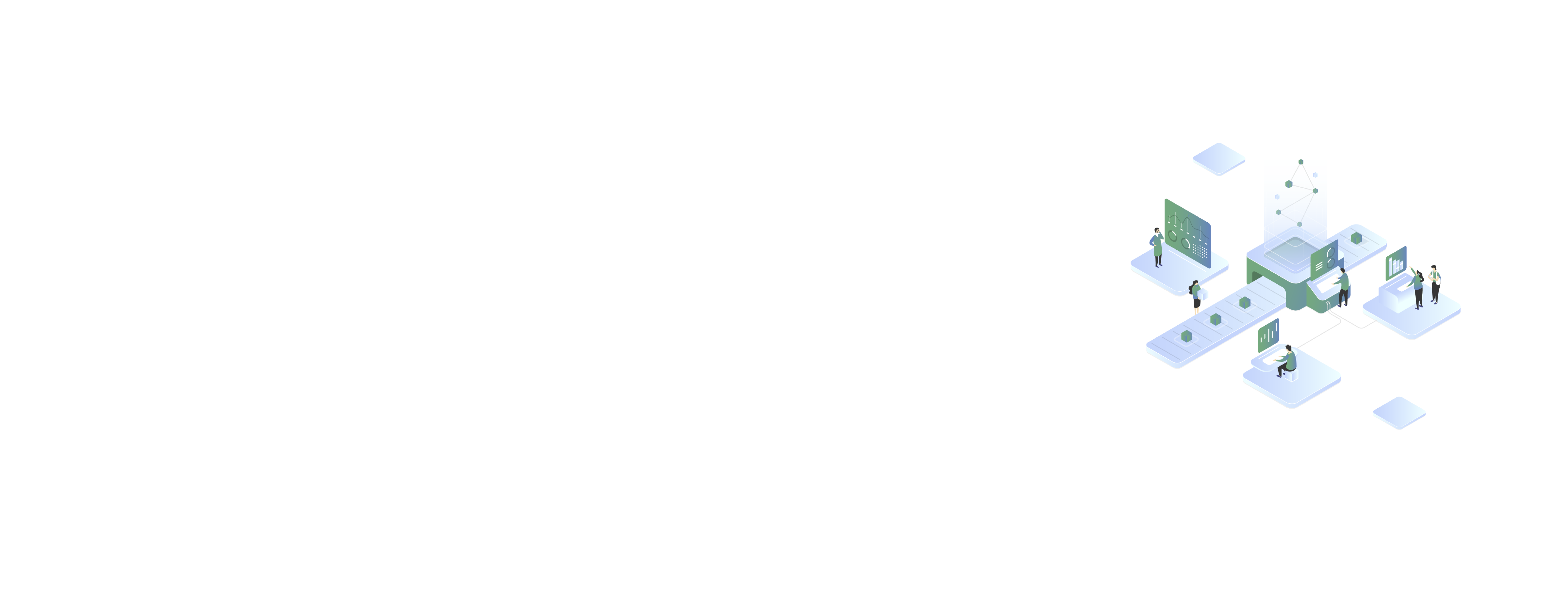
Click Detection
ManufacturingBusiness Impacts
Reduction of time in quality check
99%
Model accuracy
Better product quality
Customer Key Facts
- Location : North America
- Industry : Automotive
Problem Context
When two interlocking parts of a car are successfully joined together in a factory, they typically make a satisfying “click” sound. In case of a misconnection, it becomes difficult to debug and correct the error, driving high rework and warranty costs.
The client, an American multinational automaker with plants across the globe, had to rely on their operators to manually check for the electrical connector clicks which is time intensive and can result in errors. Therefore, they wanted an automated and scalable audio classification solution which could detect the click sound automatically and operate well on a plant floor.
Challenges
- Variation in noise
- Changes in the volume of the click
- Real-time inference pipeline
- Presence of parallel stations
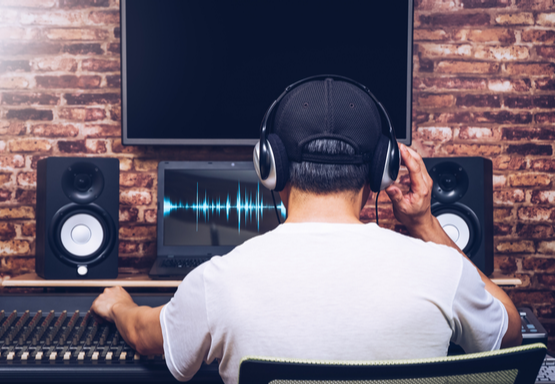
Technologies Used
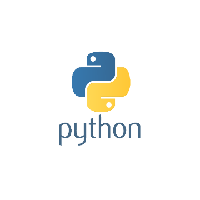
Python
Google Cloud Platform
AutoML
An Audio Classification Model to Detect Click Sound in a Noisy Environment
Solution
Quantiphi built a Convolution Neural Network (CNN) classification model to detect the click sound in real-time. The CNN-based architecture is capable of separating irrelevant background noise, distinguishing multiple target sounds, and identifying the exact time of the electrical connector click.
Result
- Quality Containment: 100% inspection of the connections, connected to plant databases, ensures that missed connections are caught and do not escape the plant
- Less Rework: Operators gain real-time feedback on connection quality, so that no connections are missed
- Improved Customer Experience: Better services results in customer satisfaction, thus building their trust towards the brand