Key Takeaways
- Machine Learning algorithms are frequently characterized as “black boxes,” making it nearly impossible to eliminate bias and inequity.
- Often, AI explainability is compromised in favor of accuracy, however companies can make their AI models explainable and transparent by employing appropriate tools.
- AI model biases can be identified and resolved with the use of explainability
- Businesses can no longer overlook the importance of incorporating transparency. This is crucial not only for fostering user acceptance but also for adhering to legal compliance requirements
Imagine a scenario where AI makes impactful decisions - decisions that influence healthcare, justice, and our everyday lives. As AI advances, it's not enough for these decisions to be accurate – they must also be understandable, justifiable, and accountable. Unfortunately, the entire computation process has transformed into a “black box" and has become difficult to interpret how the AI algorithm arrived at a particular result. In the ever-evolving landscape of artificial intelligence, this is where the concepts of explainable AI and AI transparency take center stage.
Beyond the technical jargon, we'll explore why explainability and transparency in AI are not just industry buzzwords, but the cornerstones of building humane, ethical, and trustworthy AI systems. Let's dive deep into their significance, understand how they impact various sectors and uncover the techniques that propel us toward a future where AI decisions are not shrouded in mystery, but illuminated by clarity.
Why Are Explainable AI and AI Transparency Vital?
In the complex world of AI, explainability refers to the ability of AI systems to provide understandable explanations for their decisions. This is crucial, especially in fields like healthcare and criminal justice, where AI can profoundly impact lives. The more complex AI models become, the harder it is for humans to grasp their inner workings. Transparency, on the other hand, involves making the data, algorithms and models accessible. Together, explainability and transparency build trust and accountability in AI.
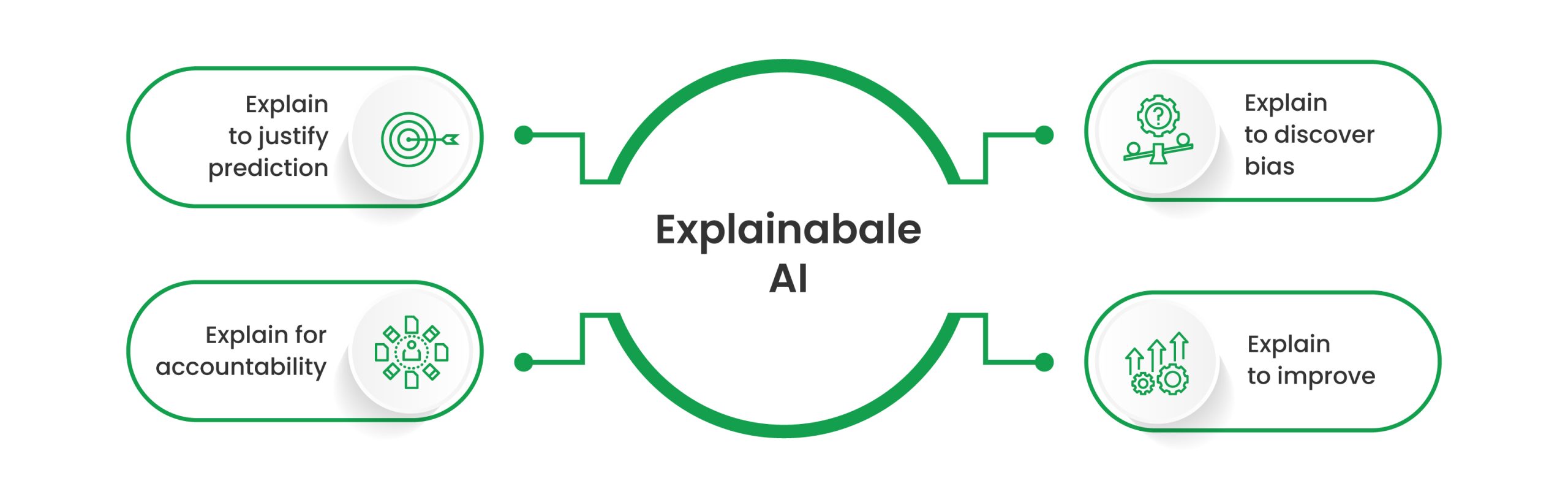
For instance, in self-driving cars transparency ensures we can predict how AI will react in challenging situations allowing us to enhance safety. It can also help pinpoint biases, enabling us to correct them and improve accuracy. Moreover, explainability can lead to valuable insights, driving advancements in AI algorithms.
Explainable AI - Key Elements
To achieve effective explainability, several criteria must be considered:
- Coherence with Prior Knowledge: Explanations should align with what users expect.
Consider this example: Imagine your job application has been denied. With a good explanation, you would already be aware that your educational background and major were taken into account if your job application was not moved forward. It essentially avoids introduction of new criteria or features that the user was not anticipating. - Fidelity: Explanations should provide the complete narrative and justification, avoiding misleading information.
For example, In medical diagnosis, the model for identifying skin cancer could provide not just a label ("malignant" or "benign") but also an explanation of the features that led to that decision, such as irregular borders or color variations in a skin mole. This way, doctors and patients can understand the rationale behind the diagnosis, increasing trust in the AI system and enabling better-informed decisions. - Abstraction: The level of technical detail in explanations should match user preferences.
Consider the case of a medical image classifier diagnosing cancer. Some users might want to know the precise critical features that caused the diagnosis, while others may prefer a more generic explanation. - Abnormalities: Explanations should clarify unusual outcomes.
Imagine a scenario where the AI model detects cancer in an image, but the doctor can't see anything unusual. In this case, the model should explain the reasons behind its decision, even if they seem abnormal. - Social Aspect: Tailor explanations to the audience's knowledge and terminology.
In the case of cancer categorization, the explanation provided to the patient and the clinician would differ significantly. The end user may not understand medical terms, while the doctor is familiar with precise medical terminology. - Unbiasedness: Ensure explanations remain impartial.
Explanations should not differ based on a person's gender, color, or other characteristics. - Privacy: Protect sensitive data in explanations.
Data privacy should be enforced and sensitive data should not be revealed in explanations. - Actionability: Advise users on what to do.
In application use cases like loan approvals, actionability is crucial. Explanations should guide users on what changes they can make to qualify.
Choosing the appropriate criteria depends on the use case. For example, actionability is vital in loan applications, while data privacy is crucial in healthcare.
Expert Speak: Built Trust by Successfully Implementing Responsible AI Practices in Your Workflows and Processes
Recipients in the Explainable AI Ecosystem
Different people and audiences require different explanations:
- Internal Users:
Employees who use AI systems for decision-making need quick, efficient explanations. In a loan extension application, the employees who use the software and approve/deny loans may want to provide quick answers to multiple customers without wasting time. - End Users:
Customers applying for loans or similar services need explanations tailored to their situation. In the same application, the customer who applies for a loan and gets denied will want to know what they need to do or change to be approved. - External Observers:
Regulators and executives may require insights into how AI applications work, ensuring fairness and consistency. In the same application, these would be regulators who want to verify how the application works or executives who may want to know that the criteria for approving/denying loans don’t change across people, locations and time windows.
Transparent AI- Key elements
Transparency involves three key elements:
Data Used:
Describe the data used to train the model. For instance, you may include, “Our model was trained with data on US applicants without financial anomalies.
Model and Inferences:
Explain the model's confidence levels and inferences. You may include, “Our model has a 95% confidence level for US applicants and 80% for worldwide candidates.”
Challenges:
Acknowledge that transparency isn't always desirable, as it can be misused for manipulation or provide a competitive edge to rivals.
AI Explainability Vs. Accuracy
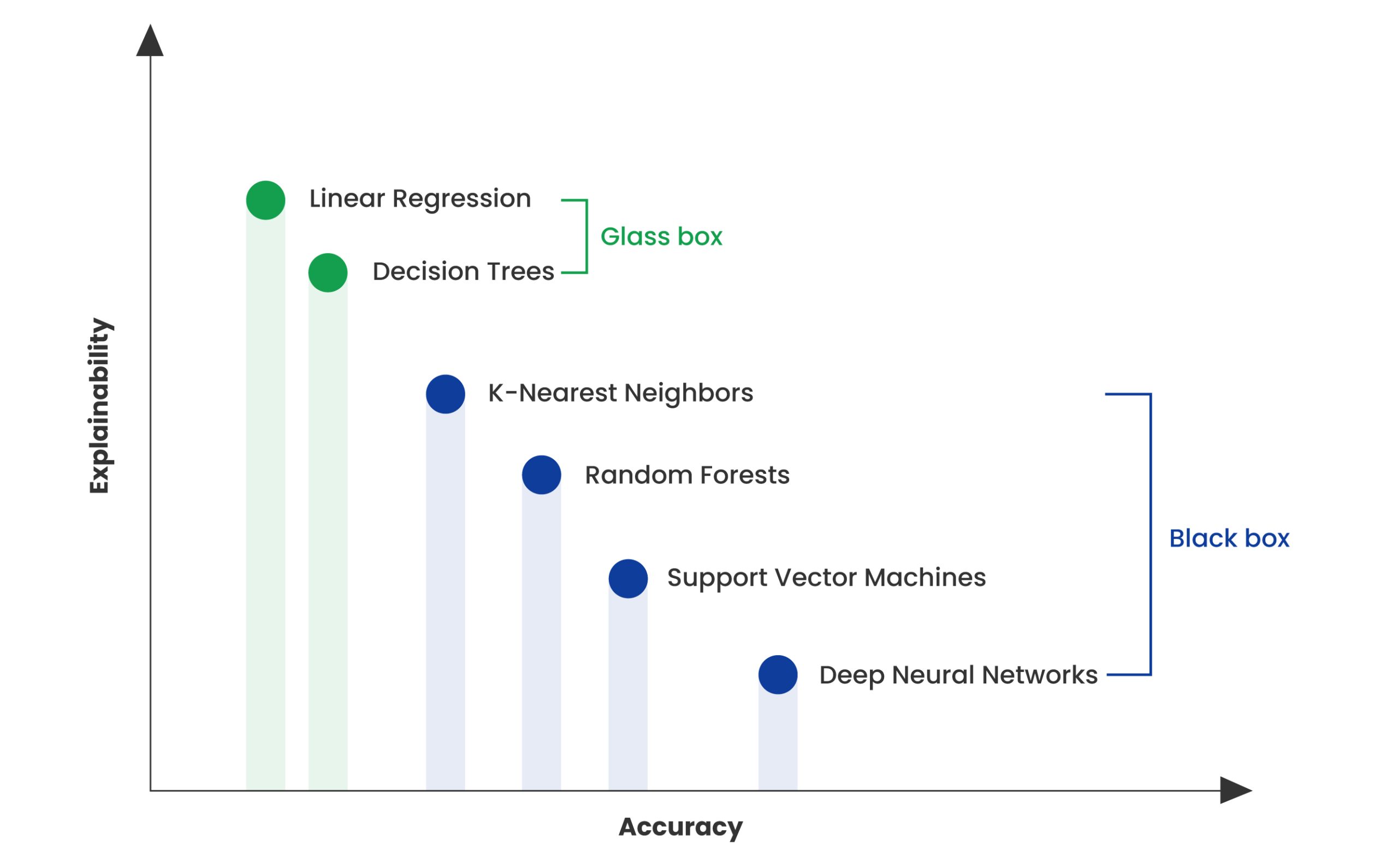
Balancing explainability and accuracy is essential. It depends on the use case, as some situations prioritize accuracy over explainability, while others require the opposite. The choice between a "black box" model and a "glass box" model depends on these considerations.
Methods for Explaining AI Models
Several techniques can be used to achieve explainability:
- Model-Agnostic Techniques:
These techniques require only the input and output of the model to explain its behavior. Examples include LIME, SHAP, and Counterfactual Analysis. - Model-Specific Techniques:
These techniques need access to the model itself, including its weights and gradients. Examples include LRP (Layer-Wise Relevance Propagation) and Integrated Gradients.ients. Examples include LRP (Layer-Wise Relevance Propagation) and Integrated Gradients.
These methods can be applied globally (explaining the entire model) or locally (explaining individual predictions). It can be difficult to achieve explainability and transparency in AI, especially in complex systems with deep neural networks. However, there are several approaches that can be helpful:
- Interpretable models: Models intended to be more transparent and understandable are known as interpretable models, and using them is one strategy. These models can offer more comprehensible explanations because they are often simpler than deep neural networks.
- Post-hoc explanations: Another approach is to use post-hoc explanations, which are explanations generated after the model has made a decision. These explanations can help provide insights into how the model arrived at its conclusion.
- Transparency frameworks: Transparency frameworks can aid in ensuring the openness and accessibility of the data, methods and models utilized by AI systems. These frameworks can include open-source software, data-sharing policies, and model documentation.
- Human-in-the-loop: Incorporating people into the decision-making process is another strategy, either as part of the model training process or as part of the decision-making process itself. This can help ensure that the decisions made by the AI system are understandable and align with human values.
The Path to Ethical and Trustworthy AI
In this blog, we navigated the intricate landscape of AI, shedding light on the critical concepts of explainability and transparency. These pillars are not mere buzzwords but are essential for fostering trust, accountability, and ethical AI practices.The journey toward achieving explainability and transparency in AI can be challenging, especially in complex systems, like deep neural networks. However, as AI continues to integrate into our lives, it's imperative that we persist in our efforts to establish these principles. By doing so, we ensure that AI systems operate in ways that are not only trustworthy but also aligned with human values.
Embrace the power of explainability and transparency in AI. Learn how to make your AI systems more understandable and trustworthy. Join the journey towards ethical and accountable AI today!