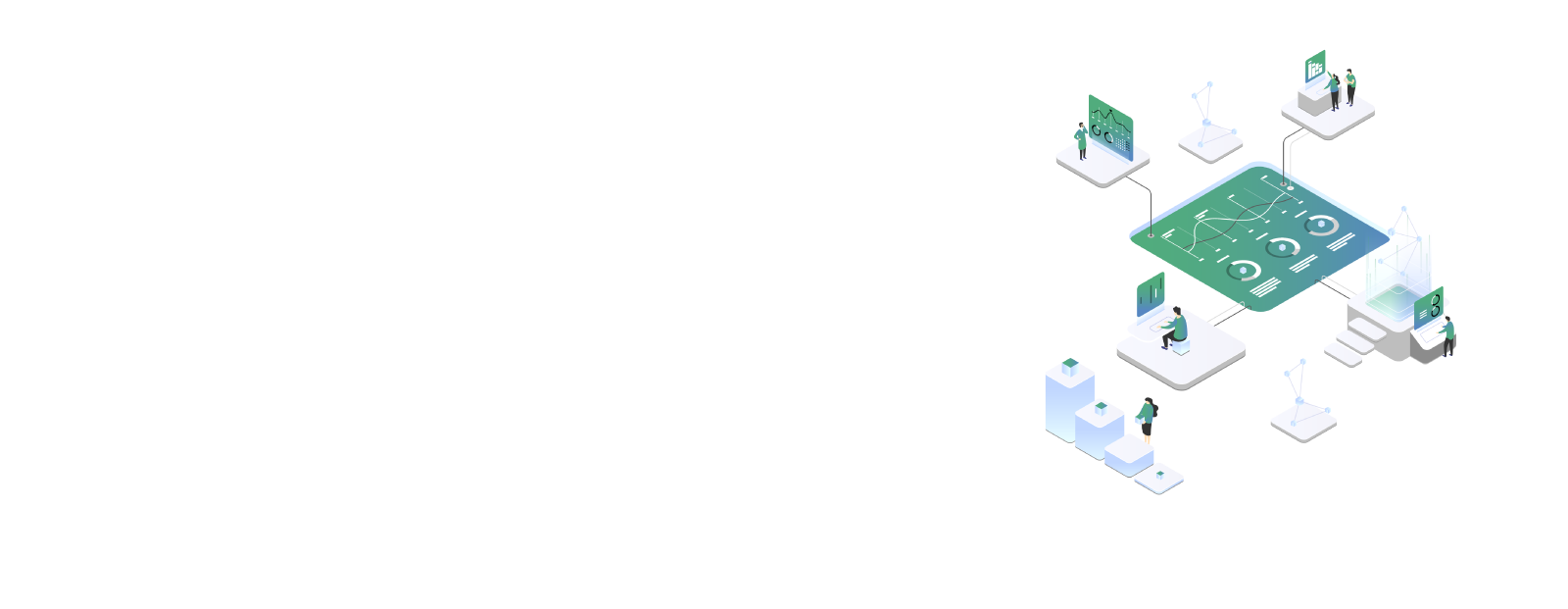
Enterprise Forecasting Engine
QSRBusiness Impacts
20%
Improved Accuracy Rate
25%
Reduction in food wastage
4.5
Million Savings per month
Accurate sizing inventory orders for restaurants
Client Key Facts
- Location : USA
- Industry : QSR
Problem Context
The client is a US-based quick-service restaurant chain with over 2.2k stores and is amongst the top 5 US food chains.
The client had a legacy forecasting engine that was inaccurate and not flexible enough to account for external features like holidays and other marketing variables.
The client wanted a forecast algorithm for every store in the restaurant chain for dollar sales, transactions, menu items, and ingredients at daily and 15 mins level. The solution had to be completely automated on AWS.
Challenges
- Finding an exhaustible set of features that affect demand trends, for building the model like holidays, weather conditions, etc.
- Developing a flexible, parameterized ETL process with no single point of failure which makes it easy to add functionalities in the future.
- Large scale data transformations with time and cost constraints for over 2000 locations and 500 SKUs.
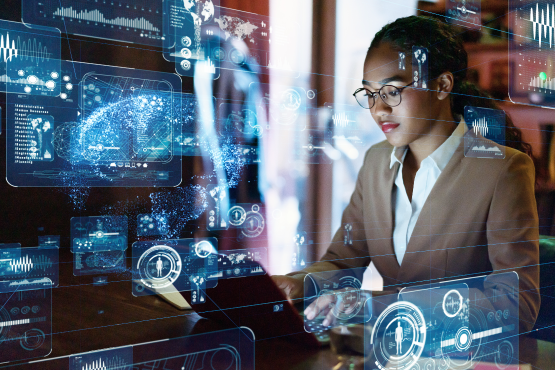
Tools & Methods
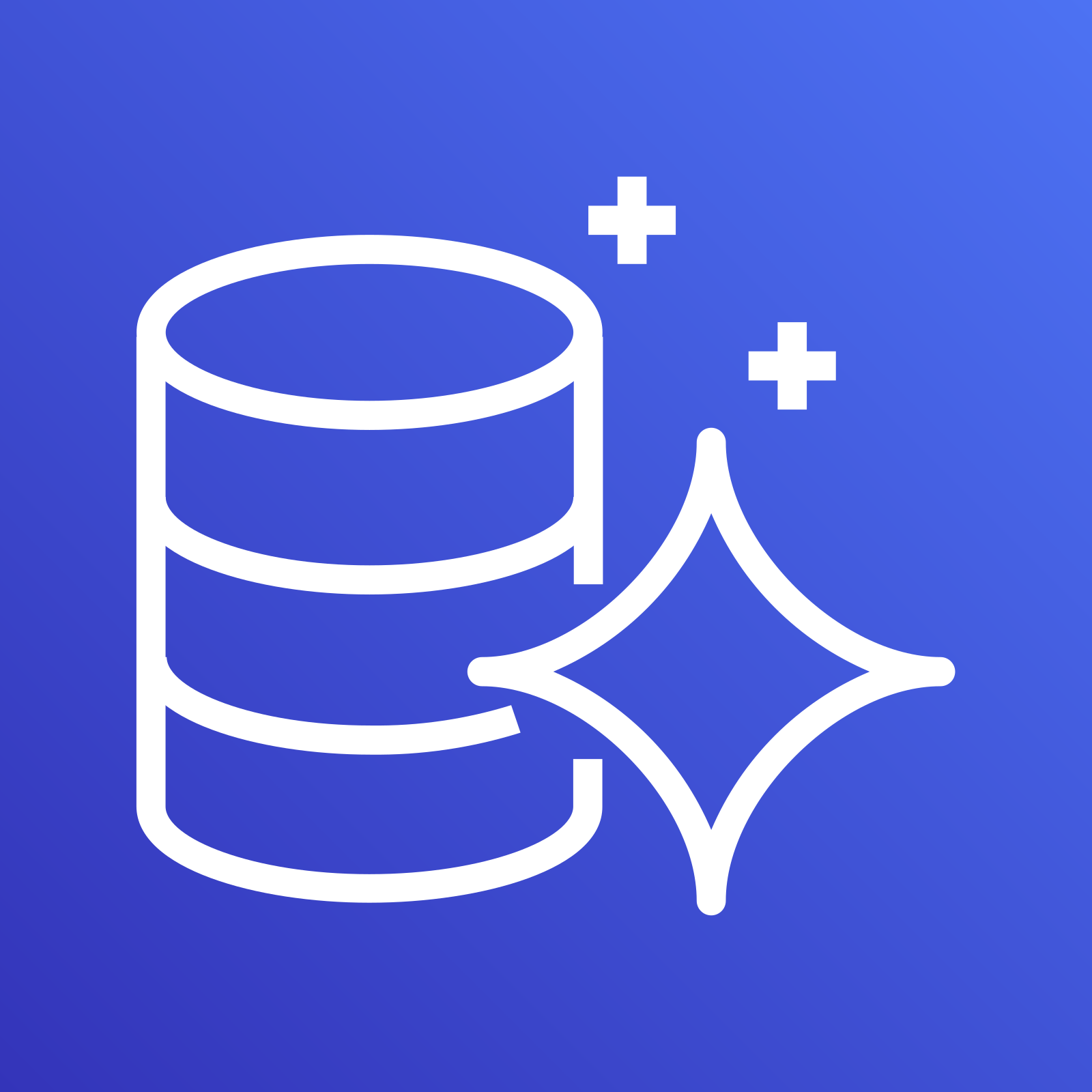
Amazon Aurora
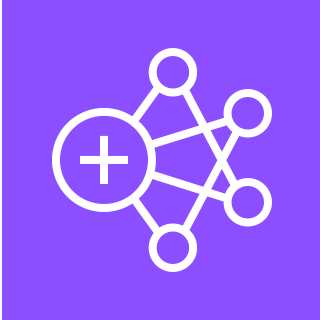
Amazon EMR
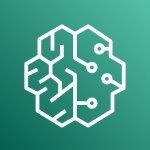
Amazon SageMaker
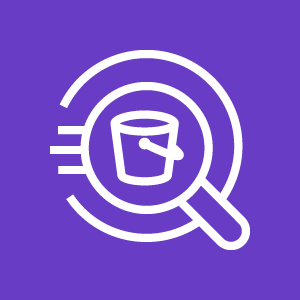
Amazon Athena
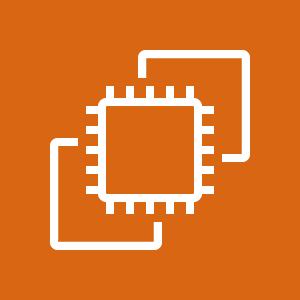
Amazon EC2
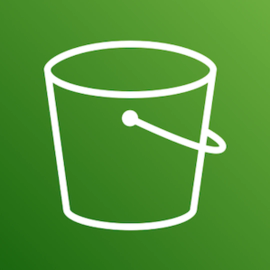
Amazon S3
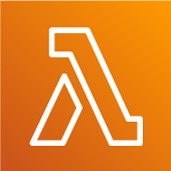
Amazon Lambda
Solution
Developed a fully automated and scalable deep learning-based forecasting engine to handle the large data and account for various business and marketing features.
The forecasting engine generated forecast for sales, number of transactions, products, and ingredients at daily and 15 mins level for 2.2k stores, 500 products, and 400 ingredients.
The solution further consists of an automated alert mechanism to send notifications on client communication channels based on custom conditions.
Result
- Successfully developed a forecasting engine to forecast demand several weeks in advance to cater to various business requirements by leveraging Amazon EMR.