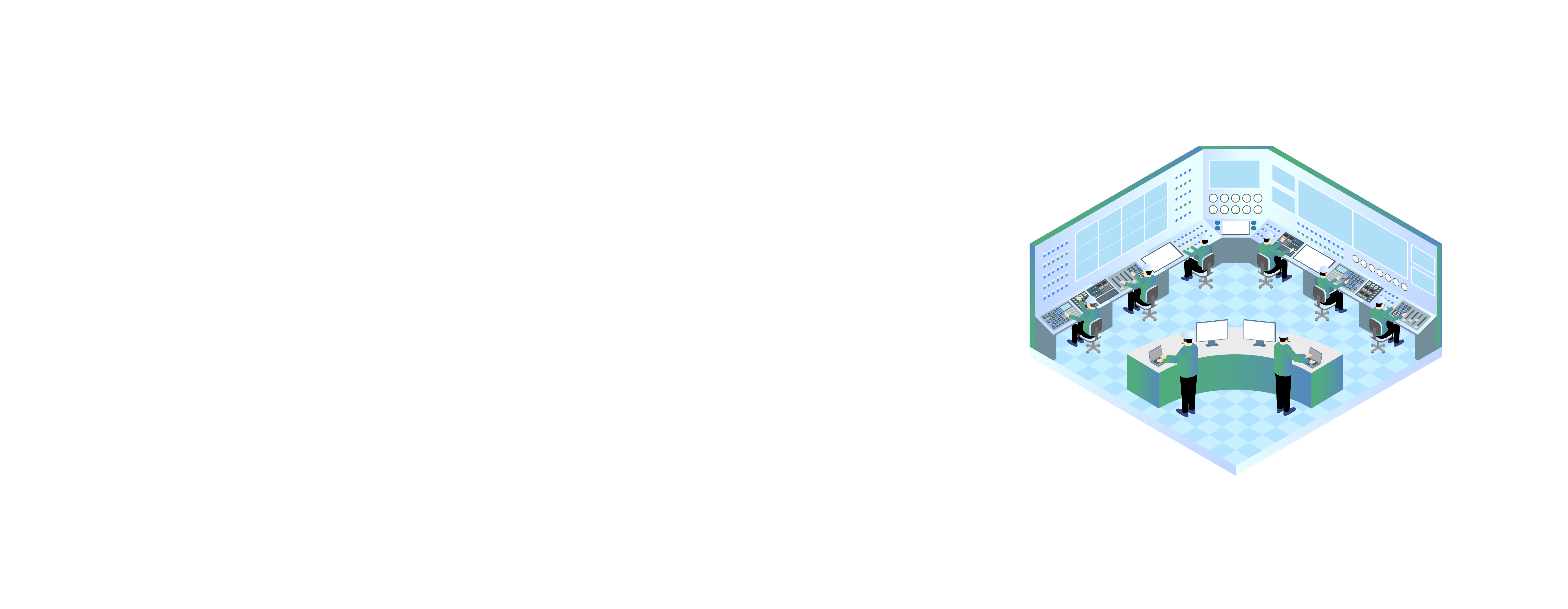
Intelligent Engineering Document Compliance
Energy & UtilitiesBusiness Impacts
30+
Million pages processed
97%
Solution accuracy
3 - 5
Years of manual effort saved
Customer Key Facts
- Location : North America
- Industry : Oil & Gas
Problem Context
The customer required a large number of engineering documents to be stamped and authenticated by a dedicated team of engineers for verification and audit purposes. However, identifying the stamped documents, which range from size and number of pages, was a manual, unstructured, and time-consuming process.
Furthermore, the stamps are not consistently placed, sized, clearly visible, or have the same signatures of the engineers. Therefore, the customer wanted an automated solution that could reduce the manual efforts involved in searching for stamps and classifying documents.
Challenges
- Stamps in diagrams are not consistently placed, sized, or have the same signatures of the engineers
- Stamps are sometimes faded or blurry
- Documents can be large in size and number, and may contain multiple of pages
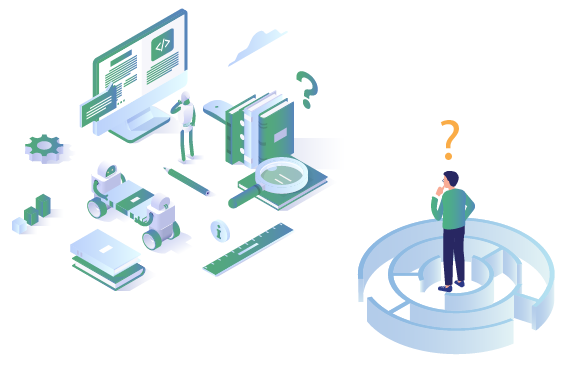
Technologies Used
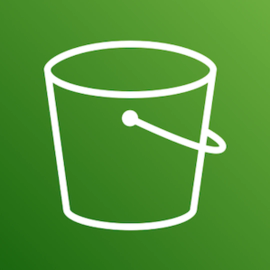
Amazon S3
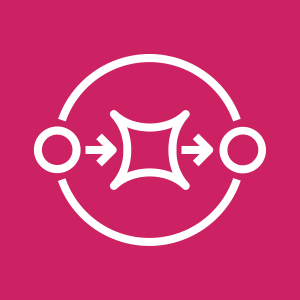
Amazon SQS
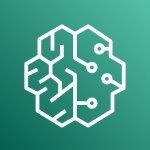
Amazon SageMaker
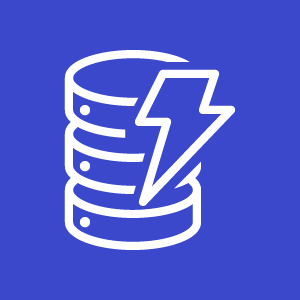
Amazon DynamoDB
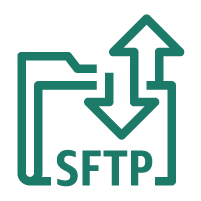
AWS SFTP
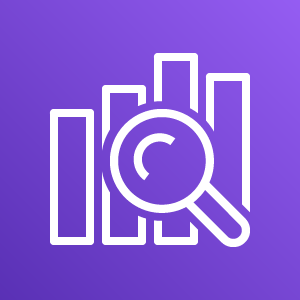
Amazon Elasticsearch
Automating the Detection of Stamps Across Large Volumes of Engineering Documents to Fast-Track Verification & Audit Processes
Solution
Quantiphi leveraged machine learning to develop a solution that helps accurately detect the presence of stamps in engineering documents and store them along with the file name for easy access, audit, and verification purposes.
Result
- Reduced manual efforts
- Streamlined process
- Documents are now structured with stamp information that is easily accessible and searchable