Hey there, fellow tech enthusiasts!
The 17th ACM International WSDM Conference took place in Mérida, México, in March 2024, marking a significant event in the realm of web-inspired research focusing on search and data mining. WSDM, one of the premier conferences on web-inspired research involving search and data mining, is a highly selective conference that includes invited talks, as well as refereed full papers. WSDM publishes original, high-quality papers related to search and data mining on the web and the social web, with an emphasis on practical yet principled novel models of search and data mining, algorithm design and analysis, economic implications, and in-depth experimental analysis of accuracy and performance.
Among the innovative minds present at WSDM, one name stood out—Quantiphi! Quantiphi has been creating breakthrough AI technologies and staying ahead of the AI maturity curve helping organizations solve what matters through cutting-edge science for more than a decade. This time our Applied Research team stole the show with three eye-catching posters, each shedding light on groundbreaking advancements in the field, during it's Industry-Day.
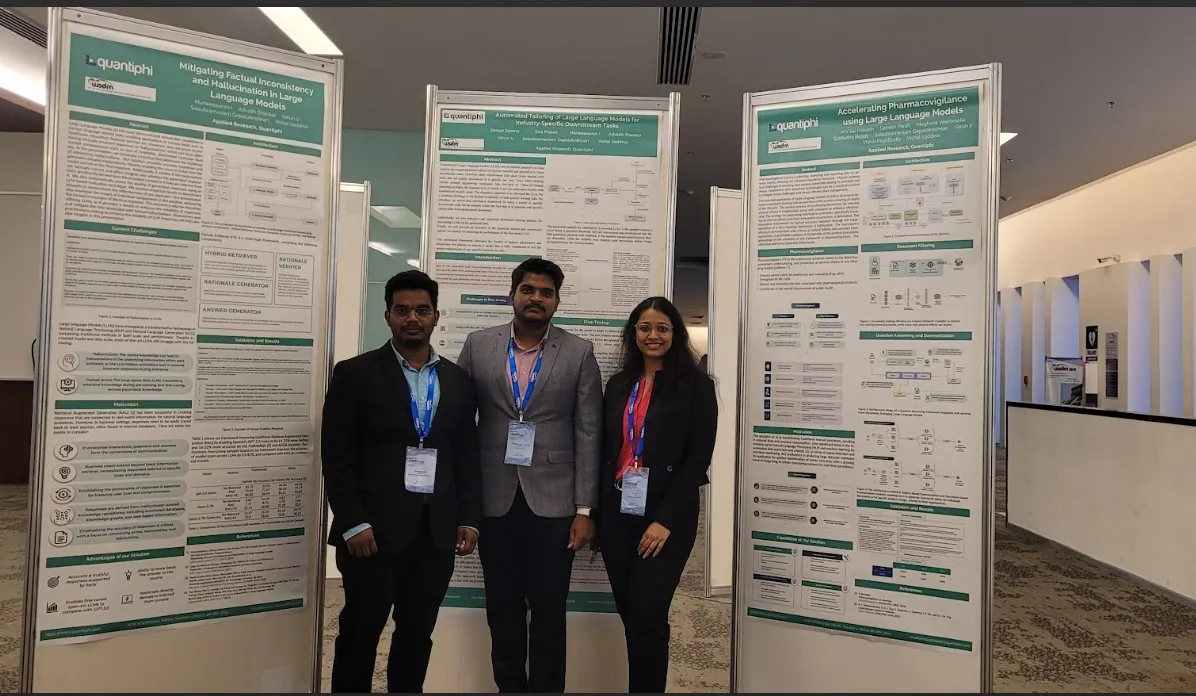
(L to R: Muneeswaran I, MV Sai Prakash, Shreya Saxena)
1. Automated Tailoring of Large Language Models for Industry-Specific Downstream Tasks
Presenter Name: Shreya Saxena, Senior Machine Learning Engineer, Applied Research, R&D, Quantiphi
Summary of Poster:
Foundational Large Language Models (LLMs) are pre-trained generally on a huge corpus of data encompassing broad subjects to become versatile. However, their effectiveness falls short when dealing with tasks that are highly specialized to a specific use case. One approach to combating this is using various prompt engineering techniques, such as few-shot and chain-of-thought reasoning prompts, but this is not sufficient for getting optimal results. An alternative approach is fine-tuning a large language model to a specific use case, but a common challenge here is the limited availability of task-specific training data.
Hence, we propose an end-to-end automated framework to train an LLM tailored for specific use cases. At its core, in the first step, this framework leverages unstructured data to generate task-specific datasets, sidestepping the challenge of limited training data. This data is then leveraged by our optimized distributed training pipeline for fine-tuning the Large Language Model. The framework also evaluates the performance of the fine-tuned model through a range of statistical and customized metrics providing an insight if the model is performing as expected.
This automated framework alleviates the burden of manual adjustments and streamlines the process to provide a model that is fully customized to suit the unique requirements of any specific business use case.
Link to Poster
2. Accelerating Pharmacovigilance Using Large Language Models
Presenter Name: MV Sai Prakash, Senior Machine Learning Engineer, Applied Research, R&D, Quantiphi
Summary of Poster:
Pharmacovigilance is the practice of monitoring, assessing, and preventing adverse effects or any other drug-related problems. Pharmacovigilance ensures the post-market safety of pharmaceuticals and plays a crucial role in public health by enhancing drug safety. This process inculcates manual systems and faces multiple challenges in handling data volume, potentially leading to oversight and delays. Automation with advanced technologies can be a practical solution to mitigate these challenges and ensure efficient data management.
Hence, we propose the application of Large Language Models (LLMs) in pharmacovigilance. Our solution uses a pre-trained LLM to sift through a substantial corpus to discern and retain only a subset of the documents that are relevant to the subject matter. After identifying the relevant documents, the information is synthesized into a summary, delineating salient adverse effects and the events that led to them. Following the generation of the summary the information is validated through a novel fact-checking mechanism to ensure an accurate response.
This system empowers healthcare professionals to analyze adverse effects summaries and reports with ease and speed to enhance patient safety. It also alleviates the burden on the assessor, allowing them to allocate more time to analyze the underlying patterns. Moreover, it expedites the dissemination of critical information, ensuring timely interventions.
Link to poster
3. Mitigating Factual Inconsistency and Hallucination in Large Language Models
Presenter Name: Muneeswaran I, Research Engineer, Applied Research, R&D, Quantiphi
Summary of Poster:
Large Language Models (LLMs) have demonstrated considerable prowess across various language tasks, finding applications in diverse sectors such as healthcare, education, and finance. However, they are susceptible to generating factually incorrect responses, known as "hallucinations," which can undermine credibility and erode trust. One approach to mitigate this is employing a Retrieval Augmented Generator (RAG) pipeline. However, in business settings, to ensure confidence in the responses, they need to be easily traced back to their sources.
Hence, we propose a multi-stage framework capable of retrieving pertinent information (context) from diverse sources like documents, knowledge graphs, and the internet based on user queries. This information is given as input to a novel multi-step approach which is capable enough to generate a rationale, verify the factual accuracy of the same and correct it based on the context if required. The verified and refined rationale is then used to facilitate the LLM to generate the final descriptive response ensuring factual accuracy of the answers. The framework also generates a list of references and citations from the data sources, enabling users to cross-verify information. This multi-stage framework addresses the problem of hallucinations in LLMs by getting more accurate and plausible responses while providing a transparent explanation for the LLM’s decisions.
Link to poster
