Leveraging AI and ML to refine customer engagement in insurance by improving customer experience throughout the life cycle.
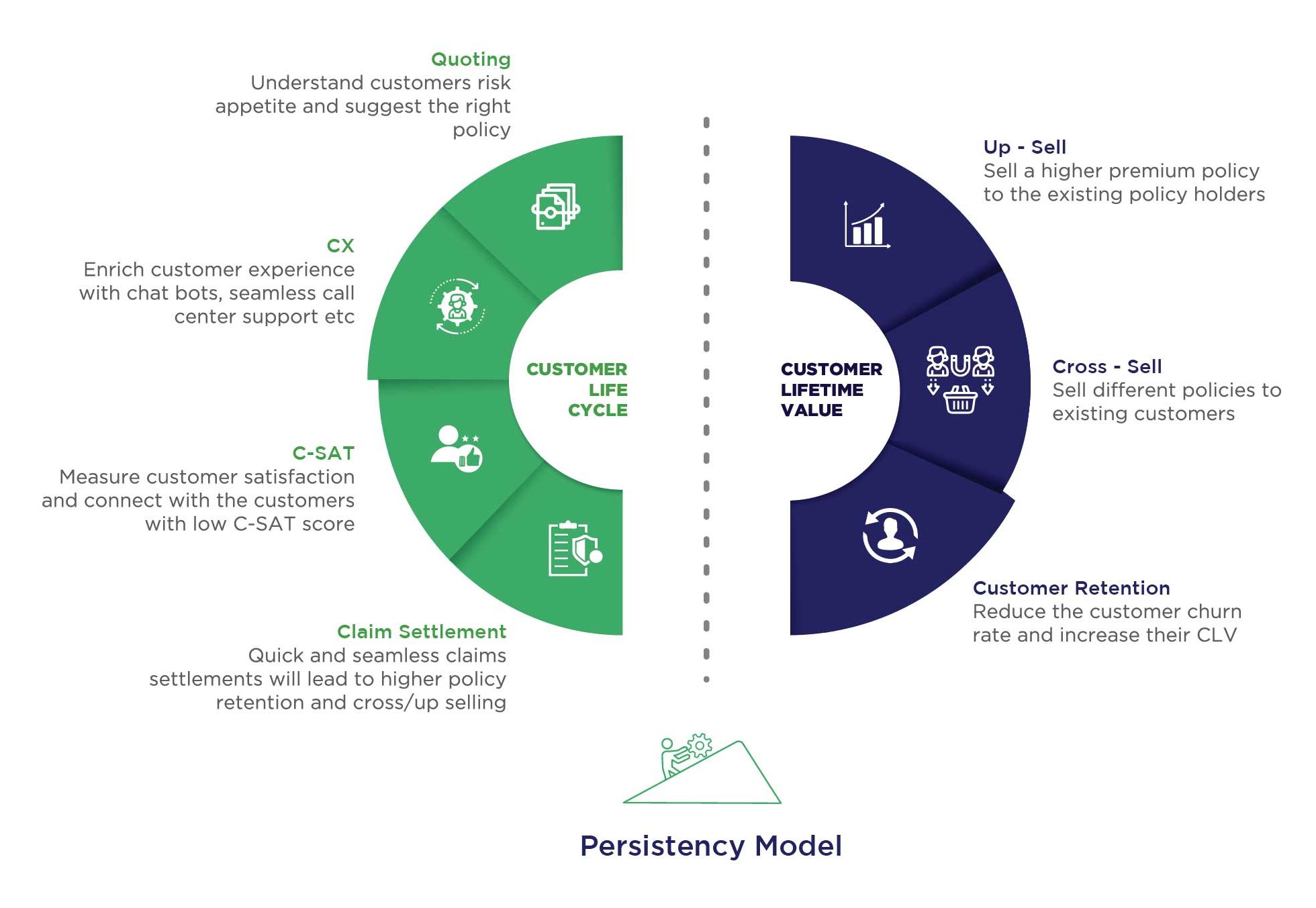
Italian economist Vilfredo Pareto’s 80-20 rule is an aphorism that asserts that 80% of outcomes result from 20% of all causes for any given event. It is true in sectors such as insurance where 80% of the company’s business comes from 20% customers, making it imperative to identify these 20% of customers and maintain good relationships with them to ensure future revenue.
However, maintaining relationships require companies to identify the key pivotal points in a customer’s journey and implement end-to-end solutions to increase customer value and retention. Retaining customers even in this era of digital is critical for insurers as:
- Acquisition of a new customer can cost five folds more than retaining an existing customer
- Satisfied customers bring in more customers
- Reduced retention rates negatively impact the reputation of the company
In addition, the number of “at-risk” insured is rising at an alarming rate in different verticals. According to a study, the percentage of customers who will “definitely renew” with their incumbent carrier has dropped significantly. The rate of switching among insurance shoppers has increased, driving down customer retention. The decreased customer retention can be attributed to a crowded market, lack of multiple and effective customer service touchpoints, rising cost of insurance, and customers’ inquisitiveness about services.
The Evolution of Customers
New consumer habits can be attributed to technological evolution. With changing times, customers’ expectations from insurance products have rapidly evolved and compelled insurers to revamp their products and services. But the drastic shift in consumer behavior came with the increasing number of millennials in the market. More and more customers now prefer direct touchpoints with insurers. There is a growing demand to redefine the customer engagement journey for delivering better services.
Want to know how our intelligent AI-driven solutions can help you keep up with the changing customer needs? Explore our insurance solutions now!
Redefining Customer Engagement Journey
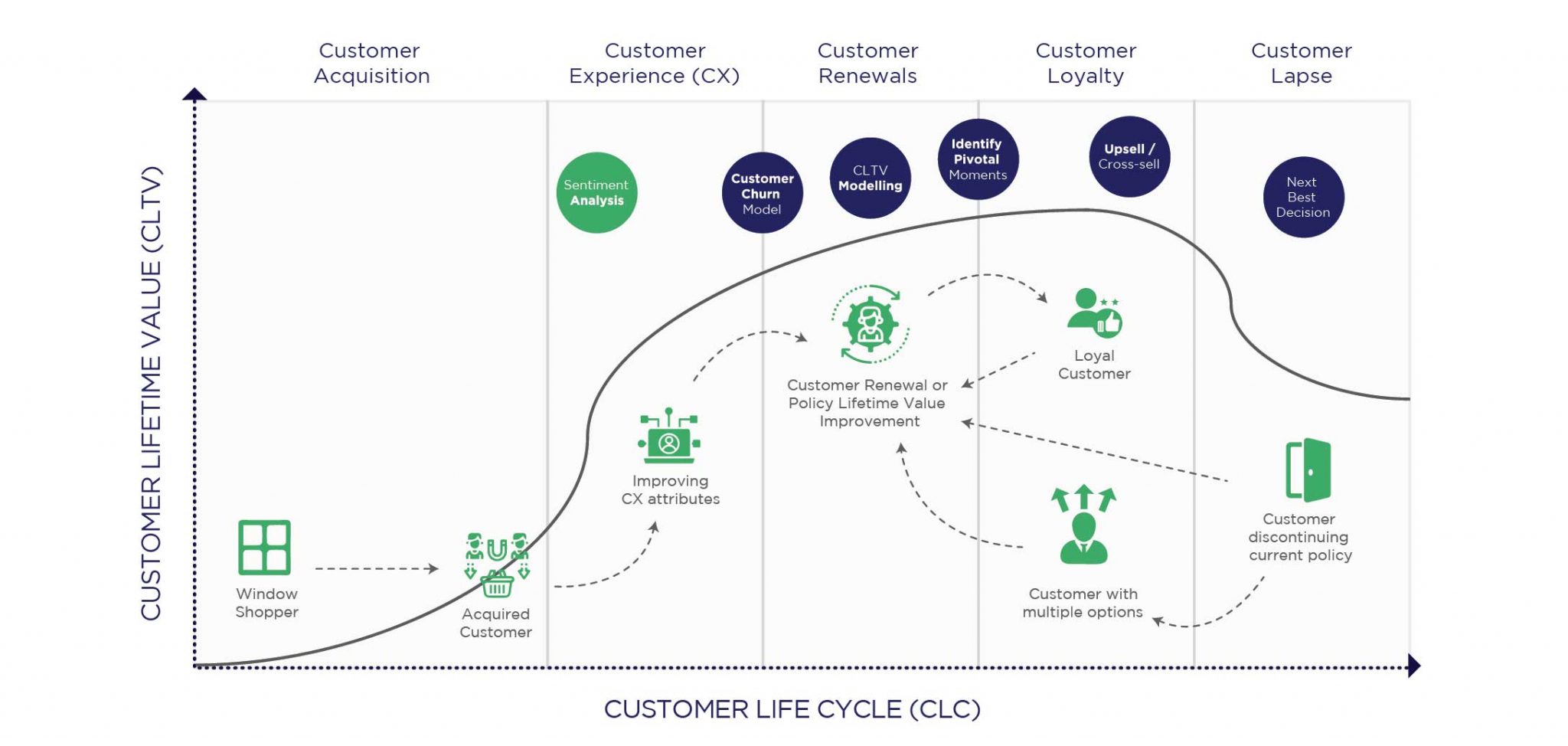
Understand – Engage – Retain – Renew
Understand:
By understanding customer’s sentiments – Insurers must analyze customer product reviews and feedback surveys to identify the subject of discussion with emotion polarity (positive, neutral, or negative) associated with it. Additionally, they should assess customer sentiments through vast amounts of data available to determine the C-SAT score, key promoters, and detractors for devising effective strategies.
By understanding customer churn probability – Companies must identify customer sentiments along with the historical data to determine the dynamic churn probability of customers. Predicting the probability of customer churn can help agents in narrowing down high-risk customers.
By understanding the value of customers – Once the risk level of customers is identified, insurers must devise strategies to prioritize customers. This can help them in figuring out high-value customers and targeting them. Understanding the current as well as the future value of customers can be helpful in identifying the right customers to engage with.
Engage:
After understanding the current and predicted future value of customers or the CLTV of customers, insurers can segment the population into 4 categories, namely:
- High Current High Future Value
- High Current Low Future Value
- Low Current High Future Value
- Low Current Low Future Value
This segmentation will help in identifying the next best actions for each set of customers.
Adding value – Cross-sell/Upsell:
Based on the segment of customers, insurers can either try cross-sell or upsell options to provide more value to their customers if they fall under high satisfaction and future value category. They can further dive deep into sentiments and churn drivers for a better understanding of concerns and challenges.
By identifying-What, Whom, When & How:
Through customer need analysis, customer preference analysis, and predicted revenue/profitability assisted with propensity modeling, agents can target policyholders with the highest propensity to purchase the appropriate products/services.
Q Solution for Persistency
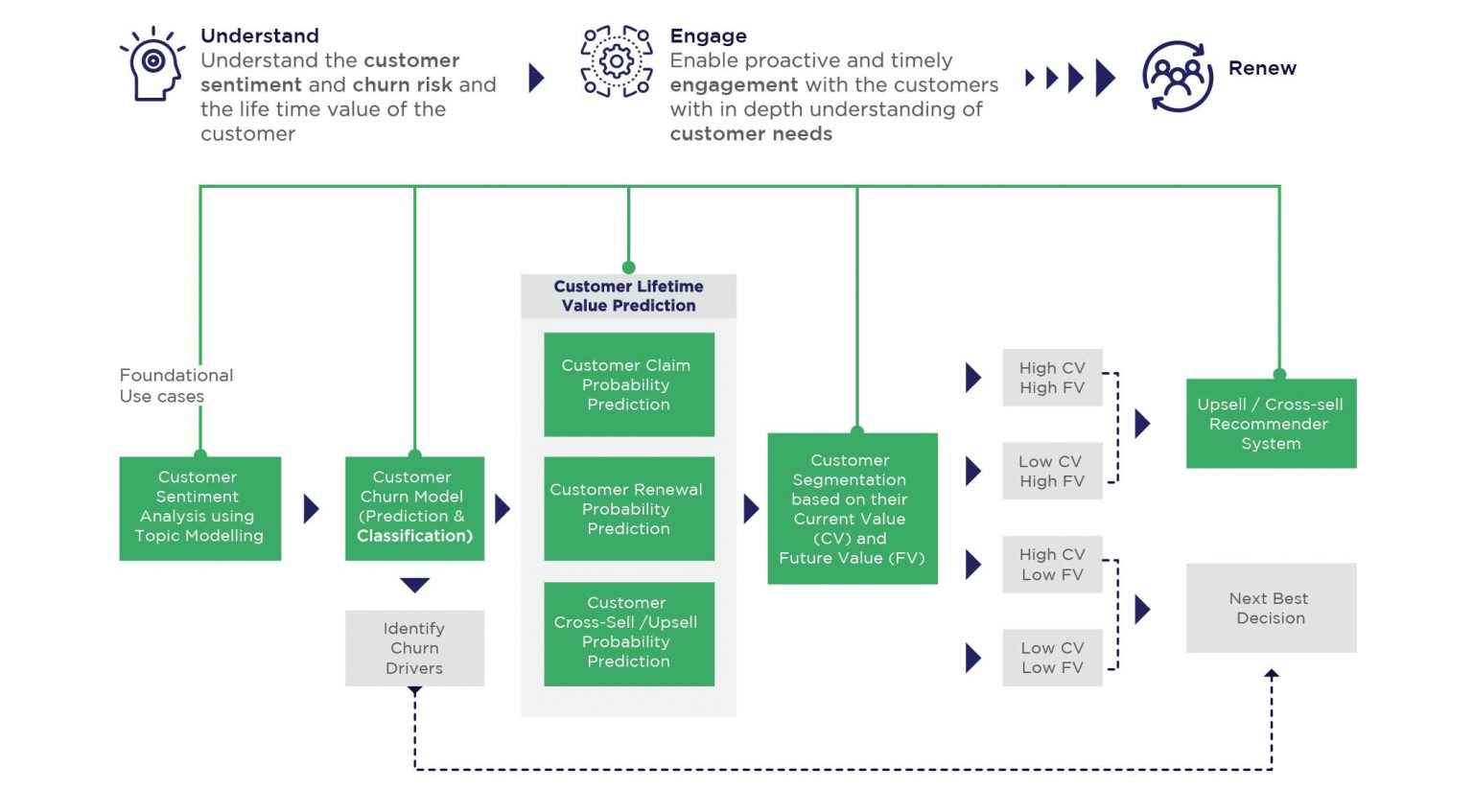
Quantiphi’s persistency solution is specifically designed to address the needs of insurers and agents. The platform identifies the customer satisfaction level through sentiment analysis by interpreting customers’ sentiments towards their products, brands, or services using online conversations and feedback surveys. It analyzes churn drivers and churn probability by leveraging the available data like demographics, transactions, call volumes, and more to help insurers better align their products/services according to the changing customer needs.
Not only this, with three independent ML models – Customer Claim Probability Prediction, Customer Renewal Probability Prediction, and Customer Cross-Sell/Upsell Probability Prediction, the platform determines the most accurate CLTV. CLTV prediction happens throughout the customer lifetime to incorporate all the changes in customer behavior, life events, environment events.
To help the agents target the most valuable customers, the solution provides a simple segmentation of the customer’s current and future value. This makes it easier to identify customers that contribute to the majority of revenue for the company.
Quantiphi’s AI-based platform, thus, refines the persistency approach and aids businesses to understand and engage with its customers while improving customer service and retention proactively across product lines.
Looking to increase your persistency rate and enhance your customer journey? Book a session with our experts now!