Public transport infrastructure acts as the engine that fuels mobility, urbanization, and long-term growth across economies. The expansion and continuous maintenance of public transport are closely linked with the upliftment of living standards and increased industrial growth around the world.
A joint research study found that expanding the road network in rural parts of China resulted in a four-fold higher relative growth in GDP for the country. The same study also highlighted how investments made in highway infrastructure resulted in 60% greater industrial output in Sri Lanka. In the US, public transportation is an $80 billion industry that contributes to the creation of 50,000 new jobs for every $1 billion invested.
While investing in public transport is lucrative, there are several fundamental challenges along the way. Building more roads or setting up new subway lines invite cost, public safety, and environmental concerns. Not to forget that transport emissions have more than doubled since 1970 as per Intergovernmental Portal for Climate Change.
The question that begs an answer is how can public transport be modernized for safety, efficiency, and eco-friendliness as new infrastructure is developed at scale?
One contender is the application of Artificial Intelligence for solving transport sector problems. AI systems can interpret data and act autonomously which makes them capable of decluttering traffic congestion in real-time or navigating self-driving cars in a busy street. At Quantiphi, we are actively working with transportation authorities to develop AI solutions that help them overcome operational and managerial challenges.
Let’s explore the ways in which AI solutions reduce the impediments to transport infrastructure modernization.
Challenges in the Transport Sector
In the US, highway congestion costs about $300 billion in wasted time annually. This is nearly 150% of the state and local government spending on highways.
(Source: Transportation Research Board, US Census Bureau)
The Transportation Research Board identifies the following as the key issues surrounding transportation:
Transformative Technology
Transformative technologies such as electric vehicles, automated delivery systems, etc, are limited in scale as of now. There is an observable lag in transport technology keeping up with the pace of urbanization, which is giving rise to more problems than it is solving.
Sustainability
Passenger vehicles can release about 4.6 metric tons of carbon dioxide into the air in an entire year. Identifying Carbon Dioxide and greenhouse emission hotspots has been an upstream battle for authorities around the world. With Climate Change on the rise, it's high time we take action to counter it.
Infrastructure Management
The upkeep of current transport infrastructure and managing the development of new roads, railways, and airports are consistent budgetary challenges for public authorities.
Public Safety
Rampant urbanization brings safety concerns in terms of road accidents and pollution-caused impairments.
While the above points touch upon the transportation sector as a whole, road transport is one dominion that needs the most attention. It’s the most widely used public infrastructure that affects more lives than any other. The focus areas for road transport include the following;
- Poor traffic management and insufficient accident prevention.
- Inferior conventional forecasting methods and difficulty in correlating ridership data from multiple sources.
- Lack of data-driven decision making and problems in analyzing live-stream data.
- Growing need for sustainable logistics operations.
- Lack of hardware capabilities in differentiating different asset types from the environment.
Embracing AI in Transportation
AI has the potential to transform the entire transportation space - from autonomous cars in road transportation to fleet management in aviation. AI’s vast range of applications is turning heads across the globe and reeling in significant investment in both the public and private sectors.
The global market for AI in transportation is expected to reach $3.5 billion by 2023. This is further validated by the fact that the US Federal government is investing 50% of the $550 billion Infrastructure Investment and Jobs Act in transportation. The main objectives of the bill are to;
- Modernize the existing transportation infrastructure including roads, bridges, railways, airports, and ports.
- Focus on the safety of all users.
- Make US infrastructure resilient against the impacts of climate change, cyber-attacks, and extreme weather events.
The European Union launched its own action plan on Artificial Intelligence which underlines certain steps to facilitate the growth of AI in transportation. The key tenets of the strategy include;
- Boost public and private investment in AI (expectedly by EUR 20 billion).
- Prepare for socio-economic changes brought about by AI.
- Update the safety and liability frameworks for the incorporation of AI technologies.
Stockholm introduced AI-powered driverless buses in 2018, which is a significant leap forward for public transport. The buses self-navigate using data inputs provided by GPS, camera, and onboard sensors that are processed by an AI system to provide real-time autonomous driving capabilities.
How Can Public Transportation be Modernized using AI?
Modernizing public transportation means equipping it with next-gen technologies that can improve operational efficiency and overcome present challenges while keeping sustainability in mind. We have recognized the following core areas for the application of AI in Public Transportation:
- Predictive Maintenance of Fleet, Roads, and Equipment: With the help of predictive analytics models, cloud-based technologies, and the Internet of Things (IoT) devices, authorities can now adopt a proactive approach to maintenance and management of infrastructure, fleet, and equipment, thus reducing cost, increasing safety and ensuring system reliability.
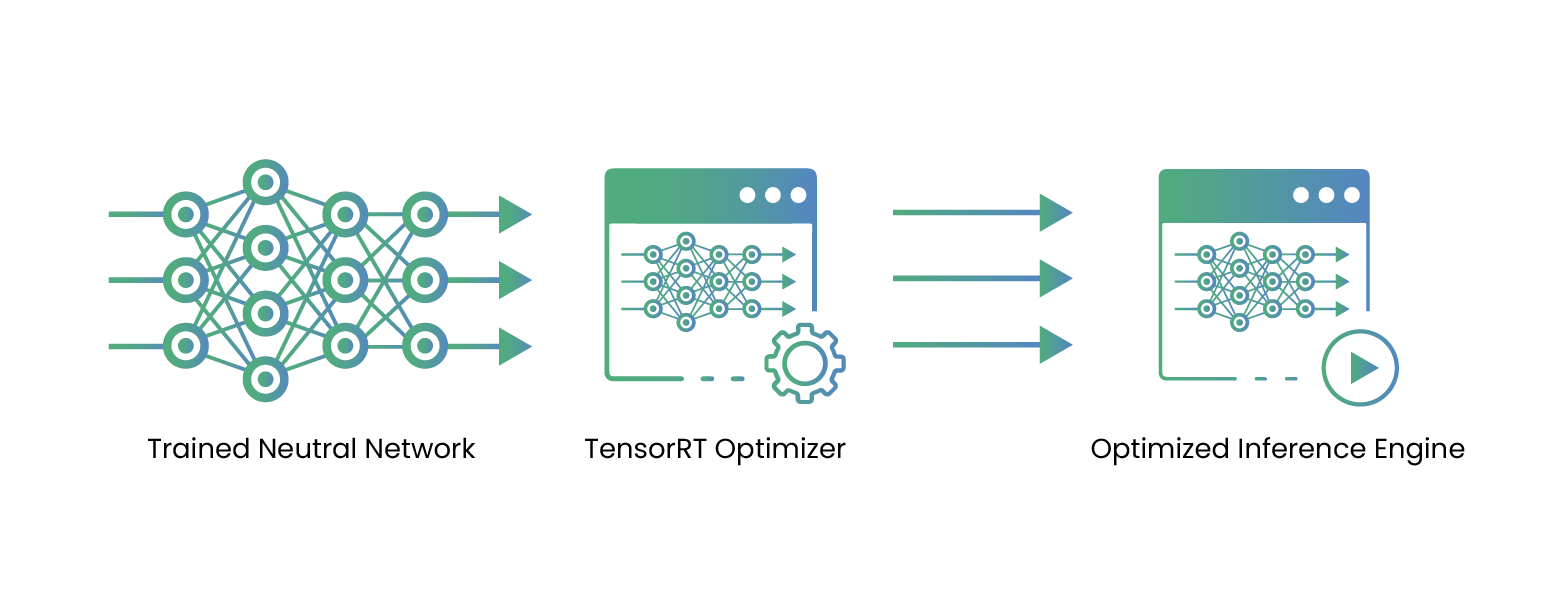
- Hot Spot and Crash Prediction: A predictive model which analyzes historical data, identifies accident hotspots and assigns a severity index to every hotspot and crash, thus enabling the authorities to take necessary steps well in advance to prevent fatal crashes.
- Asset Monitoring System: A solution for agencies to improve situational awareness around roads, bridges, tracks, and waterways to avoid accidents and delays. The solution detects, identifies, and notifies authorities about different types of assets around the respective environments, thus helping in better decision-making.
- License Plate Tracking System: Using a Computer Vision solution to analyze the camera feed and extract Region of Interest (ROI) pertaining to the license plate. This can be used to classify vehicles as private or public and visualize vehicular patterns on a customized dashboard.
- Smart Parking System: A solution that provides real-time visibility to citizens on parking availability within the lot along with the location of the potential parking spaces using Computer Vision.
- Ridership Prediction and forecasting for Public Transit: A solution leveraging predictive analysis and forecasting models to analyze transit behaviors of various citizen demographics in a city, helping authorities derive valuable insights and, in turn, solve challenges such as rush hour congestion and reduce costs.
- Contractor Evaluation: A solution that helps authorities evaluate and assess contractors and vendors on multiple parameters using Data Modeling and Auto ML algorithms. Authorities can rely on the solution to find the best-suited contractor or vendor for their needs with minimal human intervention and impeccable accuracy.
Quantiphi’s AI Innovation in Public Transportation
Quantiphi has participated in several initiatives for digitizing and automating legacy transport systems using AI. The following is an example of how we integrated AI automation into a rail navigation system of a Railway Corporation using computer vision.
Problem Background
A Railway Corporation managing a rail track infrastructure spread across 20,000 miles was relying on legacy processes for field operations. The locomotive operators needed to keep an eye on indicator lights, modifier plates, and flags located alongside the track to make pre-defined operational decisions such as stopping the train or changing tracks. They would also look out for any pedestrians or crew members who might inadvertently find themselves in the path of a moving train.
The manual nature of the job made it prone to error and labor-intensive. The client wanted to automate the track signal detection process and reduce any fatalities caused by the misjudgment.
Solution Overview
Quantiphi came up with a computer vision solution that could detect flags, signals, trespassers, and various entities on the tracks, thus helping crew members to make faster and better decisions.
The deployed solution consists of a camera affixed in front of the train that is capable of processing the real-time video streams using an AI-enabled component. It can automatically classify objects appearing in the video and enable locomotive operators to take actions with greater accuracy.
The solution also offered heatmap imagery of trespassers on the track, which enabled the operators to take proactive preventive actions to avoid accidents in time. While building this solution, Quantiphi came across some unique implementation challenges such as,
- Difficulty in analyzing real-time streaming video data and making decisions using computer vision.
- Lack of data for varied daylight and weather conditions .
- Lack of data for distinguishing between solid lights and flashing lights.
- Difficulty in associating signals to their relevant track.
Technical Overview
The engineers at Quantiphi put together a stack consisting of NVIDIA Jetson AGX Xavier - a compute platform used for AI-powered neural network training and the TensorRT framework for deep machine learning inference.
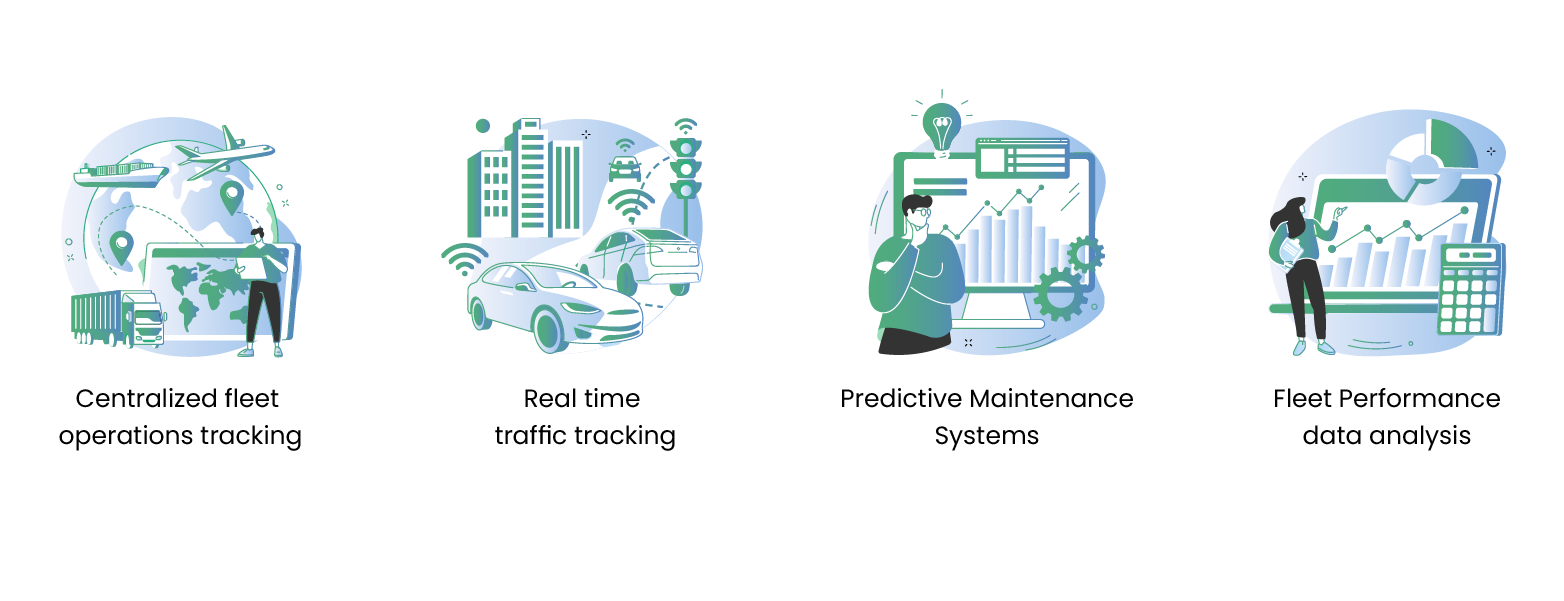
The real-time video was uploaded to NVIDIAs computer devices where it was inferred for object detection based on pre-trained ML models.
Solution Outcomes
The solution achieved an object detection and classification accuracy of 95% and signal detection accuracy of approximately 95%. It also reduced the time-to-action for operational decisions as it could detect objects much faster than the human eye. It further gave detailed insights into the operational process with the help of trespasser detection and flag detection.
On a whole, the solution transformed a predominantly labor-oriented process into an autonomous one. It met the operational challenges with near-perfect accuracy and performed as per expectations in erratic lighting conditions. The railway locomotives are now easier to operate for the client with a decremental error rate while the track is increasingly safer for the crew members to work upon.
Conclusion
With a gradual penetration of AI-powered technologies in transportation, it’s now possible to design cleaner, safer, and more efficient solutions. From scanning traffic patterns for decluttering highways and reducing road accidents to minimizing emissions at large, AI is offering new ways to transform public transport as we know it.
Quantiphi is committed to growing and evolving our services and products for transport innovation. We are constantly looking out for problem statements and areas of improvement where our deep technical expertise can be employed for optimizing public transport.
We invite your interest in discussing solution designs that require complex problem-solving and AI deployment at scale. Let’s change the face of public transportation together!
Want to talk to our experts on how to create a citizen centric experience using AI? Drop in your details here.