This article was originally published on SportsPro Media.
Quantiphi has been working with the DFL to manage six decades of archive video, but the AI-powered solution offers possibilities across all types of sports content.
The German Football League’s (DFL) Media Hub currently manages the video data equivalent of 2.75 billion smartphone photos. With fifteen years of careful archiving, the DFL has curated and recorded some of the most momentous moments in soccer – last minute wins, stunning goals and individual pieces of brilliance.
The DFL, which oversees the elite professional tiers of German club soccer, has created video data library with more than 175,000 hours of content. Managed by its Sportcast subsidiary, the DFL Media Hub, which includes the official German soccer archive, consists of content from the Bundesliga, 2. Bundesliga, Liga 3, Women's Bundesliga, and German international matches. It is continuously growing, with more than 12,000 hours of video added every year.
This archive was aggregated from formerly siloed storage sites, digitized at the highest levels of quality, categorized and preserved for long-term storage. This includes full-length matches, highlights and live broadcast recordings. However, for the DFL, aggregating match footage is only half of the story. Making this content searchable and accessible to Bundesliga clubs, its global media partners, sponsors and agencies needs an artificial intelligence (AI) intervention.
For the past few years, the DFL has augmented its existing archiving efforts with video intelligence with Quantiphi, a computer vision and AI-first digital transformation leader. Here, AI generates highly detailed, video content-related metadata to increase searchability in the DFL Media Hub.
But why is searchability an AI challenge?
The DFL leverages Sportcast’s official match data and live-logging to provide descriptive information about every fixture under its watch. Still, the content needs of the DFL Media Hub users are disparate and tagging this volume of content manually is not possible.
If the highlights of a famous player in the 1980s were to be created, searching worthy editorial shots would mean watching hours of unseen footage. AI solves this challenge by generating granular metadata within the video. This metadata helps AI auto-identify and capture essential information around players' faces, emotions, desired camera angles, and specific events such as red cards or goals. The Bundesliga recently revealed that this AI-generated metadata helped transform more than 11 petabytes of video data into a treasure trove of highly-usable content.
“Cataloguing and tagging far more than 100,000 hours of historical, as well as brand new video content, on a sequence level is simply not feasible exclusively with human resources,” said Christoph Forster, head of DFL Media Hub at Sportcast. “This is why we have always been searching for ‘technical assistants’ to do this – and we learned that machine-learning and AI-driven technologies would probably be the best solutions for this purpose.”
Metadata eliminates the manual effort in searching for the right content. Instead, over time, AI learns from the selected choices and improves itself via active learning.
How does AI search the desired content in sports archives?
“Football or any sports content is a unique field for AI given the amount of information a single match can generate and how multiple sports can differ. But, to make sports archives searchable according to editorial or custom requirements – relevant metadata is a must-have,” said Niraj Nishad, Quantiphi solution architect.
There are three core aspects in training AI models to assist with archival needs at this scale.
1. Relevance: The model must automatically identify and capture relevant metadata for archive users and editors. This is done by developing a metadata taxonomy unique to the sport. This can include:
- Face and emotion recognition
- Camera angles
- Sponsorship logos
- Custom match events (goals, red cards, etc.)
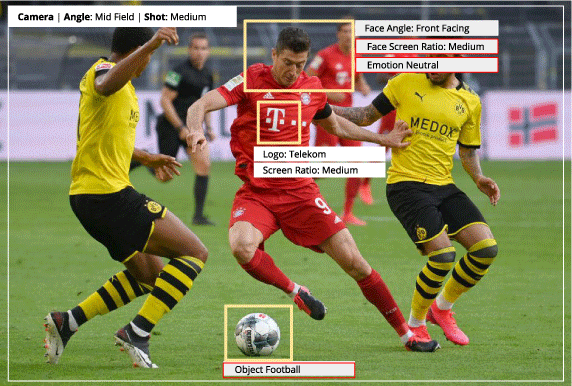
2. Metadata quality: Not all metadata which is relevant can be helpful. The metadata must pass pre-set quality criteria. For instance, the captured data should show players' faces clearly and devoid of any motion blur to be beneficial. The player who is not occupying a central position needs to be discarded despite the accurate identification of the player or emotion.
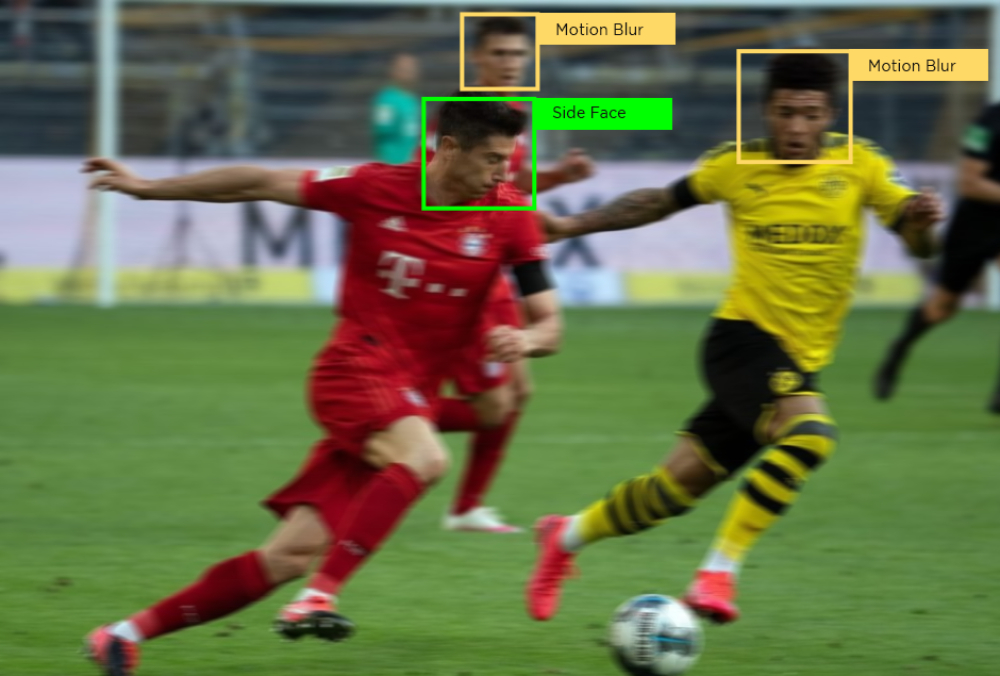
3. Scalability: This means the AI model must be scalable to manage decades of past content and work perfectly while adding new players to the team's roster. The generated tags must be integrated with existing media asset management systems and compatible with existing media workflows.
Above all, the developed solution must comply with the data privacy and security norms governing the league and its players.
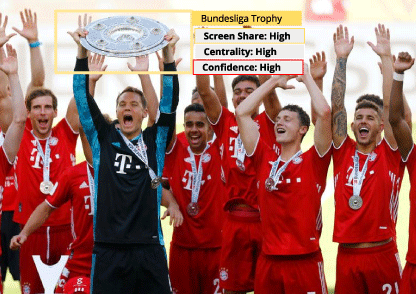
Applications of archiving sports content
Delivering desired content to diverse global users
An enriched archive opens up multiple possibilities to monetize and license sports content globally. The more comprehensive and detailed metadata entries are, the easier and faster users can find suitable video content.
Quick and custom highlights
During matches, multiple live feeds capture the same game from different angles. Editorial teams need to manually analyse and extract relevant content from this bulk data. Now, content creators and archive users can curate sports content with the utmost ease. For instance, quick highlights can be created using AI-enabled editing workflows which have been learned from editorial actions. For custom highlights, these can be targeted to different user segments depending on the fanbase, interest and other business needs based on that same metadata.
Driving storytelling in sports
Storytelling in sports is now mainstream. Multiple leagues, broadcasters are fusing match content and behind-the-scenes footage with an engaging narrative to engage fans globally. AI can also help identify footage with the right emotions, custom events and desired camera angles for sports documentaries.
How can AI be customized for other sports?
Every sport is different with its own editorial requirements, but the core pillars of relevance, quality and scalability broadly hold true. For instance, motorsport like Formula One would need a higher focus on metadata quality due to fast-moving vehicles. Content from the National Football League (NFL) would have numerous players in single frames which make the requirement to tag relevant players imperative.
What rings true universally, is that the model must adhere to the data privacy norms governing the players and the league.
Read the case study to learn how Quantiphi enabled DFL with AI powered sports archiving.