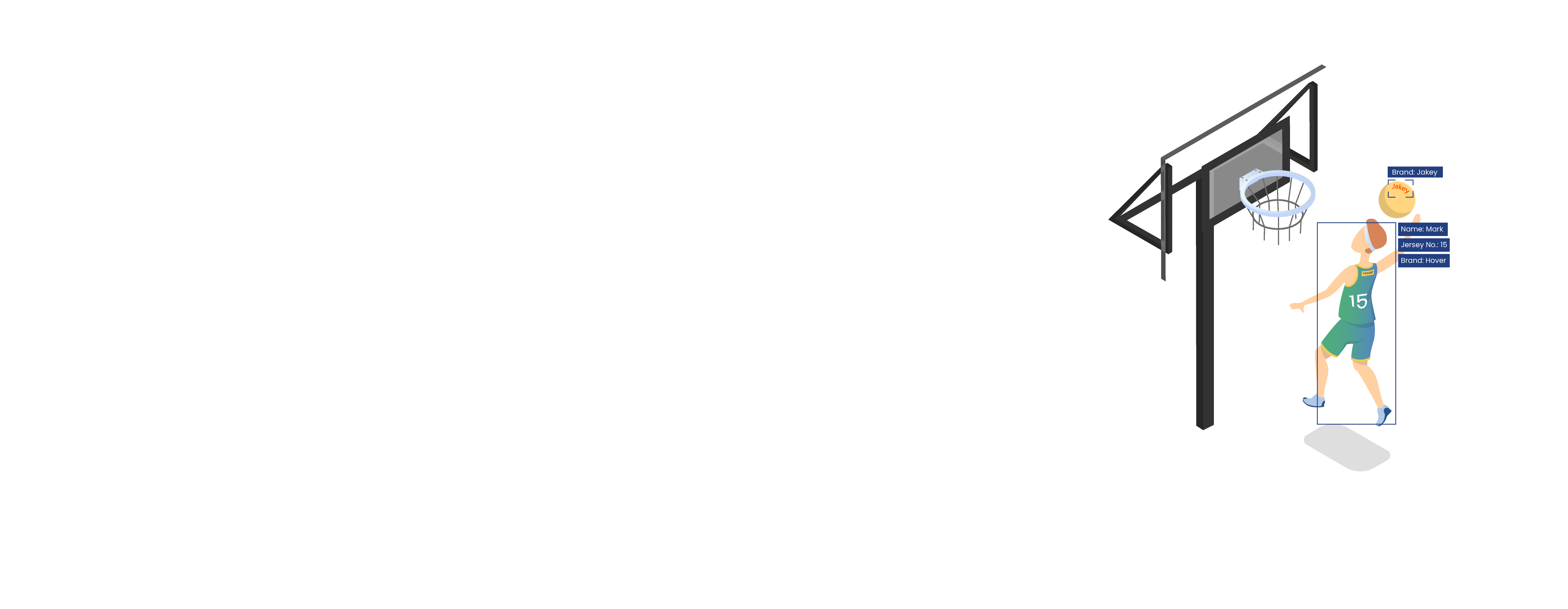
Brand Identification Using Image Classification Model
RetailBusiness Impacts
90% reduction in process time compared to legacy solution
70% reduction in manual tagging efforts
High scalability to large numbers and complex brand logos
Customer Key Facts
- Country : United States
- Industry : Retail
Problem Context
The client owns the leading marketplace platform for pre-owned and new luxury retailers to source and sell authentic, pre-owned luxury products, apparel, and accessories. Their online wholesale platform is the largest and most trusted platform for businesses to connect with global suppliers. However, with the rapid growth of the B2B wholesale unit, operational inefficiencies could be foreseen. They had a model for entity extraction of a title from an item and wanted to utilize an ML-based brand classification model to identify different brand styles.
Challenges
- Lower identification accuracy due to complex datasets.
- Switching of product style identity during the identification process.
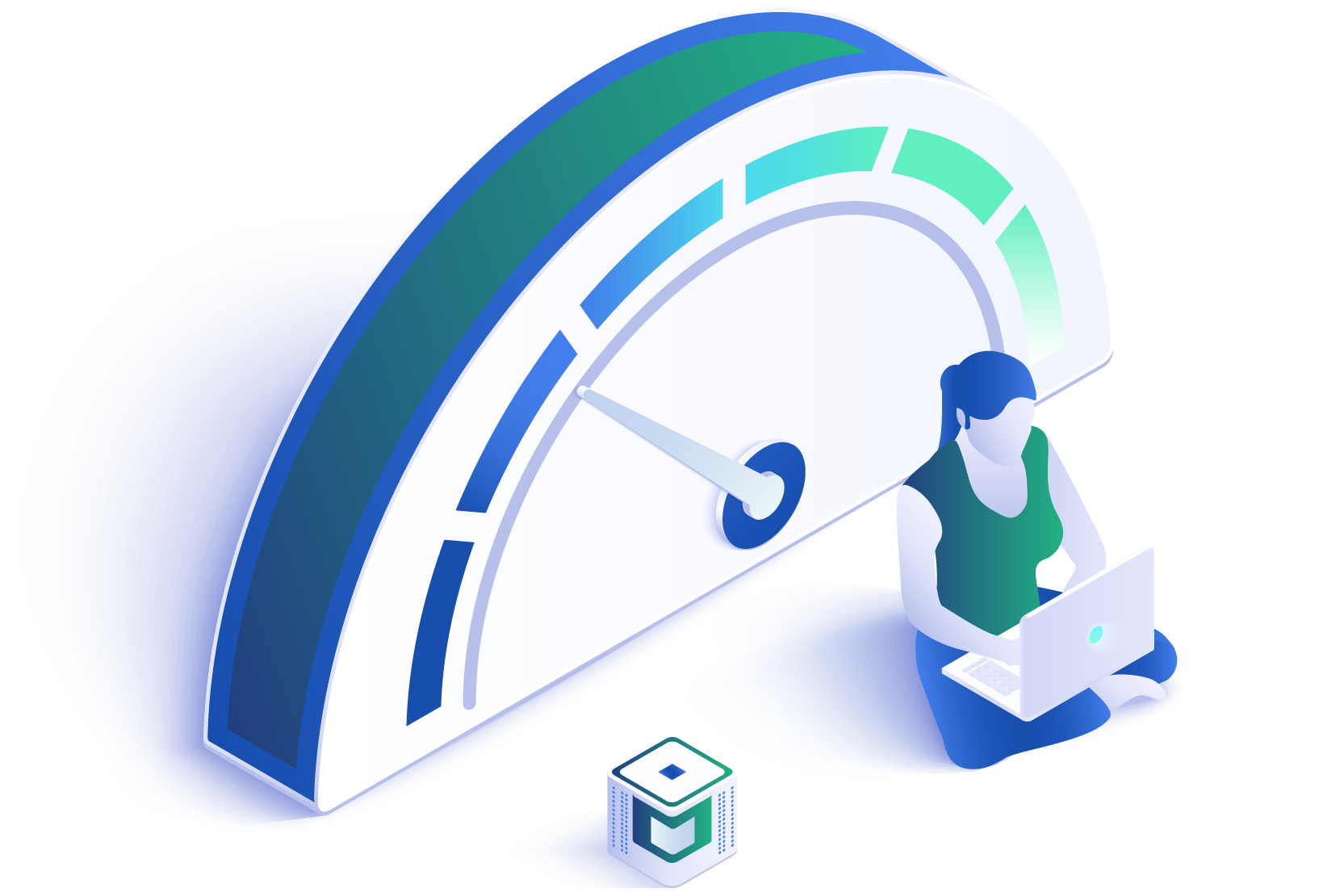
Technologies Used
Amazon Rekognition
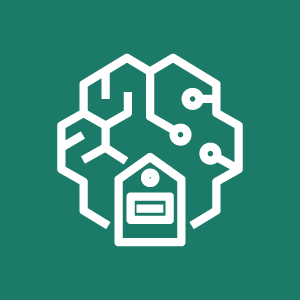
Amazon SageMaker
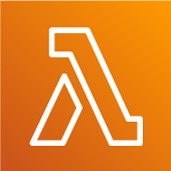
AWS Lambda
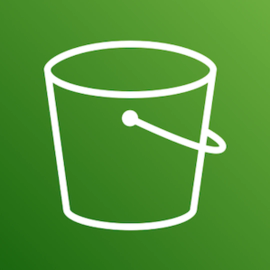
Amazon S3
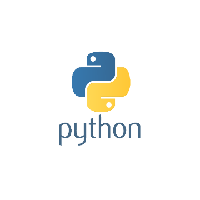
Python
Identify brand, style, print, size, and material across different fashion using Image Classification model
Solution
Quantiphi developed a machine learning-based Image Classification Model that was trained on a dataset containing thousands of images on the AWS cloud stack. The solution identified the style, size, print, and material across ten different products of a brand.
Our team also presented a comparison between Rekognition and SageMaker in terms of accuracy, cost, time taken, and parallelism for the client’s use case.
Results
- Reduced turnaround time for sorting products from days to a few hours.
- Enhanced product identification accuracy.