI facilitates diagnoses and treatments with unparalleled precision, targeted interventions champion inclusivity, insights from genomic data advance personalized medicine, and AI-powered command centers allow healthcare professionals to dedicate their expertise where it's most needed.
6. Human Centric: Augmenting Medical Expertise
AI solutions are most effective when they directly address specific human needs and healthcare challenges. By automating routine tasks, AI-powered applications can allow doctors to concentrate on more complex diagnoses and patient care. These tools are not designed to replace a doctor’s judgment but to enhance it, providing relevant data that supports more informed discussions with patients about preventive measures and early detection strategies.
A prime example of this lies in AI-Assisted Medical Imaging. This technology significantly reduces the time radiologists spend analyzing scans, especially for critical cases. Quantiphi partnered with the Johns Hopkins Brain Injury Outcomes (BIOS) Division, a clinical trial coordination center within the Johns Hopkins School of Medicine Department of Neurology, to leverage image segmentation models to detect Intracerebral Hemorrhage (ICH). This partnership underscores the importance of using AI to support—not substitute—radiologists' expertise, leading to more efficient resource use and enhanced productivity. Key medical decisions should always be guided by human judgment and expertise, using AI as a supportive tool to provide valuable insights and help make well-informed choices.
7. Transparency & Explainability: Demystifying AI
To build AI trust within healthcare, it's crucial to design AI systems that are transparent and explainable. This means making it easy for users to understand both how the data is used and how the AI models make decisions. For example, consider an AI that analyzes patient X-rays to detect potential pneumonia. If doctors know that the AI was trained using millions of past X-rays with confirmed diagnoses, understand how it identifies pneumonia-related patterns, and are aware that it was developed with input from researchers and medical experts, they can confidently rely on and interpret its recommendations.
Detailed reports, known as "model cards," which describe an AI model's purpose, strengths, and weaknesses, enhance transparency. As a principle of explainable AI, artificial intelligence systems should provide not only answers but also explanations for their conclusions. As generative AI and complex neural networks become more common, it's important for these systems to clearly explain the steps they take in processing prompts. This helps doctors understand how AI reaches its conclusions, enabling them to make more informed decisions based on AI insights.
8. Fairness: Promoting Healthcare Equity & Eliminating Bias
Bias, whether present in the training data or the AI algorithms themselves, poses a significant threat in healthcare. Imagine an AI system used to assess patient risk factors that unknowingly prioritizes certain demographics based on historical biases in healthcare data. This could lead to unfair risk assessments, potentially delaying or even denying critical care to certain patient groups. To ensure fairness and accuracy, proactive efforts are necessary to identify and address potential biases.
Fostering diversity within AI development teams helps create a broader perspective, increasing the likelihood of identifying potential biases early on. Additionally, acknowledging the inherent difficulty of achieving perfect fairness in AI is crucial. However, this shouldn't deter us. By continuously monitoring and refining AI models throughout development, we can work towards ensuring that this powerful technology serves everyone equitably within the healthcare system.
The Final Diagnosis: A Healthy Future with Responsible AI
Artificial intelligence, when developed and applied with a focus on responsible governance and ethical considerations, has the potential to significantly enhance healthcare and life sciences. From accelerating drug discovery and enhancing diagnostic accuracy to tailoring treatment plans and optimizing healthcare delivery, AI offers powerful tools to empower medical professionals and researchers and ultimately improve patient outcomes worldwide. As this technology continues to advance, ensuring responsible governance of AI through robust regulations, data privacy safeguards, and ongoing human oversight will be critical to realizing its full potential and transforming healthcare for the better. This collaborative effort between technology developers, healthcare providers, policymakers, and the public can pave the way for a future where AI serves as a powerful force for improving health and well-being for all.