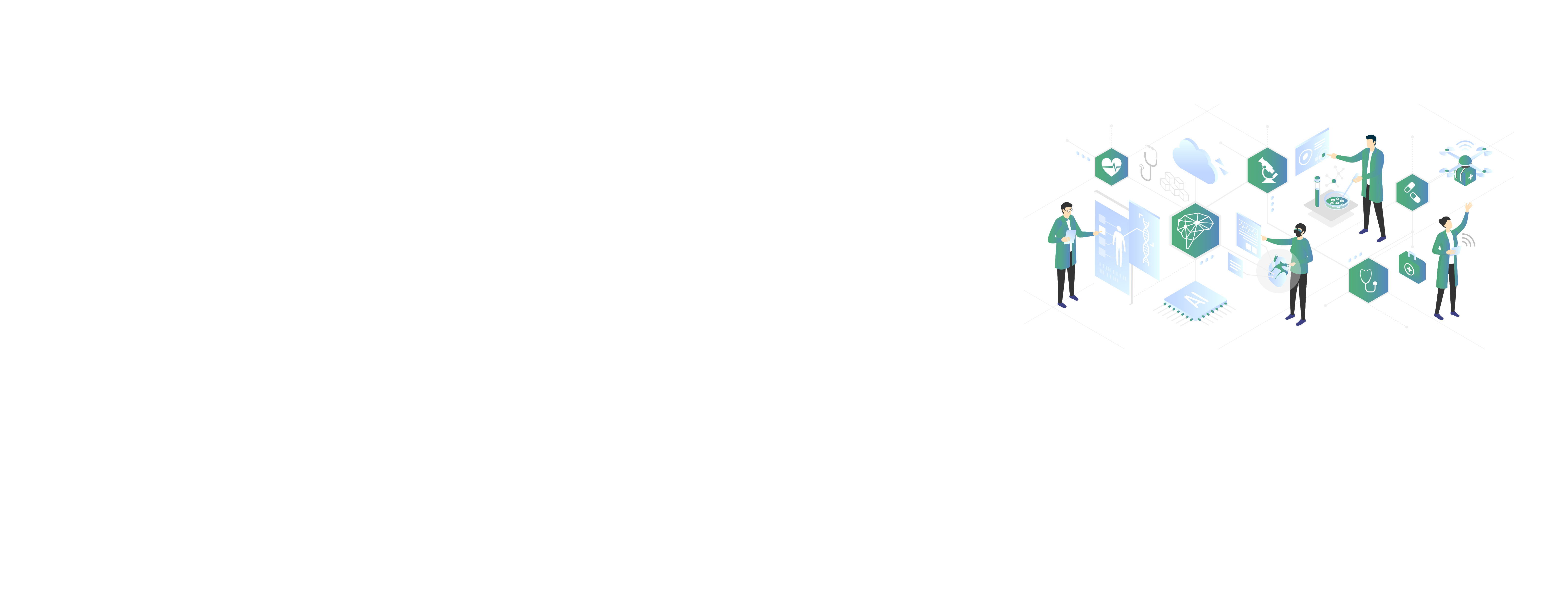
Disease Recurrence Prediction
Life SciencesBusiness Impacts
0.93 AUC
In tagging recurrence
30M+
Patient encounters processed and stored
Customer Key Facts
- Location : North America
- Industry : Healthcare
Problem Context
One of the main challenges in oncology is to track patientsโ treatment response and understand clinical and molecular features predicting treatment response.ย Crucial information is documented by physicians after analyzing a battery of tests. Another challenging component is using this information to understand the likelihood of cancer recurrence for the associated patient encounter.
Challenges
- The EHR notes from clinical, pathology, and radiology encounters contain useful information to predict subsequent events that are associated with cancer, but are mixed with non-critical information
Technologies Used
Google Cloud Platform
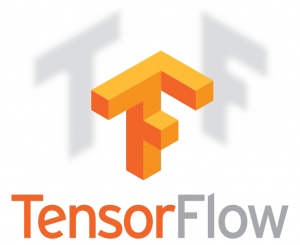
TensorFlow
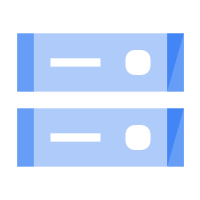
Cloud Storage

Cloud Dataproc
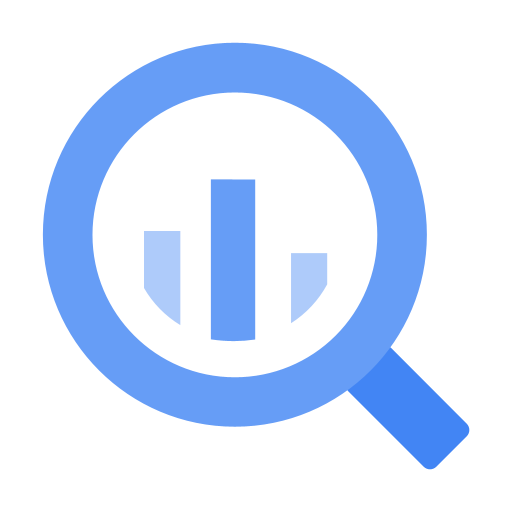
Google's BigQuery
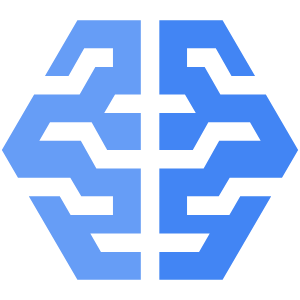
Cloud AI Platform
Natural Language Processing Approaches for Early Detection of Cancer Recurrence in Oncology Patients
The customer, one of the largest healthcare providers in the United States, wanted an efficient solution to flag cancer recurrence as it occurs in oncology patients. To improve their oncology health care, it was necessary that clinical identification be tailored to patients' unique treatment profile and expected trajectory.
Solution
Quantiphi created a predictive classification model capable of tagging patient encounters as related or unrelated to cancer recurrence for oncology patients. Included in this model were both structured and unstructured Electronic Health Record (EHR) data such as the patients' medical profiles, physician notes, pathology reports, lab reports, etc. to flag the patient feature associated with tagging the encounter as a recurrence.
A scalable data aggregation and augmentation pipeline was developed on Google's BigQuery and Dataproc, as well as a patient timeline to represent when features of the dataset occurred and the time of occurrence relative to cancer recurrence. Finally, Natural Language Processing techniques were incorporated, such as keyword search and TF-IDF, to structure the text data for use within the predictive model.
Result
- Aggregates data to establish a representation of patient history and flag recurrence as it occurs
- Automated tagging of patient encounters for cancer registry abstraction
- Helps physicians deliver life-saving assistance and targeted treatment to high-risk cancer patients