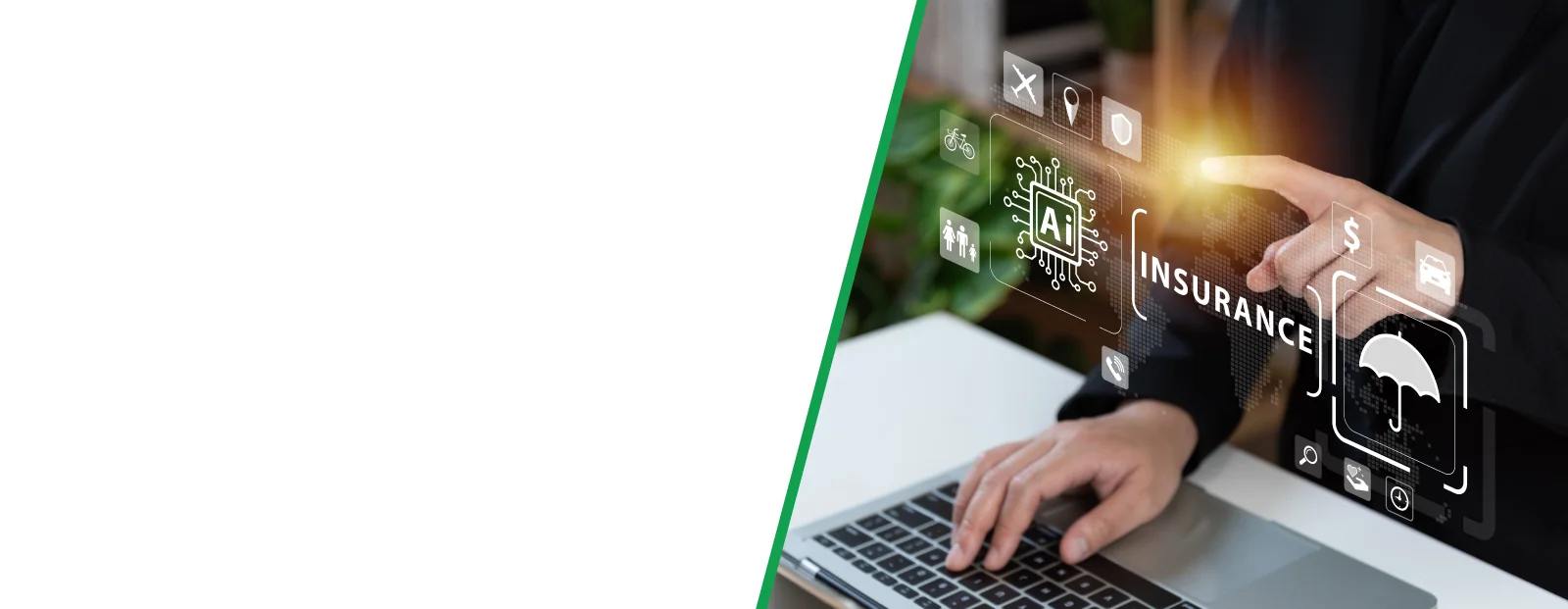
Enabling R&D by efficiently operationalizing ML models
InsuranceBusiness Impacts
6x
Increase in speed of end-to-end cycle
5 Million
Predictions generated per month
~75%
Time saved for model deployment
~83%
Time saved for Ingestion
Customer Key Facts
- Country : America
- Size : 10000+
- Industry : Insurance
- About : Client, an American insurance company, is one of the largest provider of supplemental insurance in the US providing financial protection to more than 50 million people worldwide.
Problem ContextÂ
The client had all its data on-prem. All their scheduled jobs and computation were performed on virtual machines. Client required a machine learning framework that includes data preparation, preprocessing, post-processing operations, one-click Automated ML model training and inference pipelines and an interactive dashboard to view ML model results, and insights.
Challenges
- Getting requirements for future development is a challenge as technology stack for future models is not yet defined
- Integrating real time data with CI/CD pipeline with proper security for inferencing
- The data might include the processing of PII and PHI data, thus no visibility on data for all the models
- No clearly defined requirements for consumption
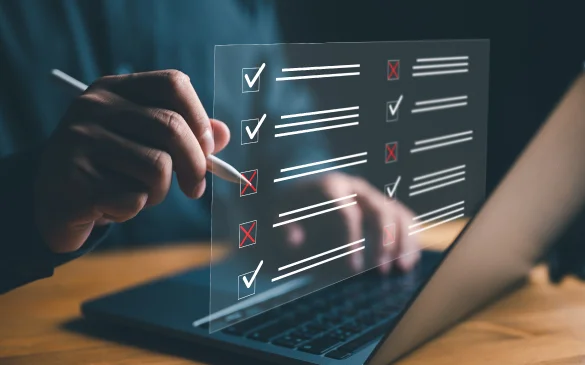
Technologies Used
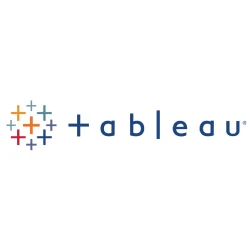
Tablaue
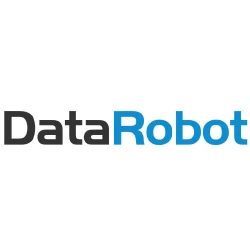
DataRobot
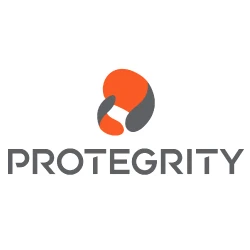
Protegrity
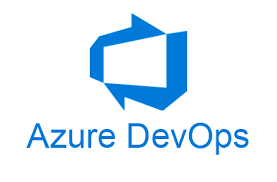
Azure DevOps
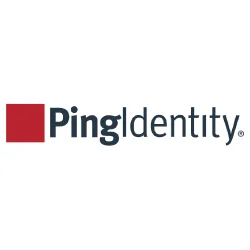
Ping Identity
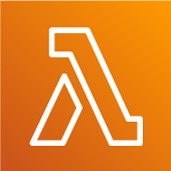
Lambda
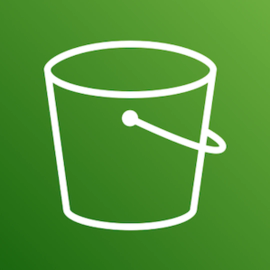
S3
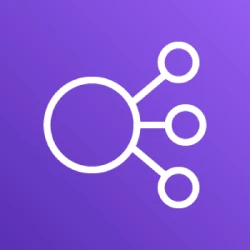
Elastic Load Balancing
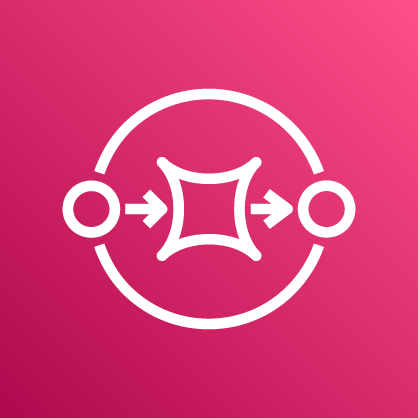
Amazon SQS
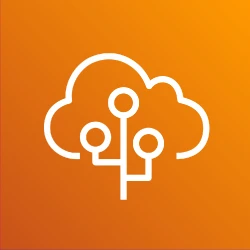
AWS Elastic Beanstalk
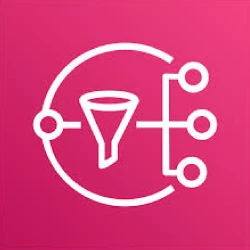
SNS
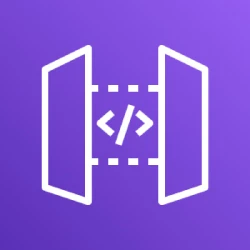
API Gateway
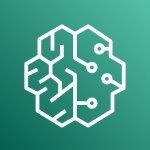
Sagemaker
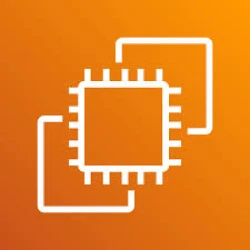
EC2
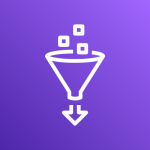
Glue
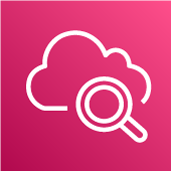
Cloud Watch
Solution
Quantiphi developed an automated ML framework, which include
- Data collection from various on-prem sources and ingest them to cloud databases
- Running scheduled jobs to load data in AWS cloud environment
- Automated training, inference pipelines and model management
- Storage of model outputs stored in a cloud database
- Democratization of Model outputs via API to all teams
Results
Enhanced Customer experience
- Significant improvements in the speed and accuracy of predictions.
- Increased customer satisfaction due to quicker response times and personalized insurance offerings.
High Reach Distribution System
- Model outputs democratized via APIs, making data accessible to all teams.
- Improved decision-making and collaboration across departments.
Creating Operational Efficiency
- Reduction in end-to-end cycle time significantly reduced
- Drastic reduction in model deployment time
Helping various departments by R&D for their KPIs
- Customer support team enabled to control Customer churn
- Sales Team enabled by having reasonable targets
- Added a recommendation engine to suggest top policy pick personalized for a customer
- Analyze Performance of different departments
- Calculating Bonus for Agents on sales