In the last few years, the data analytics field has gone through a mini revolution. Firms in industries as diverse as media, manufacturing, utilities, etc. have been shoring up their analytics departments, as they realize they are sitting on a potential gold mine of business insights, waiting to be captured from the huge volumes of data they are capturing. Analytics consultants and vendors have also been hiring at a brisk clip, as they try to capture the huge opportunity that has sprung up.
Whether analytics is being practiced in-house or through consultants, we observe that firms have identified the skill sets they require from their people. Typically known in the industry as data scientists, they are expected to be good at multiple aspects:
1) Talking to the client and understanding the business problem
2) Gathering client requirements and understanding the data available
3) Accessing and shaping the data and making it ready for analysis
4) Knowing and applying statistical techniques and models
5) Communicating to the client the insights gained
Most โdata scientistsโ in the industry are not able to live to such expectations, leaving firms confused about the way to proceed. If we take a step back and analyze a bit, the reasons are clear. The expected skill sets cover a wide number of domains, tools and techniques. Some of these skills are honed in the marketing and sales functions, others in an engineering setup, and some may require a natural quantitative aptitude and ease with math and logic subjects. There are some gifted โunicornsโ who do possess these skills or can learn them quickly, but they are few and far between, and coming across them is often a matter of luck.
One way some firms have responded to this is to search more aggressively for the right candidates, making attractive offers, hoping they will get lucky. On the other hand, some firms have decided to take a more systematic approach. For e.g. at Quantiphi, we deconstructed the expected Data Scientist role into three sub roles: Decision Scientist, Business Analyst and Data Engineer, and assigned them responsibilities in the following way:
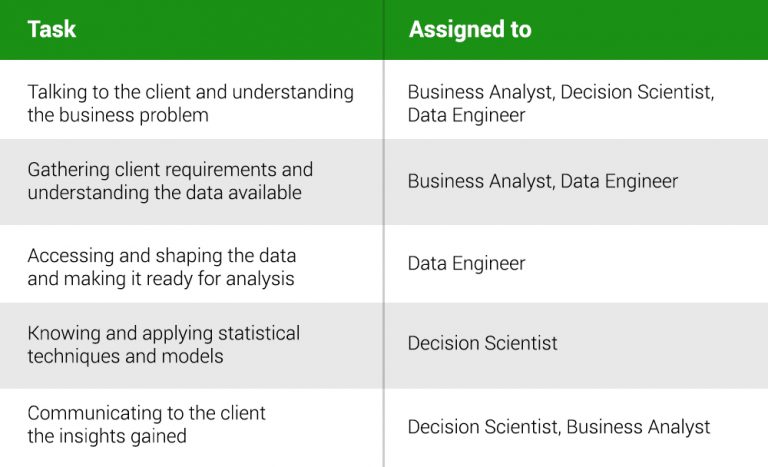
We call such a team of people a โsynthetic unicornโ. We have found it much more productive and faster to find the right people and if necessary train them for these roles, than to chase unicorns. Not only is this a more scalable model, it also allows each member of the team to specialise in their discipline and build depth, which enables us to tackle complex problems for our clients. For eg. lately, Big Data platforms have gained significant traction, and data storing and processing technologies are evolving rapidly. Delivering state-of-the-art solutions to clients requires time investment to keep abreast of the developments.
As the industry matures and realistic expectations set in, we expect a lot more analytics departments and firms to adopt a similar model, and thus industrialise their insight generation process.